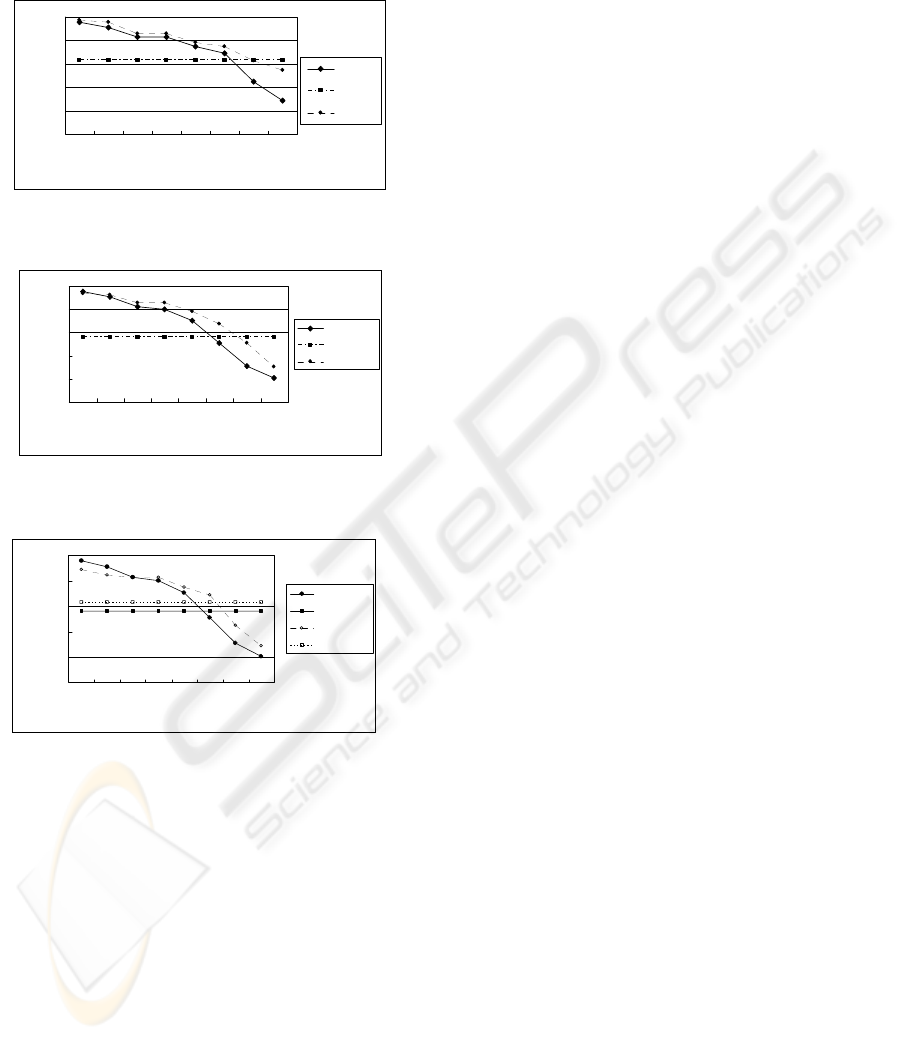
The video stream is a sequence of images with
resolution of 100 × 75 pixel from the database.
Before we compute the motion estimation, some
techniques are applied to the images in order to
make the computation convenient and increase the
precision of the motion estimation. In our system,
original 100 × 75 pixel image is extracted as a 100 ×
72 pixel window around the center of mass for
computational convenience.
Speech noise is added by using random noise at
various SNRs. The system is trained on clean speech
and tested under noisy conditions. The HMM-based
recognizer implemented in MATLAB on Pentium-
IV PC, using 4 states for Mandarin digits and 5
states for English digits. The time for feature
extraction is under 6 seconds, and HMM parameter
training spends about 10 seconds.
Initial results for the clean speech are good.
Figure 5 and 6 show the results for English and
Mandarin digits recognition, respectively. Figure 7
shows the comparison for English and Mandarin
digits recognition at audio-only and visual-only
feature situation. This result shows that the
Mandarin digits recognition gets higher correct rate
under audio-only features than English digits, the
English digits recognition gets better correct rate
under visual-only features than Mandarin digits.
5 CONCLUSIONS
In this paper, our focus is to construct a new audio-
visual database and a lip-motion based feature
extractor for the recognition system with a HMM
based recognizer. The experimental results show a
comparison between English and Mandarin speech
recognition, and the improvement of using both
audio and visual feathers.
The results for our proposed approach at the
various SNRs for the speech show that the method
including the visual or lip features produces a better
performance than using the audio-only features. In
the future, we will try to improve the overall
recognition rate using other more robust features and
recognizers.
REFERENCES
T. Chen, “ Audio-visual speech processing,” in IEEE
Signal Processing Magazine, Jan. 2001
T. Chen and R. Rao, “Audiovisual interaction in
multimedia communication,” in ICASSP, vol. 1.
Munich, Apr. 1997, pp. 179-182.
C. C. Chibelushi, F. Deravi, and J. S. D. Mason, “A
review of speech-based bimodal recognition,” in IEEE
Trans. Multimedia, vol. 4, Feb. 2002, pp. 23-37.
M. N. Kaynak, Q. Zhi, etc, “Analysis of Lip Geometric
Features for Audio-Visual Speech Recognition,” IEEE
Transaction on Systems, Man, and Cybernetics-Part
A: Systems and Humans, vol. 34, July 2004, pp. 564-
570.
J. Luettin and G. Potamianos and C. Neti, “Asynchronous
stream modeling for large vocabulary audio-visual
speech recognition,” 2001.
I. Matthews, T. F. Cootes, J. A. Bangham, S. Cox, and R.
Harvey, “Extraction of visual features for lipreading,”
in IEEE Trans. pattern analysis and machine
intelligence, vol. 24, 2002, pp. 198-213.
Figure 5: Comparison of recognition rate using
different features at various noise levels for digits
in English.
0
20
40
60
80
100
clean 30dB 25dB 20dB 15dB 10dB 5dB 0dB
SNR
Correct Rate
Audio-only
Visual-only
Audio-visual
0
20
40
60
80
100
clean 30dB 25dB 20dB 15dB 10dB 5dB 0dB
SNR
Correct Rate
Audio-only
Visual-only
Audio-visual
Figure 6: Comparison of recognition rate using
different features at various noise levels for digits
Figure 7: Comparison of the English and Mandarin
digits recognition rate at various features and noise
levels. (Solid lines are for English, and dashed line
are for Mandarin).
0
20
40
60
80
100
clean 30dB 25dB 20dB 15dB 10dB 5dB 0dB
SNR
Correc t Rate
Audio-only
Visual-only
Audio-only
Visual-onl
AN AUDIO-VISUAL SPEECH RECOGNITION SYSTEM FOR TESTING NEW AUDIO-VISUAL DATABASES
195