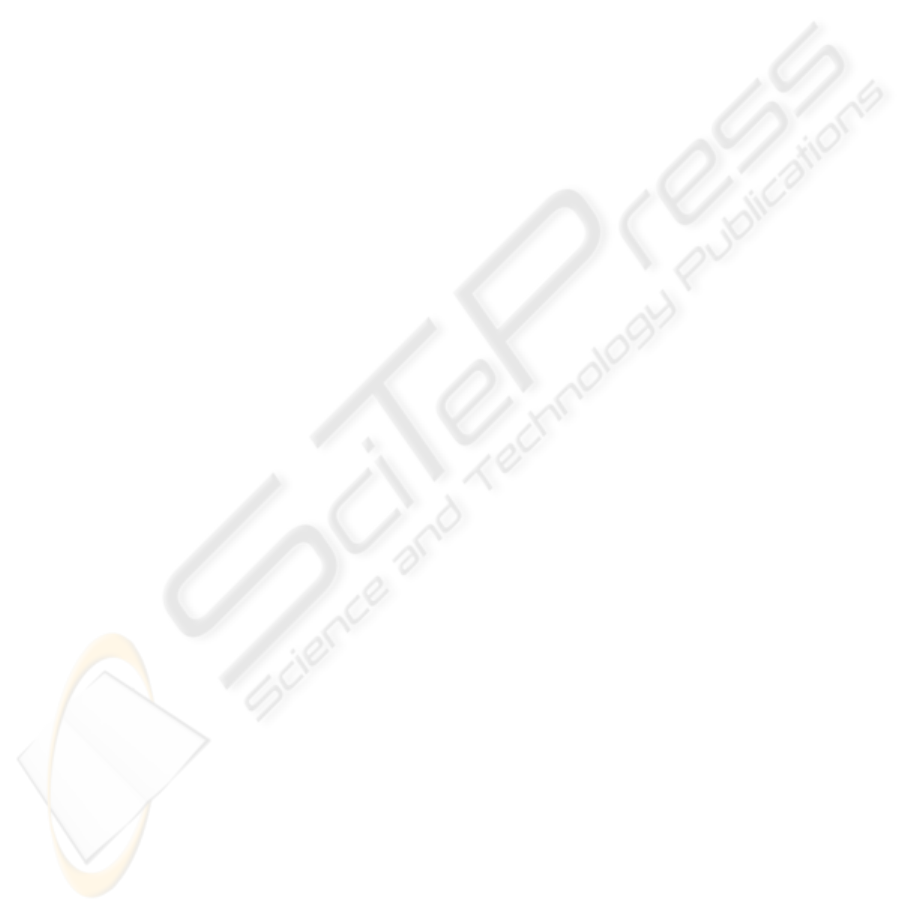
have been tuned experimentally, Figure 1 shows an
example of PSNR and MSSIM values obtained sev-
eral values of p. It is shown that both measures give
the similar optimum values of p. For example, with
α =1.5 the optimum value of p is around 1.3, and
with α =1.9 the optimum value of p is around 1.7.
We show in Figure 2 the results of the adaptive l
p
-
norm filter in suppressing impulsive noise in Lena im-
age. Figure 2(a) shows the image corrupted by im-
pulsive noise which is represented by Symmetrical α-
stable noise (α=0.5). The output of the NLMS filter
is shown is Figure 2(b). Figure 2(c) shows the filtered
image by using the weighted median filter (WMF).
The filtered image using the weighted myriad filter
(WMyF) is shown in Figure 2(d). Figure 2(e) shows
the filtered image using the 2-D adaptive LMP filter
with fixed step-size. Finally, the filtered image by the
normalized l
p
-norm filter is shown in Figure 2(f). The
filter parameters have been tuned to obtain the best vi-
sual results.
The visual quality demonstrates the superiority of
using fractional lower-order statistics filtering algo-
rithms which gives a good performance with edges
and fine image details preserving.
