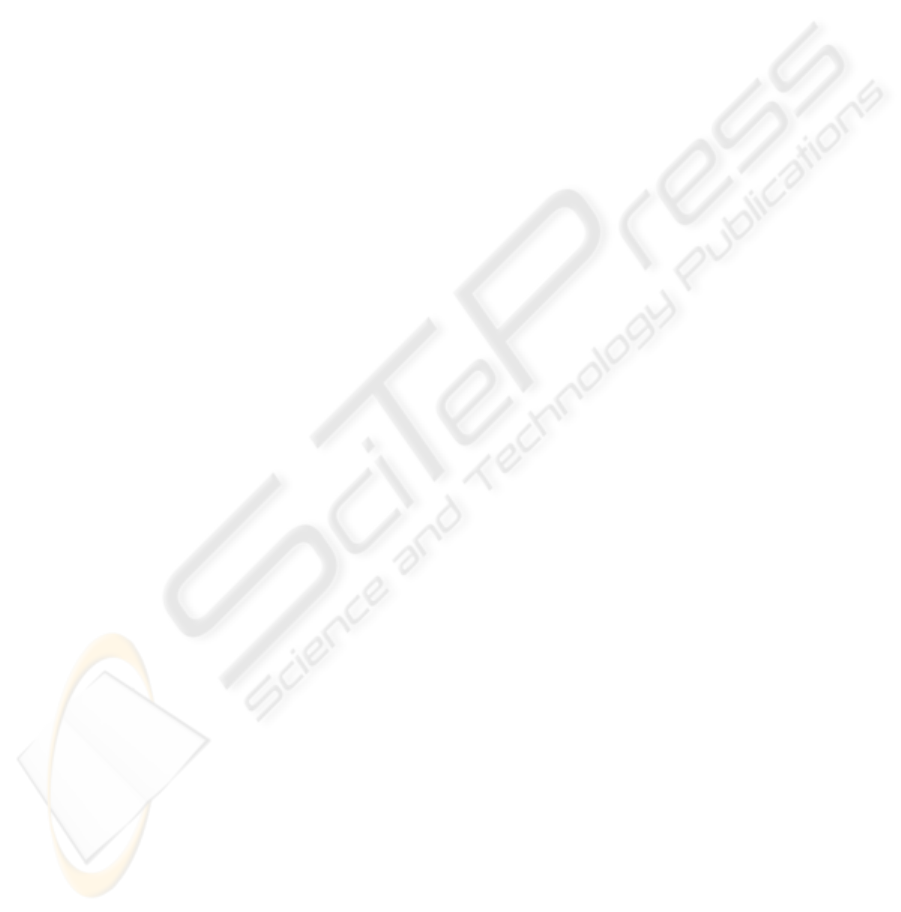
taken to avoid injurious falls. Also, such nonlinear index could potentially be used as
gait diagnostic parameter in clinical situation.
In this study, MFC data from steady-state gait have been used to characterize gait
patterns. There are two major reasons for this. Firstly, MFC provides a more sensitive
measure of motor function of the locomotor system compared to some gross overall
kinematic descriptions of gait such as joint angular changes or stride phase times,
secondly its close linkage with tripping falls [8]. Furthermore, long-term MFC data,
as used in this study, are required so that variability indexes of MFC having long
range correlation could be
captured representative of the real gait performance.
Our results suggest that gait pathologies with falls and balance problems are re-
flected in Poincaré plots and features extracted from these plots are effective in dif-
ferentiating between healthy and falls-prone gaits. Poincaré plots were used in our
earlier study for young-old gait classification [6]. In this study, such analysis has been
extended to identifying elderly with a history of falls and balance problems. More-
over, nonlinear parameter like ApEn has been applied in this study in identifying gait
characteristics. Although both Poincaré plot indexes and ApEn were effective in
discriminating the gait characteristics patterns, results of our present study suggest
that ApEn could perform better than Poincaré plot indexes in identifying gait pattern.
One possible reason why a nonlinear index like ApEn could be a more effective gait
identifier might be that physiologic control mechanism of healthy human gait is
nonlinear and correlated. However, higher ApEn values displayed in the fallers group
might be an indication of the breakdown of locomotor control mechanism in the falls-
risk elderly. ApEn reflects irregularity, randomness and complexity of the MFC time
series data, and would therefore, indicate the stability in the control of foot motion
over the ground.. In a previous study involving gait, Costa et al [7] applied multi-
scale entropy (MSE) for analysing gait with different speeds and studied the scaling
effect on sample entropy for different walking rates. Sample entropy (SampEn) on
single scale in a healthy walking time series was found to be the lowest value in that
study [7]. Although both SampEn and ApEn quantify the regularity of a time series,
methods of calculation are different [5]. However, ApEn results of this study sug-
gests that the pathologic gait having higher ApEn is more random than healthy physi-
ologic gait having lower ApEn. Nonlinear index like ApEn which probe a dynamical
property of human gait control, has, therefore, implications for quantifying and mod-
elling gait control under various conditions. Further investigation should be carried
out to derive additional nonlinear variability indexes of human gait.
Acknowledgements
MFC gait data for this study were taken from VU Biomechanics Unit. Several people
have contributed to the creation of the gait database, especially Simon Taylor of the
VU Biomechanics Unit.
150