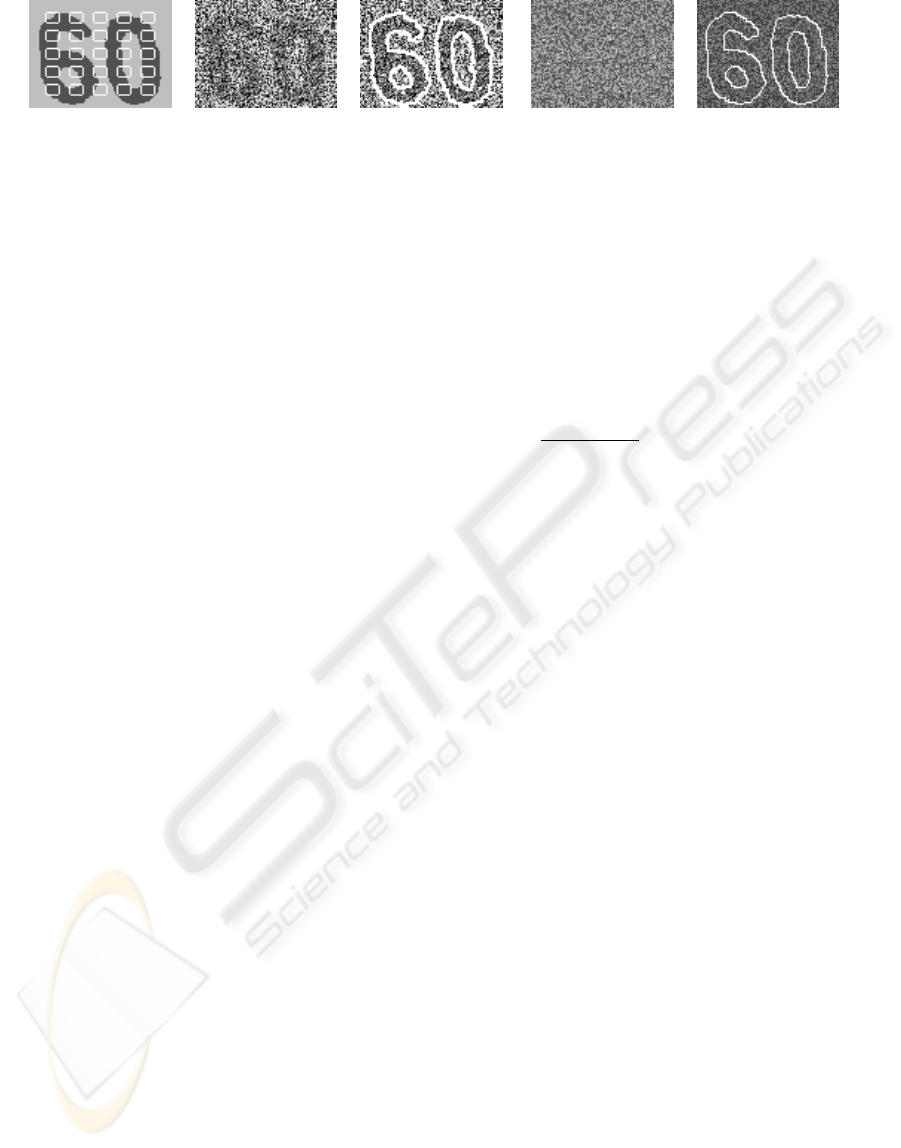
(a) (b) (c) (d) (e)
Figure 1: (a) Synthetic image without noise (72 × 96 pixels) with initial contour. (b) Noisy version of image (a), pdf of the
background and object gray levels are shown in Fig. 2. (d) Noisy version of image (a), gray levels of the object region are
distributed with a Gaussian pdf and gray levels of the background have a bi-valuated pdf with the same mean and variance
values as those of the object region. (c), (e) Final contour respectively obtained on image (b) and (d) with the proposed
approach.
3 EXPERIMENTAL RESULTS
We show in Fig.1c a result of segmentation on an im-
age quantized with Q = 256 levels. The noisy image
in Fig.1b has been generated with a step function of
order q =4for the pdf models of the object and the
background. One can see the histograms in Fig.2a and
Fig. 2b in solid line. The minimum value of the sto-
chastic complexity is obtained for q =4(Fig.2a and
Fig.2b in dotted line) which corresponds to the true
value. In Fig.1e, we show the segmentation result on
an image with a Gaussian noise on the object region
and a bi-valuated noise on the background region cho-
sen in order to get the same mean and variance values
in both regions.
An example of application of this approach to a
RGB image is shown in Fig.3a. The final contour Γ is
obtained on the saturation component S in HSV rep-
resentation. One can see in Fig.3c the final contour
on a real SAR image corrupted with speckle noise. In
Fig. 4, one can see a segmentation result on a textured
image. In order to get homogeneous regions, the im-
age s has been preprocessed to obtain the new image
f defined by f (x, y)=F ∗ s(x, y) where F is the
Roberts filter (Jain, 1989) defined with 3 × 3 pixel
neighborhoods and ∗ is the convolution operator.
4 ROBUSTNESS OF THE
PROPOSED TECHNIQUE
Let us analyse in this section the efficiency of the pro-
posed nonparametric approach pdf estimation based
on irregular step functions. For that purpose, we com-
pare the results obtained either with this approach or
with a parametric modelization of the actual pdf of the
background and object gray levels which will be dis-
tributed with a pdf which belongs to the exponential
familly (Martin et al., 2004).
The results shown in Fig.5 demonstrate that the
proposed approach leads to segmentation results
equivalent to those obtained with a parametric model
for the pdf which is adapted to the noise which
describe the gray level fluctuations. On the other
hand, if an inadapted parametric model is used for
the pdf, one can see that poor results can be ob-
tained. It has been shown (Goudail et al., 2003) for
polygonal (Ruch and R
´
efr
´
egier, 2001) and level set
snakes (Martin et al., 2004) that the Bhattacharyya
distance (Cover and Thomas, 1991), defined by B =
− log
P
t
(z)P
b
(z)dz, is an appropriate measure
of contrast between the target and the background.
We have thus determined the average number of
misclassified pixels (ANMP) obtained as a function
of B on the images described in Fig.5 for the pre-
vious discussed parametric and nonparametric ap-
proaches. The number of misclassified pixels is de-
termined from the final contour by counting the pixels
which belong to the true target shape but lie outside
the contour, and those which belong to the true back-
ground but lie inside the contour. Both approaches
lead to equivalent ANMP (with a precision lower than
1.7%) when B≥0.29. The parametric statistical ap-
proach leads to lower ANMP than the proposed non-
parametric statistical technique only when B < 0.29.
5 CONCLUSION
We have proposed a nonparametric statistical level
set snake based on the minimization of the stochas-
tic complexity. We have demonstrated that this ap-
proach leads to good segmentation results when the
pdf of the object and the background are approxi-
mated by a step function whose values and order are
estimated. This approach leads to minimize a crite-
rion without free parameter to be tuned by the user.
We have seen that this technique provides good results
on SAR, video (color) and textured images. More-
over, we have shown that the segmentation results of
the proposed approach are closed to those obtained
with a parametric statistical approach but with a bet-
ter robustness.
There exists different perspectives to this work. It
will be interesting to generalize this technique to a
multi region approach and to take into account possi-
ble spatial correlations.
NONPARAMETRIC STATISTICAL LEVEL SET SNAKE BASED ON THE MINIMIZATION OF THE STOCHASTIC
COMPLEXITY
465