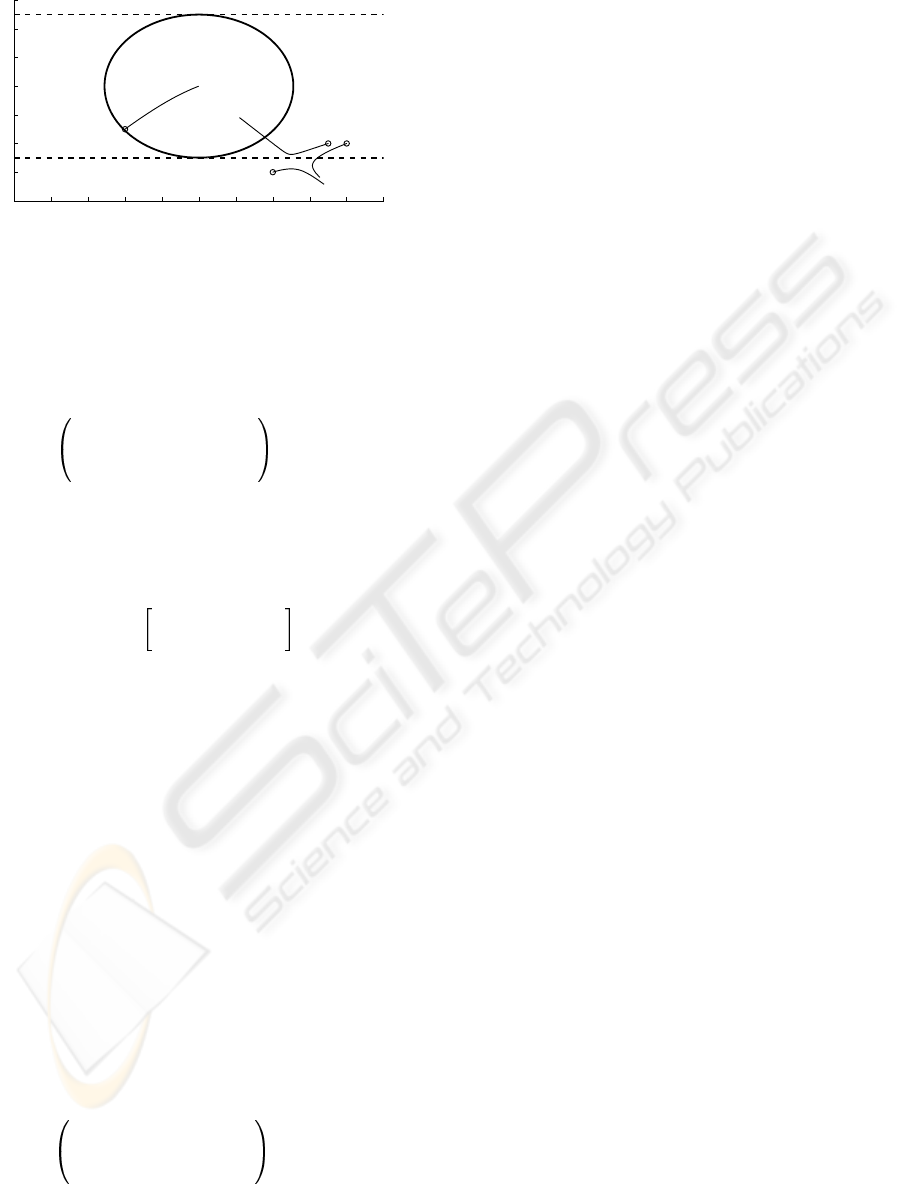
−1 −0.8 −0.6 −0.4 −0.2 0 0.2 0.4 0.6 0.8 1
−0.8
−0.6
−0.4
−0.2
0
0.2
0.4
0.6
x
1
x
2
Θ
Ω
Figure 2: The Basin of attraction of x = 0 provable by
quadratic stability (Example 1).
for any ρ < 0.5. for instance, ρ
n
= 0.499 results in
the following LMI conditions:
A
∗
1
T
P + P A
∗
1
< 0
A
∗
2
T
P + P A
∗
2
< 0
P
0
1/ρ
n
0 1/ρ
n
1
> 0
P > 0
λI > P
which are feasible for the above value of ρ
n
and, the
matrix P obtained for the minimum λ defines an ellip-
soidal basin of atraction Θ = {x\x
T
P x ≤ 1} with
P =
3.8274 0
0 4.016
Figure 2 depicts the obtained basin of attraction,
and some examples of trajectories from a set of start-
ing points. The maximum circle found by the LMI
toolbox has a radius of 0.499.
