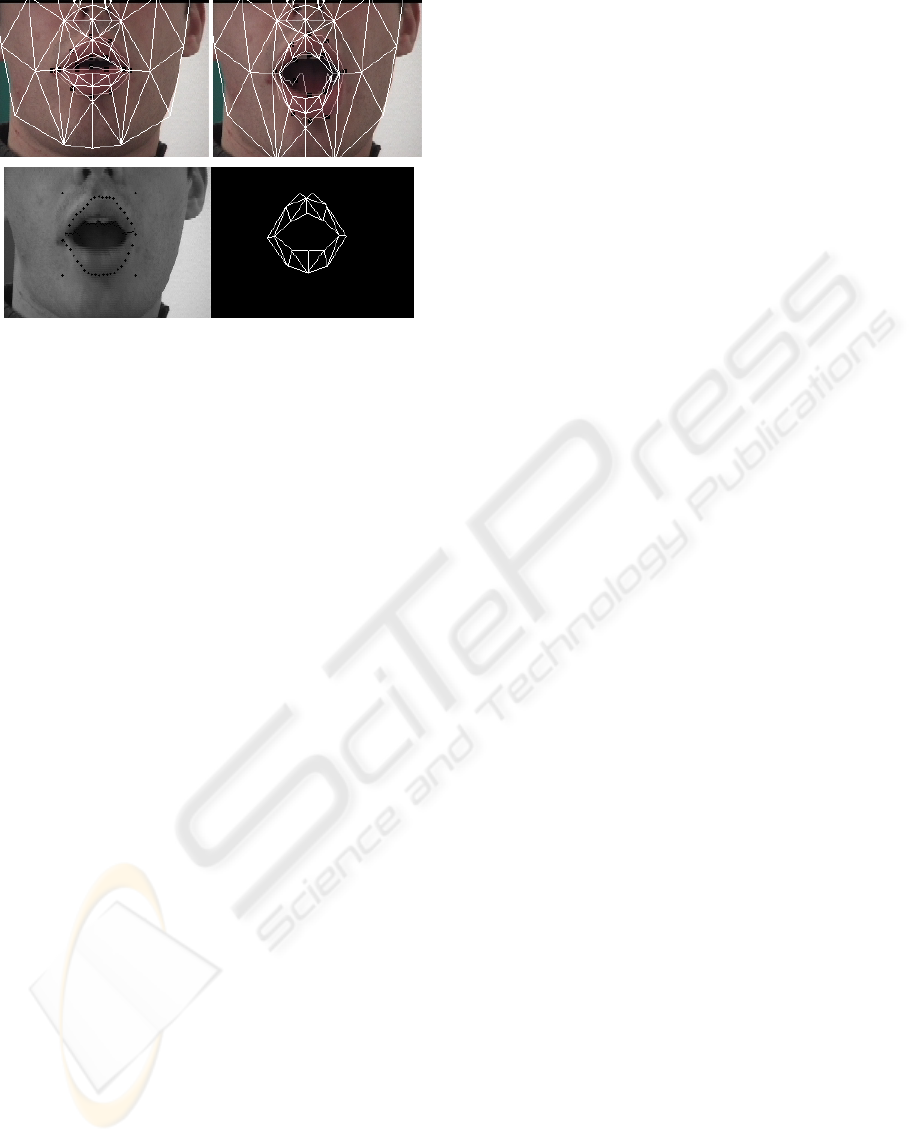
Figure 7: Regularisation through the 3D model: a) Good
match between segmentation and model; b) Bad match: a
control point of the inner snake is clearly erroneous and
is corrected by the 3D mesh corresponding position ; c)d)
After correction through feedback: front view with 3D lip
model reshaped.
and lip separation, K-means classification with 3 hue
classes, non linear filter for BB initial localisation,
motion estimation via LK algorithm for Bounding
Box tracking, snake initialisation deduced from the
BB position, snake convergence towards inner and
outer lip contours. The (eventually) badly segmented
points (detected by a distance measure on the 3D
model) are re-processed (thanks to the closed-loop).
