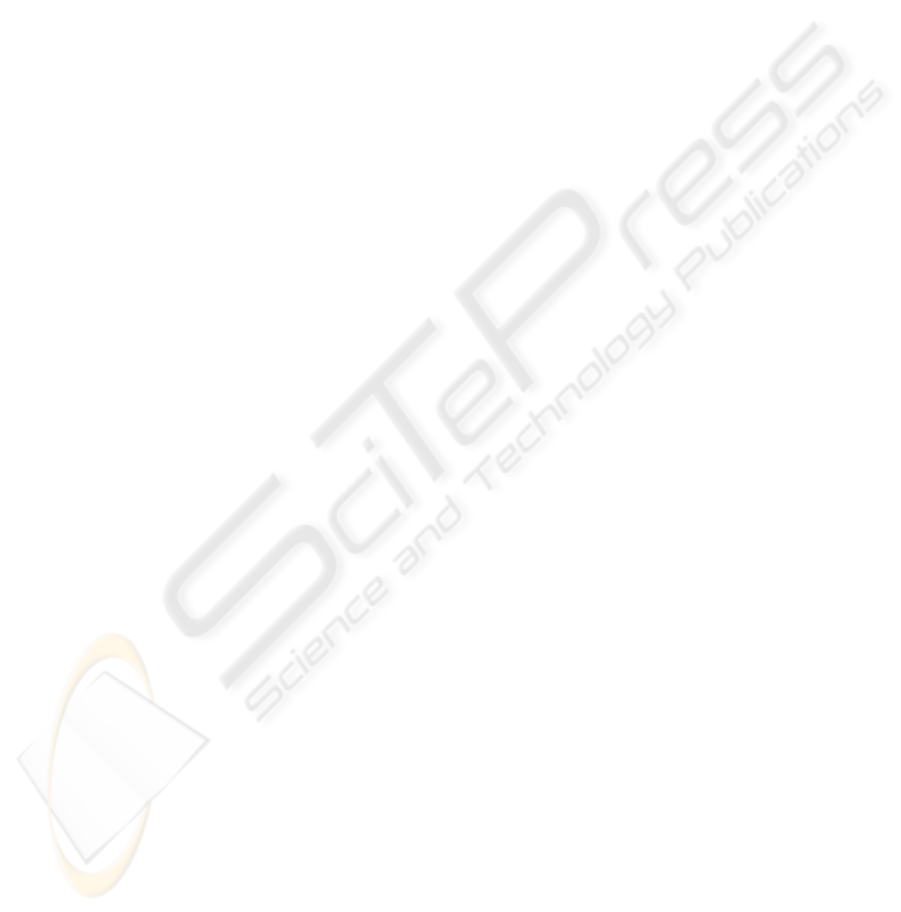
in section 4.2 is shown in Fig 5(d), where the groups
of the initial grouping procedure are refined in order
to form the final groups which will be classified
with the use of the information obtained by the DOC
of each CC. Final extracted areas, from all color
planes, considered as text are shown in Fig 5(e).
The second experimental result is presented in
Fig. 6 which is a color document of a book cover. In
this example vertically and horizontally aligned text
coexists. The grouping of the CCs that corresponds
to the color plane of the vertically aligned text is
shown in Fig. 6(c) and Fig. 6(d). The final result
(Fig. 6(e)) shows that the method successfully
extracted text of both orientations.
7 CONCLUSIONS
Text localization is an important processing in
computer vision systems, especially for document or
text image related applications. In this paper, we
have presented a new method for text localization
which is suitable for complex cover pages and any
type of color documents. In these cases of document
images text and graphics are highly mixed with the
background. The proposed technique efficiently
integrates a KSOM color quantization procedure and
a color plane text localization technique. The
proposed technique is robust and has the following
characteristics:
• Preferable estimation of the number of
dominant colors
• Color reduction by using the KSOM neural
network.
• Splitting the color document image into a
number of binary images, called color
planes, corresponding to the dominant colors
obtained.
• In every color plane, CCs are spatially
formed in groups with the use of local
information in an adaptively defined area.
• The information that is used of each CC for
classification involves not only the closest
neighbors but a large number of similar CCs.
First results on a large number of data set of above
500 color text images collected from Internet, most
of which are color cover pages, are very
encouraging.
REFERENCES
Atsalakis, A., Papamarkos, A. and Andreadis, I., 2002.
On estimation of the number of image principal colors
and color reduction through self-organized neural
networks, Int. Journal of Imaging Systems and
Technology, 12(3), 117-127.
Chen, W.Y. and Chen, S.Y., 1998. Adaptive page
segmentation for color technical journals’ cover
images, Image and Vision Computing, 16(12-13),
855-877.
Fletcher, L. and Kasturi, R., 1988. A robust algorithm for
text string separation from mixed text/graphics
images, IEEE Trans. PAMI, 10(6), 910–918.
Hase, H., Shinokawa, T., Yoneda, M. and Suen, C.Y.,
2001. Character string extraction from color
documents, Pattern Recognition, 34(7), 1349–1365.
Jain, A.K. and Zhong, Y., 1996. Page Segmentation Using
Texture Analysis, Pattern Recognition, 29(5), 743-
770.
Jain, A.K. and Bhattacharjee, S., 1992. Text segmentation
using Gabor Filters for automatic document
processing, Mach. Vision Appl., 5, 169–184.
Jung, K. and Han, J., 2004. Hybrid approach to efficient
text extraction in complex color images. Pattern
Recognition Letters, 25(6), 679-699.
Jung, K., Kim, K.I. and Jain, A.K., 2004. Text information
extraction in images and video: A survey, Pattern
Recognition, 37(5), 977-997.
O’Gorman, L., 1993. The Document Spectrum for Page
Layout Analysis, IEEE Trans. PAMI, 15(11), 1162-
1173.
Papamarkos, N., 1999. Color reduction using local
features and a SOFM neural network, Int. Journal of
Imaging Systems and Technology, 10(5), 404-409.
Simon, A., Pret, J.C. and Johnson, A.P., 1997. A Fast
Algorithm for Bottom-Up Layout Analysis, IEEE
Trans. Pattern Analysis and Machine Intelligence,
19(3), 273-277.
Sobottka, K. et al., 2000. Text Extraction from Colored
Book and Journal Covers, International Journal on
Document Analysis and Recognition, 2(4), 163-176.
Strouthopoulos, C., Papamarkos, N. and Atsalakis, A.,
2002. Text extraction in complex color documents.
Pattern Recognition, 35(8), 1743-1758.
Zhong, Y., Karu, K., Jain, A.K., 1995. Locating text in
complex color images, Pattern Recognition, 28 (10),
1523–1535.
TEXT LOCALIZATION IN COLOR DOCUMENTS
187