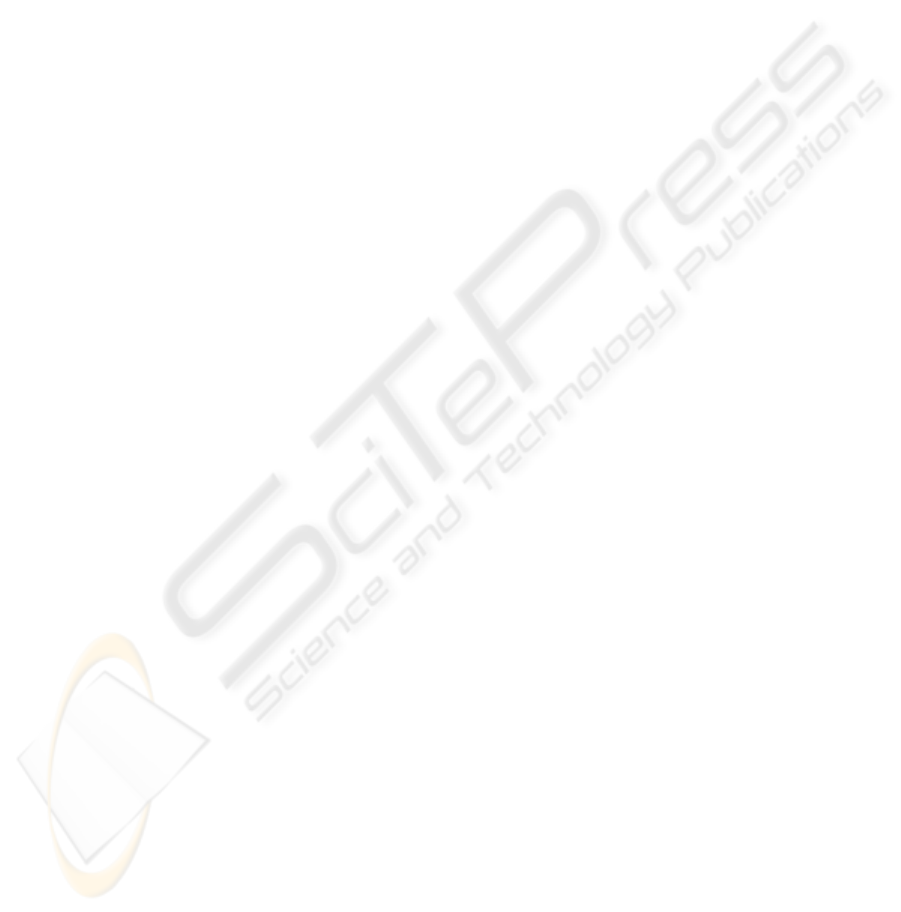
6 CONCLUSION
The supervised learning method SUCRAGE allows
to generate classification rules then to exploit them
by an inference engine which implements a basic
reasoning or an approximate reasoning. The
originality of our approach lies in the use of
approximate reasoning to refine the learning: this
reasoning is not only considered any more as a
second running mode of the inference engine but is
considered as a continuation of the learning phase.
Approximate reasoning allows to generate new
wider and more general rules. Thus imprecision of
the observations are taken into account and
problems due to the discretization of continuous
attributes are eased. This process of learning
refinement allows to adapt and to improve the
discretization. The initial discretization is regular, it
is not supervised. It becomes, via the approximate
reasoning, supervised, as far as the observations are
taken into account to estimate their adequacy to
rules and as far as the belief degrees of these new
rules are then computed on the whole training set.
Moreover the interest of this approximate generation
is that the new base of rules is then exploited by a
basic inference engine, easier to interpret. Thus
approximate reasoning complexity is moved from
the classification phase (a step that has to be
repeated) to the learning phase (a step which is done
once). The realized tests lead to satisfactory results
as far as they are close to those obtained with a basic
generation of rules exploited by an approximate
inference engine.
