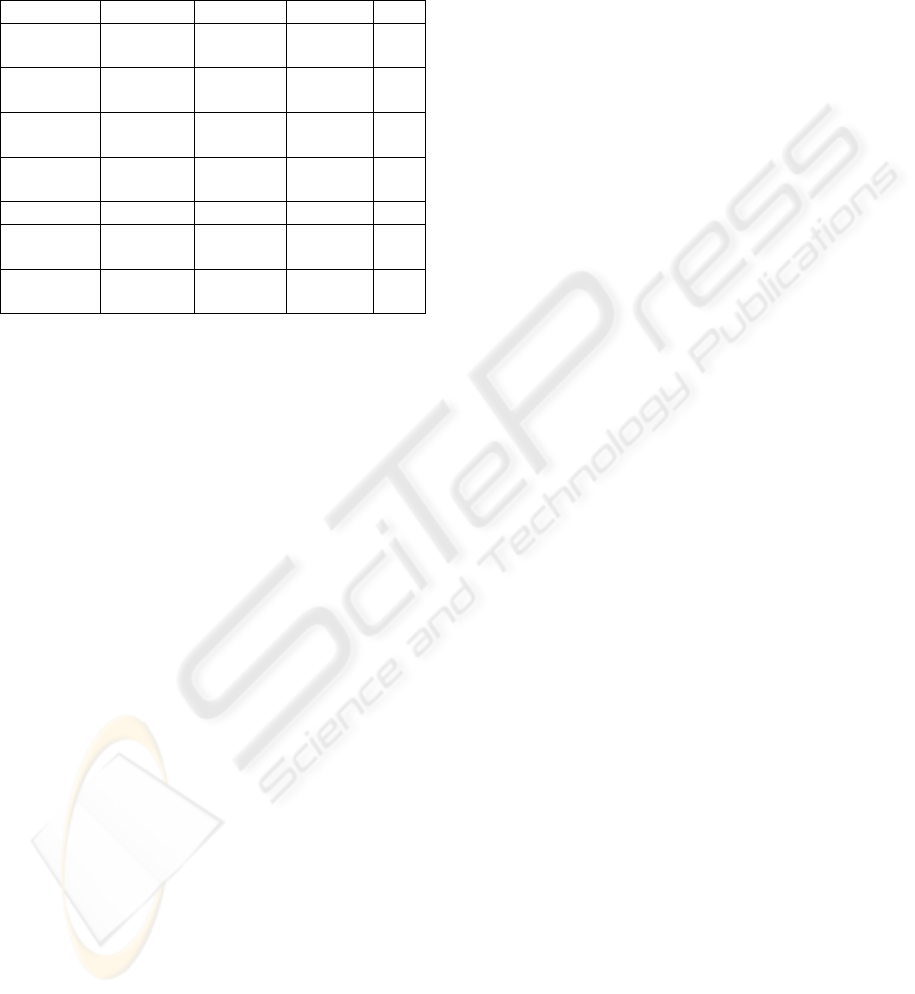
Table 1: Varying deviations of γ
i
or (x
i
,yi) over 100 tests
and their influence on the absolute deviation of pose estima-
tion parameters. (sub row 1=mean, sub row 2=max). The
right column shows the number of images that have been
erroneously localized.
∆γ
i
∆x
c
(m) ∆y
c
(m) ∆γ
c
(˚) bad
0˚ 0.0007 0.0060 0.0917 0
0.0677 0.0597 1.7762
2˚ 0.0110 0.0127 1.0485 1
0.0523 0.0998 2.3090
5˚ 0.0294 0.0305 2.4981 3
0.1743 0.1163 4.2628
10 ˚ 0.0501 0.0647 4.8415 4
0.1820 0.2830 7.3625
∆(x
i
,yi) ∆x
c
(m) ∆y
c
(m) ∆γ
c
(˚) bad
0.2m 0.0467 0.0516 1.1115 2
0.1895 0.1645 3.6326
0.5m 0.1321 0.1455 1.6100 4
0.7256 0.4124 7.7750
inside three different rooms (approx. surface: 60m2)
containing 63 main vertical lines (wall corners, win-
dows and doors borders, racks and desks) whose posi-
tions are measured by hand. The fig 7.b shows a part
of the indoor environment used in this experiment.
