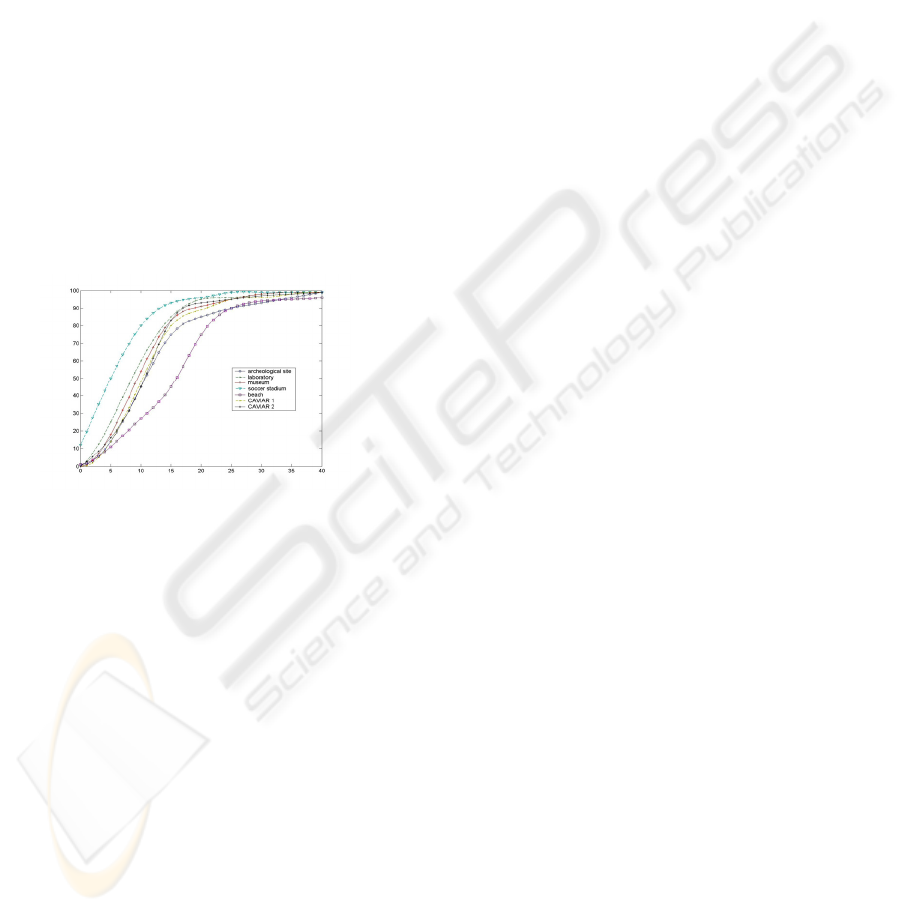
without assuming knowledge of the actual
foreground distribution. This analysis is performed
by shifting or perturbing the entire background
distributions by values with fixed magnitude Δ, and
computing an average detection rate as a function of
contrast Δ. More details about this procedure can be
found in relative paper. The PDR analysis has been
applied to all the experimental contexts presented
above. The test set is given by 500 points for each
frame, 200 frames for each sequence in each
context. So, for each Δ, 200*500 perturbations and
detection tests were performed. In figure 2 we have
plotted the resulting PDR graphs. The worst results
have been obtained in the beach, where the critical
conditions due to the presence of moving
background objects decrease the performance. In
this case, the pixel intensity variations, due to the
movement of the vegetation, are amplified by the
perturbation introduced, causing a decrease of the
global detection ability. On the other hand, the
results obtained in the remaining contexts are very
interesting, with a fast growth of the curve towards
best performances, as already observed in table 2.
Figure 2: the PDR analysis on the test sequences. It can
be note that the best performance have been obtained in
the soccer stadium and in the indoor contexts, while the
worst results have been reported in the beach, probably
due to the presence of moving vegetations.
5 CONCLUSIONS
In this paper an approach for the unsupervised
building of a background model has been proposed.
The proposed algorithm is able to model a scene
even in presence of foreground objects and moving
background ones; no a-priori assumptions about the
presence of these moving objects, and changing in
light conditions are needed. It combines the energy
information of each pixel with a temporal analysis of
the scene, by means of sliding windows, to detect
static background points. Energy information is used
again over the whole observation period to
distinguish moving background objects from
foreground ones, and the background is modeled by
means of a multilayer statistical distribution.
The algorithm has been tested in many different
contexts, such as a soccer stadium, a parking area,
an archeological site and two test sequences
extracted from the CAVIAR 2005 dataset. The
experimental results prove that the proposed
algorithm try to correctly model the background in
every kind of condition, in presence of both moving
background and foreground objects. The quantitative
evaluation presented confirm that the proposed
algorithm gives reliable results.
REFERENCES
Chalidabhongse, T.H., Kim, K., Harwood, D., Davis, L.S.,
2003. A Perturbation Method for Evaluating
Background Subtraction Algorithms, Proc. VS-PETS.
Doretto, G., Chiuso, A., Wu, Y.N., Soatto, S., 2003.
Dynamic textures. In IJCV, 51 (2), pp 91-109
Elgammal, A., Harwood, D., Davis, L.S., 2000. Non-
parametric model for background subtraction. In
ECCV, Vol. 2, pp. 751-767
Haritaoglu, I. ,Harwood, D., Davis, L.S., 1998. Ghost: A
human body part labeling system using silhouettes. In
Fourteenth ICPR, Brisbane.
Jaraba, E.H., Urunuela,C., Senar,J., 2003. Detected motion
classification with a double-background and a
Neighborhood-based difference, PRL, (24),pp.2079-
82.
Kanade, T., Collins, T., Lipton, A., 1998. Advances in
Cooperative Multi-Sensor Video Surveillance. Darpa
Image Und. Work., Morgan Kaufmann,pp.3-24.
Kim, K, Chalidabhongse, T.H., Harwood, D., Davis, L.,
2004. Background modeling and subtraction by
codebook construction. Proc. ICIP, Vol. 5, pp 3061–
64
Koller, D., Weber, J., Malik, J., 1994. Robust multiple car
tracking with occlusion reasoning. In ECCV pp 189-96
Lipton, A.J., Haering, N., 2002. ComMode: an algorithm
for video background modeling and object
segmentation. Proc. of ICARCV, pp 1603-08, vol.3
Monnet, A., Mittal, A., Paragios, N., Ramesh, V., 2003.
Background modelling and subtraction of dynamic
scenes. In ICCV, pp. 1305-12
Stauffer, C., Grimson, W., 1999. Adaptive background
mixture models for real-time tracking. In CVPR, pp
246-252
Toyama, K., Krumm, J., Brumitt, B., Meyers, B., 1999.
Wallflower: Principles and practice of background
maintenance. In ICCV, pp. 255-261
Wren, C.R. Azarbayejani, A., Darrell, T., Pentland, A.P.,
1997. Pfinder: real-time tracking of human body.
IEEE Trans. PAMI, 19(7), pp. 780 – 785.
Zhong, J., Sclaroff, S., 2003. Segmenting foreground
objects from a dynamic, textured background via a
robust kalman filter. In ICCV, pp.44-50
AN ENERGY-BASED BACKGROUND MODELLING ALGORITHM FOR MOTION DETECTION
383