Table 1 summarizes respectively the FSNR and
the MSSIM achieved by the adaptive proposed fil-
ters with different values of α . According to
these results, the filtered image using l
p
-norm fil-
ter has higher FSNR and MSSIM improvement from
the LMP/NLMP linear equalizers than those of the
NLMS filter, weighted median filter and weighted
myriad filter. The measures achieved by the normal-
ized l
p
-norm filter give good improvement in term of
visual quality and signal-to-noise ratio improvement.
When a reference image is not available. We use
the same methodology as described in (Kotropoulos
and Pitas, 2001). We have tested the robustness of
the filter coefficients that are determined at the end
of a training session and are applied to filter a noisy
image that has been produced by corrupting a differ-
ent reference image than the one used in the training
session. In Figure 3, we present a filtered house im-
age using the coefficients determined at the end of a
training session on lenna image. The same noise pa-
rameters have been used during the training session.
When, regarding the quality of the filtered images and
the quality evaluation measures, we can say that the
proposed filter is very good for α-stable noise perfor-
mance measurement.
5 CONCLUSION
In this paper, we presented a 2-D adaptive l
p
-norm
filter for noise suppression in images. Experimental
results on natural images showed marked improve-
ment in visual and numerical qualities when using the
normalized l
p
-norm algorithm adaptation. The filter
is very suitable for α-stable and impulsive noise re-
moval.
REFERENCES
G. Aydin, O. Arikan, and E. Cetin. Robust adaptive filter-
ing algorithms for α-stable random processes. IEEE
Trans. On Circuits and Systems II, vol. 46, N. 2, pp.
198-202, February 1999.
M. Shao, C. L. Nikias. Signal processing with fractionnal
lower order moments : Stable processes and their ap-
plications. Proc. IEEE, vol. 81, pp. 986-1009, 1993.
J. G. Gonzalez, G. R. Arce. Weighted myriad filters :
A robust filtering framework derived from alpha-
stable distributions. Proc. of the IEEE Int. Conf. on
Acoustics, Speech and Signal Processing, Atlanta,
GA, May 1996, Vol. 5, pp. 2833-2836.
J. G. Gonzales, G.R. Arce. Zero-order statistics : a signal
processing framework for very impulsive processes.
Proceedings of the IEEE Signal Processing Workshop
on Higher-Order Statistics, Banff, Canada, July 1997,
pp. 254-258.
P. Kidmose. Adaptive filtering for non-Gaussian noise
processes. Proceedings of International Confer-
ence on Acoustics, Speech and Signal Processing,
ICASSP2000, pp. 424-427.
Ercan E. Kuruoglu, Peter J. W. Rayner, William J. Fitzger-
ald. Least l
p
-norm impulsive noise cancellation with
polynomial filters. Signal Processing. v. 69, pp. 1-14.
1998.
A. Ben Hamza and H. Krim. Image denoising : A nonlin-
ear robust statistical approach. IEEE Trans. on Signal
Processing, Vol. 49, N0. 12, Decembre 2001.
Ercan E. Kuruoglu. Nonlinear least lp-norm filters for non-
linear autoregressive α-stable processes. Digital Sig-
nal Processing 12, 119-142 (2002).
O. Tanrikulu and A. G. Constantindes, ”Least-mean Kur-
tosis : A novel higher-order statistics based adaptive
filtering algorithm”, Electronics Letters, vol. 30, no.
3, pp. 189-190, February 1994.
C. Kotropoulos and I. Pitas, Nonlinear Model-Based Im-
age/Video Processing and Analysis, J. Wiley, 2001.
A. H. Money, J. F. Affleck-Graves, G.D.I. Barr, The linear
regression model: L-p-norm estimation and the choice
of p, Communications in Statist. Simulation Comput.
11 (1) (1982) 89-109.
Zhou Wang, Alan C. Bovik, Hamid R. Sheikh and Eero
P. Simoncelli. Image quality assessment: from er-
ror measurement to structural similarity. To appear in
IEEE transactions on image processing, vol. 13, No.
1, January 2004.
VISAPP 2006 - IMAGE FORMATION AND PROCESSING
160