We processed 40 images randomly selected from a
sequence of 150 frames acquired at 15fps. 32 im-
ages were correctly localized, the position of the cam-
era being estimated inside a 20cm tolerance (We use
the tiled floor to localize approximately the camera as
ground thrust). γ
c
is compared with the angle given
by an electronic compass whose accuracy is about 2 ˚ .
The maximum detected orientation error for γ
c
in the
32 images is less than 4 ˚ . The 8 images which have
been erroneously localized can geometrically corre-
spond to different locations due to outliers. However
when we use the complete sequence to track the pose
and the correspondences between vertical lines and
γ
i
, all the 150 images are correctly localized.
The detection of the lines images takes about 40 ms
(mean time for 150 images for approx 20000 contour
points and up to 100 lines to detect). The compu-
tation of (ˆα,
ˆ
β) is generally achieved in less than 1
ms. The 2D pose estimation time greatly depends of
the complexity of the 2D map and the number of the
detected vertical lines. In our experiments, the local-
ization of the first image of the sequence has needed
1.2 sec. The tracking of the following poses, how-
ever, has been achieved in a few ms. During the first
two seconds, the computer processes the first pose and
caches the incoming images. Then it processes the
cached images more quickly than the acquisition rate
and thus can localize in realtime at 15 fps after about
three seconds of initialization.
5 CONCLUSION
In this paper, we have proposed an original method to
detect the pose of a central catadioptric camera from
an image of a indoor environment containing verti-
cal lines. The two axes orientation detection which
is first applied can be used in others applications to
detect arbitrary sets of parallel lines. The 2D pose
estimation, in spite of its apparent simplicity has ex-
hibited an high computational complexity due to the
presence of outliers and unknown matches. We have
proposed improvements allowing to achieve the pose
estimation in realtime using a smart selection of the
correspondences between the lines in the 2D map and
the detected vertical lines. Realtime is also obtained
thanks to a caching of the images and a tracking of
the correspondences inside the sequence. As future
work, we plan to integrate colorimetric information to
avoid false detections that are geometrically correct
and to accelerate the search of the correspondences
by discarding incompatible matches. Methods based
on 1D Panoramas (Briggs 2005) will also be investi-
gated. Then, experiments on entire buildings will be
achieved to validate the approach at a wide scale.
REFERENCES
X. Ying, Z. Hu (2004). Catadioptric Line Features De-
tection using Hough Transform. In Proceedings of
the 17th International Conference on Pattern Recog-
nition, volume 4, pp 839-842, 2004.
P. Vasseur and E. M. Mouaddib (2004). Central Catadiop-
tric Line Detection. In BMVC, Kingston, Sept 2004.
T.Pajdla, V.Hlavac (2001). Image-based self-localization by
means of zero phase representation in panoramic im-
ages. In Proceedings of the 2nd International Confer-
ence on Advanced Pattern Recognition, March 2001.
C. Geyer and K. Daniilidis (2001). Catadioptric Projective
Geometry. In International Journal of Computer Vi-
sion , 45(3), pp. 223-243, 2001.
C. Geyer and K. Daniilidis (1999). Catadioptric Camera
Calibration. In Proceedings of the 7th International
Conference on Computer Vision, volume 1, p. 398,
1999.
R. Benosman and S. B. Kang (2001). Panoramic Vision.
Springer, 2001.
A. Briggs, Y. Li and D. Scharstein (2005). Feature Match-
ing Across 1D Panoramas. In Proceedings of the OM-
NIVIS, 2005.
O. Shakernia, R. Vidal, S. Sastry (2003). Structure from
Small Baseline Motion with Central Panoramic Cam-
eras. In the Fourth Workshop on Omnidirectional Vi-
sion, 2003
REALTIME LOCALIZATION OF A CENTRAL CATADIOPTRIC CAMERA USING VERTICAL LINES
421