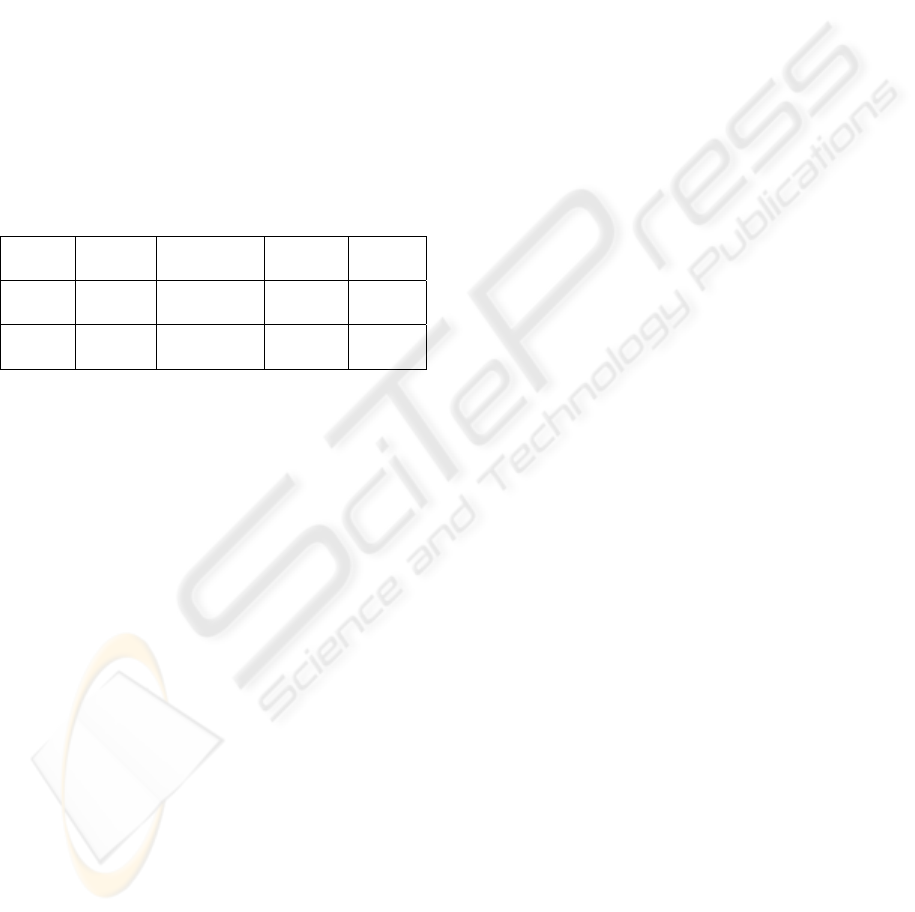
falling forward,
z The body crooked and falling backward, and
z The body crooked and falling right side or left
side.
These activities are simulated by the MatLab7
neural network tool in the learning phase. Through
20,000 times iterative learning, the back propagation
network is converged. In the recall phase, we
evaluate 90 activities which are the same activity
types as in the learning phase. In the evaluation, two
types of failure are adapted in our testing
(Yamaguchi, 1998). The first type of failure is that
the device indicating a fall event, but the real
situation is nothing. The next type of failure is the
fall accident is real in happened, but not detected by
the detector. The evaluation results are shown in
Table 2.
Table 2: The evaluation result for detecting normal and fall
activities.
Activitie
s
Num. of
evaluation
Success
detection
Error
detection
Correct
rate
Normal
activitie
60
No alarmed
57
Alarmed
3
95%
Fall
activitie
30
Alarmed
28
No Alarmed
2
93.3%
The similar experiment is repeated several
times. We have found that detection errors in testing
normal activities are almost distributed in “sit down”
activity. The errors in testing fall activities are
distributed in the “body crooked and falling right
side”. Next, the factors of subject’s agility, the
distance from detector to ground, and the numbers of
learning cycle in back-propagation neural network
are also infected the correct rate in the experiment.
5 CONCLUSIONS
An intelligent fall detector that based on artificial
neural network technology is introduced in this
paper. Comparing with other approaches, the correct
rate of recognition of fall activity by neural network
learning approach is better than the predefined
threshold value approach. In the future research, we
will explore the others learning approaches, such as
Hidden Markov Model classifier, to compare with
the neural network approach.
ACKNOWLEDGEMENTS
This project was funded by the National Science
Council, Taiwan (grant number NSC94-2218-E-
320- 003).
REFERENCES
Blake, A.J., 1992. Fall in the elderly. Br J Hosp Med, 47,
268-72.
Degen, T. and Jaeckel, H., 2003. SPEEDY: a fall detector
in a wrist watch. In Proceedings of 7th IEEE
International Symposium on Wearable Computers.
21-23 Oct.. pp.184 – 187
Doughty, K., 2000. Fall prevention and management
strategies based on intelligent detection, monitoring
and assessment. Presented at New Technologies in
Medicine for the Elderly, Charing Cross Hospital, 30
th
,
Nov.
Haga, H., Shibata, H., Mitsuzaki, T., and Hatano, S., 1986.
Falls in the institutionalized elderly in Japan. Arch
Gerentol. Geriatr. Vol.5, pp.1-9
Mathie, M.J., Basilakis, J., and Celler, B.G., 2001. A
system for monitoring posture and physical actitity
using accelerometers. In Proceedings of the 23rd
Annual EMBS International Conference, 25-28 Oct.
Turkey. Pp.3654-3657
Noury, N., 2002. A smart sensor for the remote follow up
of activity and fall detection of the elderly. 2
nd
Annual
International IEEE-EMBS Special Topic Conference
on Microtechnologies in Medicine & Biology. May
Madison, Wisconsin. USA. pp.314-317
Tinetti, M.E., 1994. Prevention of falls and fall injuries in
elderly persons: a research agenda. Preventive
Medicine. Vol 23. pp.756-762
Williams, G., 1998. Doughty, K., Cameron,K. and
Bradley, D.A.. A smart fall and activity monitor for
telecare applications. In International Conference of
IEEE-EMBS, HongKong, pp.1151-1154
Wu, G., 2000. Distinguishing fall activities from normal
activities by velocity characteristics. Journal of
Biomechanics, Vol. 33. pp.1497-1500
Yamaguchi, A., 1998. Monitoring behavior in home using
positioning sensors. In International Conference of
IEEE-EMBS, HongKong, pp.1977-1979
ICINCO 2006 - INTELLIGENT CONTROL SYSTEMS AND OPTIMIZATION
206