The continuation of the work will focus on the
first method of new rules generation (with constant
number of rules) to make it closer to what takes
place during approximate inference. The search for
other forms of g-distance can turn out useful notably
to be able to obtain results of generation between the
g-threshold value 0 (where we remain too close to
the observation) and the g-threshold value 1 (where
we go away too many "surroundings" of the
observation). The second method, which enriches
the base of rules with all the new rules, is penalized
by the final size of the obtained base. An interesting
perspective is to bend over the manners to reduce
the number of rules without losing too much
classification performance.
REFERENCES
Borgi A., 2005. Différentes méthodes pour optimiser le
nombre de règles de classification dans SUCRAGE .
3
rd
Int. Conf. Sciences of Electronic, Technologies of
Information and Telecom. SETIT 2005, 11 p., Tunisia.
Borgi A, Akdag H., 2001. Apprentissage supervisé et
raisonnement approximatif, l’hypothèse des
imperfections. Revue d’Intelligence Artificielle, vol
15, n°1, pp 55-85, Editions Hermès.
Borgi A., Akdag. H., 2001. Knowledge based supervised
fuzzy-classification : An application to image
processing. Annals of Mathematics and Artificial
Intelligence, Vol 32, p 67-86.
Borgi A., 1999. Apprentissage supervisé par génération
de règles : le système SUCRAGE, Thèse de doctorat
(PhD thesis), Université Paris VI.
Duch W., R. Setiono, J.M. Zurada, 2004. Computational
Intelligence Methods for Rule-Based Data
Understanding. Proceedings of the IEEE, Vol. 92, 5.
El-Sayed M., Pacholczyk D., 2003. Towards a Symbolic
Interpretation of Approximate Reasoning. Electronic
Notes in Theoretical Computer Science, Volume 82,
Issue 4, Pages 1-12.
Gupta M. M., Qi J., 1991. Connectives (And, Or, Not) and
T-Operators in Fuzzy Reasoning. Conditional
Inference and Logic for Intelligent Systems, 211-233.
Haton J-P., Bouzid N., Charpillet F., Haton M., Lâasri B.,
Lâasri H., Marquis P., Mondot T., Napoli A., 1991 Le
raisonnement en intelligence artificielle. InterEditions.
Kohavi R., 1995. A Study of Cross-Validation and
Bootstrap for Accuracy Estimation and Model
Selection, Proc. of the Fourteenth International Joint
Conference on Artificial Intelligence, Vol. 2.
Michalski R.S., S. Ryszard, 1983. A theory and
methodology of inductive learning. Machine
Learning : An Artificial Intelligence Approach, Vol. I,
83-134.
Nozaki K., Ishibuchi H., Tanaka H., 1994. Selecting
Fuzzy Rules with Forgetting in Fuzzy Classification
Systems. Proc. 3rd IEEE. Conf. Fuzzy Systems Vol. 1.
Pearl. J., 1990. Numerical Uncertainty In Expert Systems.
Readings in Uncertain Reasoning, Ed. by Shafer and
Pearl. Morgan Kaufman publishers. California.
Ruspini E., 1991. On the semantics of fuzzy logic.
International Journal of Approximate Reasoning, 5.
Vernazza G., 1993. Image Classification By Extended
Certainty Factors. Pattern Recognition, vol. 26, n° 11,
p. 1683-1694, Pergamon Press Ltd.
Yager R.R., 2000. Approximate reasoning and conflict
resolution. International Journal of Approximate
Reasoning, 25, p. 15-42, Elsevier.
Zadeh L.A., 1979. A Theory of Approximate Reasoning.
Machine Intelligence, vol. 9, p. 149-194.
Zhou Z. H., 2003. Three perspectives of data mining.
Artificial Intelligence 143, 139–146, Elsevier.
ICSOFT 2006 - INTERNATIONAL CONFERENCE ON SOFTWARE AND DATA TECHNOLOGIES
210