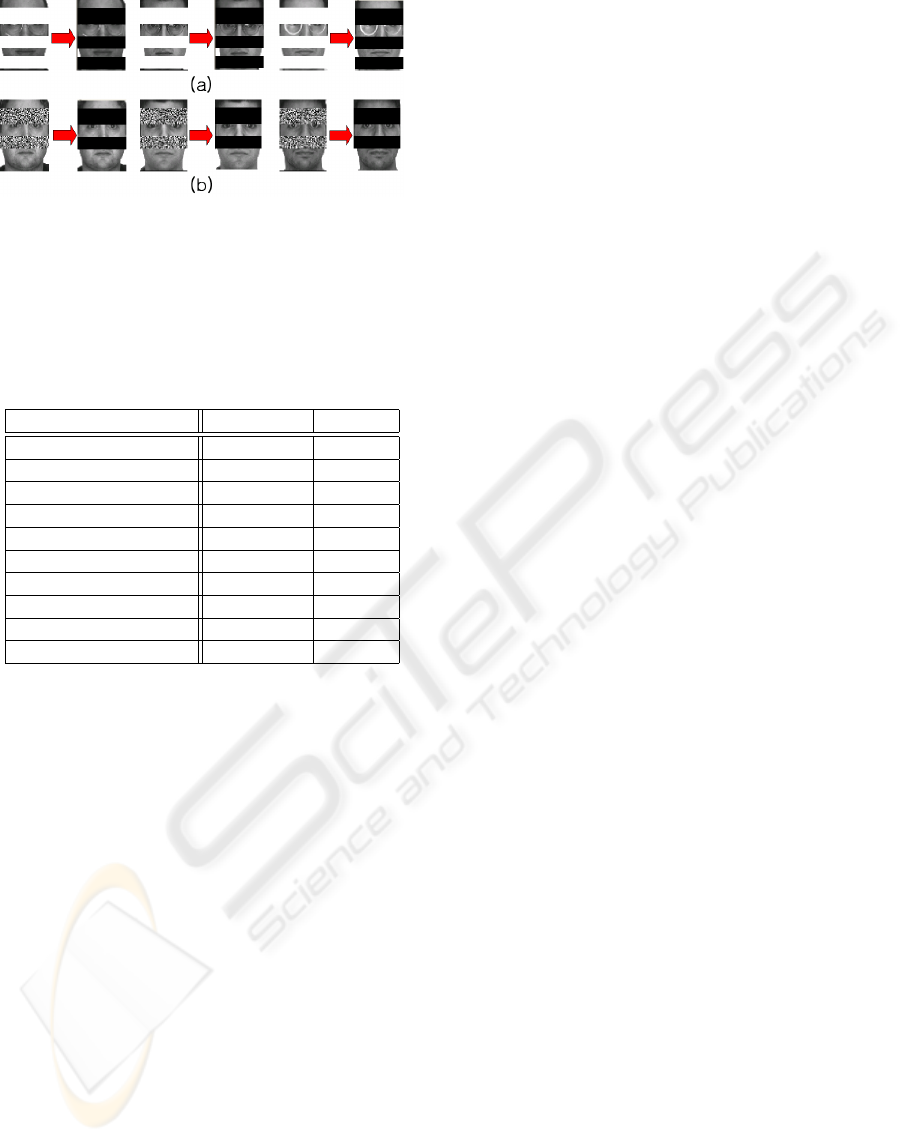
Figure 10: Classifier test to virtual occlusions.
Table 1: The recognition rate under occlusion on the AR
face database : Proposed method (a) occlusion detection by
row, (b) occlusion detection by 6 regions, and (c) occlusion
detection by row after image transformation as shown in
Fig. 4 (b).
Detection Method Sunglasses Scarfs
Proposed Method (a) 98.00% 99.00%
Proposed Method (b) 96.00% 98.00%
Proposed Method (c) 98.00% 98.00%
1-NN 43.18% 20.45%
PCA 43.18% 20.45%
NMF 25.00% 2.27%
LNMF 43.18% 13.64%
AMM 80.00% 82.00%
LEM 68.18% 63.64%
Face-ARG 73.48% 87.88%
ACKNOWLEDGEMENTS
This work has been supported in part by the 3DRC
(3D Display Research Center) under the ITRC (In-
formation Technology Research Center) program of
Korean government.