Currently, we simply replace the bad point by the cor-
responding point of the model. But we will now im-
plement a reprocessing on that pixel (zoom-in in the
neighborhood and local re-computation in that little
area).
Indeed we still have some time left to spend at this
reprocessing: the whole analysis algorithm, imple-
mented in non optimised C-code on an i386 processor
at 1.4GHz, works in real-time (i.e. processing rate
better than 30Hz). This is 30 times faster than the
algorithm presented in (Li
´
evin and Luthon, 2004).
Another direction of our current research is to seg-
ment not only the lips, but also other face features
(namely nostrils, eyes, eyebrows and ears). This will
help to get other relevant points for 3D model scal-
ing (cf. user-dependent facial geometry taken into ac-
count at initialisation step), for regularisation of poor
input data (cf. re-segmentation through the feedback
loop that adds rigidity constraints and other seman-
tics to the ill-posed problem at the pixel side) and for
more realistic animation (cf. face expressions during
speech).
Moreover, having more information on the whole
face may enable a better understanding of some spo-
ken phonemes (e.g. to make the difference between
French phonemes [e] and [y], the nose position is
very important for animation: indeed, from a visual
perception viewpoint, the mouth has almost the same
shape in both cases. The only difference is that for
phoneme [y], the mouth is closer to the nose
Finally, as we want to be able to animate various
clones and propose a generic solution, we are also
working on the use of MPEG4-compliant 3D models
(using FDP and FAP, facial animation parameters).
REFERENCES
Batur, A. U. and Hayes, M. H. (2005). Adaptive active ap-
pearance models. IEEE Trans. on Image Processing,
14(11):1707–1721.
Beno
ˆ
ıt, C., Lallouache, T., Mohamadi, T., and Abry, C.
(1992). A set of French visemes for visual speech
synthesis. In Bailly, G., Beno
ˆ
ıt, C., and Sawallis,
T., editors, Talking Machines: Theories, Models and
Designs, pages 485–504, Amsterdam, North-Holland.
Elsevier Science Publishers B.V.
Cootes, T., Edwards, G., and Taylor, C. (1998). Ac-
tive appearance models. In European Conference on
Computer Vision, ECCV, volume 2, pages 484–498.
Springer-Verlag.
Erdem, C. E., Sankur, B., and Tekalp, A. M. (2004).
Performance measures for video object segmentation
and tracking. IEEE Trans. on Image Processing,
13(7):937–951.
Erdem, C. E., Tekalp, A. M., and Sankur, B. (2003). Video
object tracking with feedback of performance mea-
sures. IEEE Trans. on Circuits and Systems for Video
Technology, 13(4):310–324.
Fu, Y., Erdem, A. T., and Tekalp, A. M. (2000). Track-
ing visible boundary of objects using occlusion adap-
tive motion snake. IEEE Trans. on Image Processing,
9(12):2051–2060.
Kass, M., Witkin, A., and Terzopoulos, D. (1987). Snakes:
Active contour models. International Journal of Com-
puter Vision, pages 321–331.
Li
´
evin, M., Delmas, P., Coulon, P. Y., Luthon, F., and
Fristot, V. (1999). Automatic lip tracking: Bayesian
segmentation and active contours in a cooperative
scheme. In IEEE Int. Conf. on Multimedia Computing
and Systems (ICMCS’99), pages 691–696, Firenze,
Italy. Vol. 1.
Li
´
evin, M. and Luthon, F. (2004). Nonlinear color space
and spatiotemporal MRF for hierarchical segmenta-
tion of face features in video. IEEE Transactions on
Image Processing, 13(1):63–71.
Mirmehdi, M., Palmer, P. L., Kitler, J., and Dabis, H.
(1999). Feedback control strategies for object recog-
nition. IEEE Trans. on Image Processing, 8(8):1084–
1101.
Pichler, O., Teuner, A., and Hosticka, B. J. (1998). An un-
supervised texture segmentation algorithm with fea-
ture space reduction and knowledge feedback. IEEE
Trans. on Image Processing, 7(1):53–61.
REAL-TIME LIPTRACKING FOR SYNTHETIC FACE ANIMATION WITH FEEDBACK LOOP
407