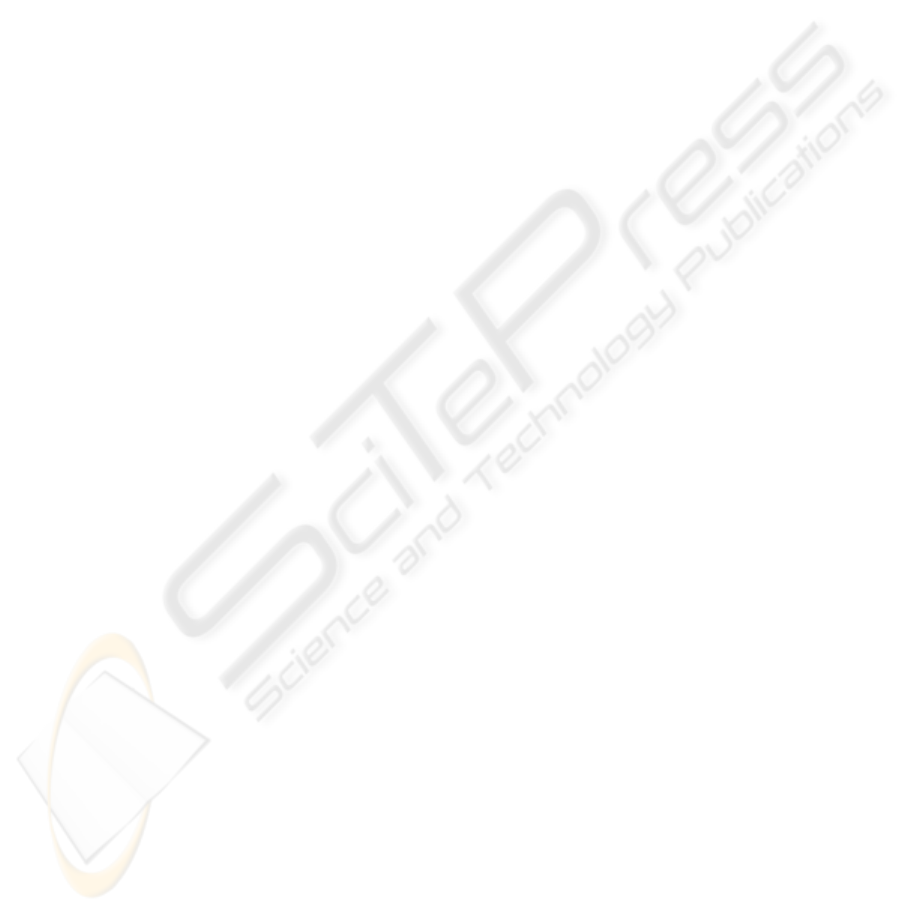
but it was Zibulvesky et al. who noticed that in the case of sparse sources, their lin-
ear mixtures can be easily separated using very simple ”geometric” algorithms. Sparse
representations can be used in blind source separation. When the sources are sparse,
smaller coefficients are more likely and thus for a given data point t. if one of the
sources is significantly larger, the remaining ones are likely to be close to zero. Thus
the density of data in the mixture space, besides decreasing with the distance from the
origin shows a clear tendency to cluster along the directions of the basis vectors. Spar-
sity is good in ICA for two reasons. First the statistical accuracy with which the mixing
matrix A can be estimated is a function of how non-Gaussian the source distributions
are. Thus, roughly speaking the sparser the sources are the less data is needed to esti-
mate A. Secondly the quality of the source estimates given A, is also better for sparser
sources.
A signal is considered sparse when values of most of the samples of the signal do
not differ significantly from zero. These are from sources that are minimally active.
Zibulevsky et al. have demonstrated that when the signals are sparse, and the sources
of these are independent, these may be separated even when the number of sources
exceeds the number of recordings [4]. The over-complete limitation suffered by nor-
mal ICA is no longer a limiting factor for signals that are very sparse. Zibulevsky also
demonstrated that when the signals are sparse, it is possible to determine the number of
independent sources in a mixture of unknown signal numbers. One application where
the use of blind source separation for SEMG is required is when the signal strength is
very small, and the sources are minimally active, such as during maintained posture.
This leads to the argument of the use of Zibulevsky’s ICA technique to separate muscle
activity originating from muscles that are minimally active. It also provides the basis
for identifying the number of active independent sources in the mixture to validate the
use of ICA for SEMG application.
3.1 Identification of Sources using Plotting of Sparse Data
Zibulvesky et al. developed a simple probabilistic method for over determined ICA
source separation. For a more general case they used maximum aposteriori approach
which includes the situation of over complete dictionary and more sources than sen-
sors. They have also demonstrated the combination of clustering and shortest path de-
composition technique to be faster and more robust. This required the estimation of
the mixing matrix before hand by clustering and then reconstruction of the sources by
shortest path decomposition. They demonstrated the separation of up to six different
audio sounds mixed into two mixtures (recordings). During maintained posture of the
unloaded hand, muscles are minimally active and the SEMG signal strength is very
small. Hence SEMG is expected to be sparse in these conditions. The plotting of the
recording against each other would be expected to demonstrate the number of indepen-
dent sources. As the first stage, it is necessary to suitably linearly transform and filter the
signals to ensure the signals are sufficiently sparse. There are number possible methods
that are available. Most common one is spectral filtering of the data for the signal to be
sparse in the time domain. The filter properties such as frequency and order needs to be
selected according to the frequency content of the signal. Typically, while maintaining
the properties of the original signal, and desiring to make the signal sparse, filtering is
56