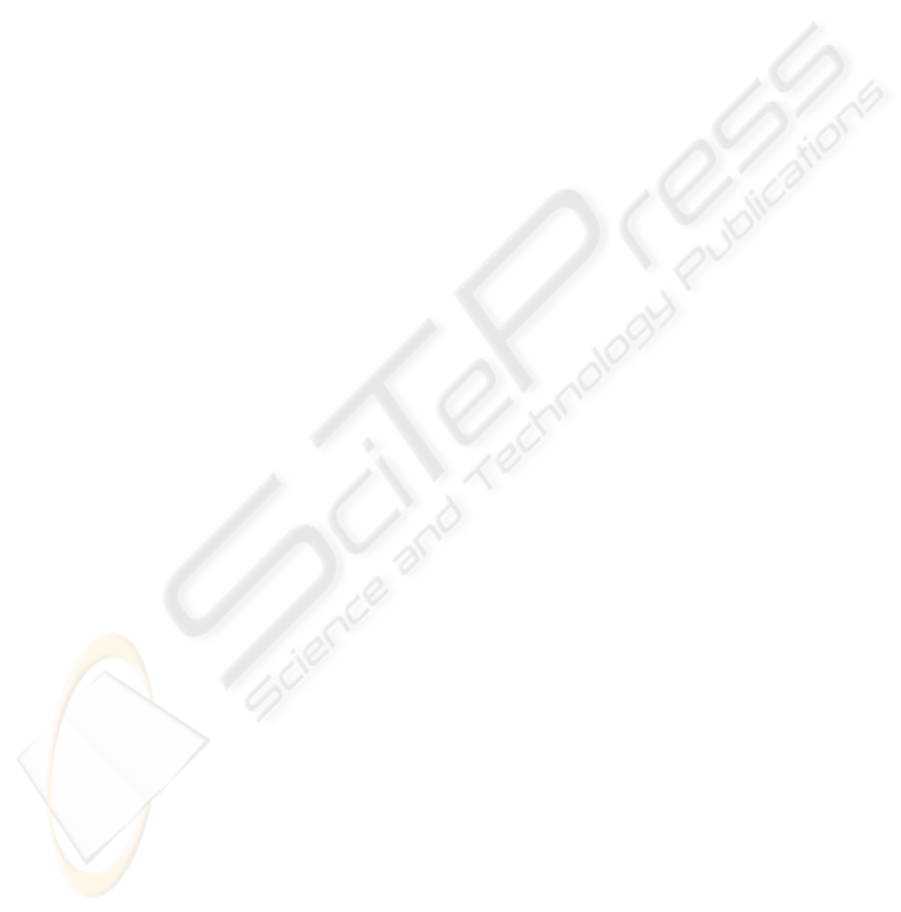
The energy distribution based on the complex
atomic dictionary in Figure 4 is better localized in
time and frequency than the other. Also its resolu-
tion is much higher, and its high-energy deterministic
components can be much better separated from low-
energy stochastic components.
As we have announced, we are not only interested
in separating deterministic and stochastic components
in control signals, but also in separating their con-
stituents, i.e. periodic, subharmonic, chaotic and tran-
sient for deterministic nonlinear time-varying com-
ponents versus non-stationary and stationary for sto-
chastic components. The non-stationary and deter-
ministic chaotic vibrations present in the servo error
signal have narrow high-frequency support and are
difficult to be detected using Wigner distribution. By
applying the adaptive decomposition algorithm de-
scribed in Section 2.2.2, we are also able to identify
such narrow-band frequency characteristics, as can be
seen from the ‘banana’ shape of the dark patterns in
Figure 4, which are much more expressive than the
black patterns in Figure 2.
In Figure 2 the local cross-terms around t =
{0, 0.1, 0.2, 0.3} are not eliminated, while in Figure
4 they are not present anymore.
The energy plot in Figure 4 does not contain cross-
terms and any numerically undesired effects, as in
Figure 2.