Trajectories with starting points inside the ellipsoid
Θ are guaranteed to converge to the origin. Note that
the ellipsoid obtained by the LMIs is not a circle.
Points outside the ellipsoid may lead to either con-
vergent or non-convergent trajectories.
Example 2. Let us considerer the same fuzzy
model as in Example 1. Then applying the theorem
2,
minimize λ subject to
(A
T
1
P + P A
1
) − (1 − µ
M
1
)N
M
j
+ (1 − µ
m
1
)N
m
1
+µ
M
2
N
M
2
− µ
m
2
N
m
2
< 0 ∀i 6= j
(A
T
2
P + P A
2
) − (1 − µ
M
2
)N
M
j
+ (1 − µ
m
2
)N
m
2
+µ
M
1
N
M
1
− µ
m
1
N
m
1
< 0
P
0
1/ρ
n
0 1/ρ
n
1
> 0
P , N
M
1
, N
m
1
, N
M
2
, N
m
2
> 0
λI > P
Conveniently, we take the same region shape Ω that
in Example 1, the limits µ
m
i
and µ
M
j
are the mini-
mum and maximum value of µ
i
in the region Ω. The
maximum ρ obtained is 0.26, i.e. 0.24 units less than
the obtained in the previous example. From this ex-
ample, the conditions discussed in Section 4.2 seem
more conservative than those in Section 4.1.
6 CONCLUSIONS
This paper shows how local stability results (the
largest sphere around x = 0 for which a quadratic
Lyapunov function can be proven via LMI) may
be obtained in fuzzy systems via the knowledge of
the membership functions, even when no feasible
quadratic Lyapunov function can be found to prove
global stability. The found sphere is part of a larger
ellipsoidal guaranteed basin of attraction.
In this way, if the linearised system around the
equilibrium is stable, a precise characterisation of the
local stability region stated in Lyapunov 1st theorem
is achieved.
The approach based on relaxed LMI conditions
from membership bounds yields more conservative
results but it is simpler, without the need of changing
the Takagi-Sugeno consequents.
REFERENCES
Boyd, S., ElGhaoui, L., Feron, E., and Balakrishnan, V.
(1994). Linear matrix inequalities in system and con-
trol theory. Ed. SIAM, Philadelphia, USA.
Johansson, M. (1999). Piecewise quadratic stability of
fuzzy systems. IEEE Trans. Fuzzy Syst., 7:713–722.
Khalil, H. (1996). Nonlinear Systems (2nd. ed.). Ed.
Prentice-Hall, Upper Saddle River, New Jersey, USA.
Luenberger, D. (2003). Linear and Nonlinear Program-
ming. Springer, 2nd edition.
Oliveira, M. d., Bernussou, J., and Geromel, J. (1999).
Anew discrete-time robust stability condition. System
& Control Letters, 37:261–265.
Takagi, T. and Sugeno, M. (1985). Fuzzy identification
of systems and its applications to modelling and con-
trol. IEEE Trans. on Systems, Man and Cybernetics,
15(1):116–132.
Tanaka, K. and Wang, H. O. (2001). Fuzzy control systems
design and analysis. Ed. John Wiley & Sons, New
York, USA.
Wang, H. O., Tanaka, K., and Griffin, M. F. (1996). An
approach to fuzzy control of nonlinear systems: Sta-
bility and design issues. IEEE Transactions on Fuzzy
Systems, 4:14–23.
DETERMINING ELLIPSOIDAL BASINS OF ATTRACTION OF FUZZY SYSTEMS
91