REFERENCES
Zhao, W. Y., Chellappa, R., Rosenfeld, A. and Phillips, P.
J. (2000). Face Recognition : A Literature Survey. In
UMD CfAR Technical Report CAR-TR-948.
Gao, Y. and Leung, M. K. H. (2002). Face Recognition Us-
ing Line Edge Map. In IEEE Trans. Pattern Analysis
and Machine Intelligence, vol.24, no.6, pp.764-779.
Park, B. G., Lee, K. M. and Lee, S. U. (2005). A Novel
Face Recognition Technique Using Face-ARG Match-
ing. In IEEE Trans. Pattern Analysis and Machine In-
telligence, vol 27, no. 12, pp.1982-1988.
Turk, M., Pentland, A. (1991). Eigenfaces for Recognition.
In Journalof Cognitive Neuroscience, vol.3, pp.71-86.
Belhumeur, P. N., Hepanha, J. P. and Kriegman, D. J.
(1997). Eigenfaces vs. Fisherfaces : Recognition Us-
ing Class Specific Linear Projection. In IEEE Trans.
Pattern Analysis and Machine Intelligence, vol.19,
no.7, pp.711-720.
Georghiades, A. S., Belhumeur, P. N. and Kriegman, D. J.
(2001). From Few to Many : Illumination Cone Mod-
els for Face Recognition under Variable Lighting and
Pose. In IEEE Trans. Pattern Analysis and Machine
Intelligence, vol.23, no.6, pp.643-660.
Saito, Y., Kenmochi, Y. and Kotani, K. (1999). Estimation
of eyeglassless facial images using principal compo-
nent analysis. In IEEE International Conference on
Image Processing, vol.4, pp.192-201.
Hwang, B. W. and Lee, S. W. (2003). Reconstruction of
partially damaged face images based on a morphable
model. In IEEE Trans. Pattern Analysis and Machine
Intelligence, vol.25, no.3, pp.365-372.
Leonardis, A. and Bischof, H. (1996). Dealing with Oc-
clusions in the Eigenspace Approach. Proceedings
of IEEE Conference on Computer Vision and Pattern
Recognition.
Black, M. and Jepson, A. (1998). Eigentracking : Robust
matching and tracking of articulated objects using a
view-based representation. In International Journal
of Computer Vision, vol.26, no.1, pp.63-84.
Yang, J., Zhang, D., Frangi, A. F. and Yang, J. Y.
(2004). Two-Dimensional PCA:ANewApproach to
Appearance-Based Face Representation and Recogni-
tion. In IEEE Trans. Pattern Analysis and Machine
Intelligence, vol.26, no.1.
Gose, E., Johnsonbaugh, R. and S. Jost (1996). The
Book. Pattern Recognition and Image Analysis, Pren-
tice Hall.
Yang, J., Yang, J. Y. (2002). From Image Vector to Ma-
trix : A Straightforward Image Projection Technique-
IMPCA vs. PCA. In Pattern Recognition, vol.35, no.9,
pp.1997-1999.
Ridder, D., Tax, D. M. J. and Duin, R. P. W. (1998). An
Experimental Comparison of One-Class Classification
Methods. In Proceedings of the Fourth Annual Con-
ference of the Advanced School for Computing and
Imaging, Delft.
Martinez, A. M. and Benavente, R. (1998). The AR Face
Database. In CVC Technical Report, no.24.
Lee, D. D. and Seung, H. S. (1999), Learning the parts of
objects by non-negative matrix factorization. In Na-
ture, vol.401, pp.788-791.
Li, S. Z., Hou, X. W., Zhang, H. J. and Cheng, Q. S. (2001).
Learning spatially localized, part-based representa-
tion. In Proceedings of IEEE Conference on Computer
Vision and Pattern Recognition, pp.207-212.
Martinez, A. M. (2002). Recognizing Imprecisely Lo-
calized, Partially Occluded, and Expression Variant
Faces from a Single Sample per Class. In IEEE Trans.
Pattern Analysis and Machine Intelligence, vol.24,
no.6, pp.748-763.
OCCLUSION INVARIANT FACE RECOGNITION USING TWO-DIMENSIONAL PCA
61