Additionally, our multi-dictionary approach leads
to a clearer identification of time-varying non-linear
and stochastic servo error components and therefore,
to an improved adaptive ILC design.
We further observe that an overall smaller band-
width than in Figure 2 is obtained (not higher than
about 400 [Hz] in the beginning of the acceleration
profile and 100 [Hz] around other jerk moments).
Therefore, the ILC needs to learn only around these
jerk moments up to smaller frequencies than those
found when Wigner distribution was applied for the
computation of the bandwidth profile.
This means that the stochastic effects will not be
amplified unnecessarily (high bandwidth means good
tracking performance and noise amplification) while
all existent deterministic effects will be learned. Also,
unlike in Figure 2, we do not obtain any increased
bandwidth because of cross terms: the bandwidth of
the Q-filter needs to be increased just around the jerk
moments while, in between, a small bandwidth can be
maintained.
Also, as seen in Figure 4, the bandwidth profile has
a very good smooth approximation. Therefore, fast
switching between the cut-off frequency of Q-filters
that corresponds to different time instances is avoided
and the stability of the switched ILC system is not an
issue anymore.
REFERENCES
Buckheit, J., Chen, S., Donoho, D., Johnstone, I., and Scar-
gle, J. (1995). Wavelab. In Tech. Rep., Stanford Uni-
versity.
Cappellini, V. and Constantinides, A. (1984). Interference
terms in the Wigner distribution. Digital Signal Proc.
Casalino, G. and Bartolini, G. (1984). A learning proce-
dure for the control of movements of robotic manipu-
lators. In Proc. 4th IASTED Symp. Robotics Automa-
tion, pages 108–111.
Chandroth, G. O. (1999). Diagnostic classifier ensembles:
Enforcing diversity for reliability in the combination.
In University of Sheffield, UK.
Chang, C.-K., Longman, R. W., and Phan, M. Q. (1992).
Techniques for improving transients in learning con-
trol systems. Adv. Astronautic. Sci, 76:20352052.
Chen, Y. and Moore, K. (2001). Frequency domain adap-
tive learning feedforward control. In Proc. of the 2001
IEEE Int. Symp. Comp. Intell. Robotics and Automa-
tion, pages 396–401.
Cohen, L. (1989). Time-frequency distributions – a review.
Proc. IEEE, 77(7).
Daubechies, I. (1991). Ten lectures on wavelets. Series in
Applied mathematics, SIAM.
Goh, C. J. (1994). A frequency domain analysis of learn-
ing control. J. Dynamic Syst., Measurement, Contr.,
116:781–786.
Mallat, S. and Zhang, Z. (1993). Matching pursuit with
time-frequency dictionaries. IEEE Trans. on Signal
Processing, 41(12):3397–3415.
Margrave, G. F. (1998). Theory of nonstationary linear fil-
tering in the fourier domain with applications to time-
variant filtering. Geophysics, 63(1):244 – 259.
Mecklenbr
¨
auker, W., Hlawatsch, F., and Janssen, A. (1997).
The wigner distribution, theory and applications in
signal processing. Elsevier.
Moore, K. L. (1993). Iterative learning control for de-
terministic systems. Adv. in Ind. Control. Springer-
Verlag.
Norrl
¨
of, M. (2002). Iteration varying filters in iterative
learning control. In Proc. 4th Asian Control Conf.,
page 21242129.
Rioul, O. and Vetterli, M. (1991). Wavelets and sig-
nal processing. IEEE Signal Processing Magazine,
8(4):14–38.
Rotariu, I., Dijkstra, B., and Steinbuch, M. (2004). Com-
parison of standard and lifted ilc on a motion system.
In 3rd IFAC Symp. Mechatronic Systems, Sydney, Aus-
tralia, pages 211–6.
Rotariu, I., Ellenbroek, R., and Steinbuch, M. (2003a).
Time-frequency analysis of a motion system with
learning control. In Proc. ACC Conf., pages 3650 –
3654. American Control Conf.
ICINCO 2006 - SIGNAL PROCESSING, SYSTEMS MODELING AND CONTROL
30