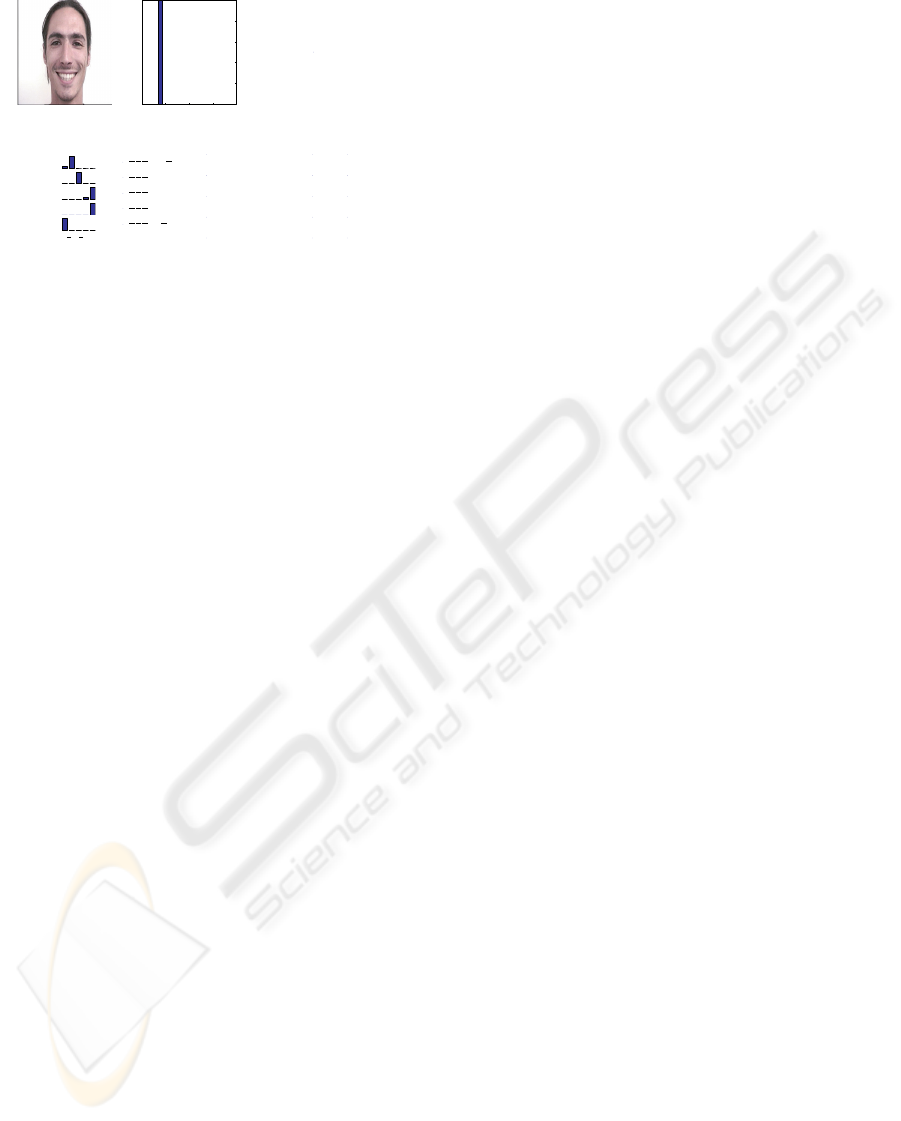
Expressive face 44
1 2 3
0
0.2
0.4
0.6
0.8
1
/Joy
PE
Temporal Classification
/Joy (100%)
Distances states
Dist States and PE
D1D1D1D1D1D1D1D1D1D1D1D1D1D1D1D1D1D1D1D1D1D1D1D1D1D1D1D1D1D1D1D1D1D1D1D1D1D1D1D1D1D1D1D1
>SC
D2D2D2D2D2D2D2D2D2D2D2D2D2D2D2D2D2D2D2D2D2D2D2D2D2D2D2D2D2D2D2D2D2D2D2D2D2D2D2D2D2D2D2D2
>S
D3D3D3D3D3D3D3D3D3D3D3D3D3D3D3D3D3D3D3D3D3D3D3D3D3D3D3D3D3D3D3D3D3D3D3D3D3D3D3D3D3D3D3D3
>C+
D4D4D4D4D4D4D4D4D4D4D4D4D4D4D4D4D4D4D4D4D4D4D4D4D4D4D4D4D4D4D4D4D4D4D4D4D4D4D4D4D4D4D4D4
>C+
D5D5D5D5D5D5D5D5D5D5D5D5D5D5D5D5D5D5D5D5D5D5D5D5D5D5D5D5D5D5D5D5D5D5D5D5D5D5D5D5D5D5D5D5
>C
C SC SSC+C+SC SSC+C+
Features states
Slackened eyebrows 100%
Open mouth 100%
Mouth corners pulled up 100%
Frame/Frame
Figure 4: Classification results and facial features behav-
iors analysis on frame 44 being part of a Joy sequence.
permanent facial features behavior (eyes, eyebrows
and lips), one of the main goals is to be able to han-
dle the Unknown expressions corresponding to all the
facial deformations which do not match to any of the
studied expressions (in this case even a human ob-
server cannot decide between one of them). Hence in
this case the analysis of the individual facial features
behaviors is particularly informative because it pro-
vides a description of these deformations. This analy-
sis is based on the study of the eyes, the mouth and the
eyebrows state. Then the states of the characteristic
distances are recovered. Each characteristic distance
state is translated into behavior of the corresponding
facial features associated with its piece of evidence
(see Figure 4). To provide the most accurate possible
information, only the facial feature behaviors whose
state is singleton (i.e S, C
+
or C
−
) are analysed. The
doubt states are not used. The set of recognized facial
features deformations rules are:
Eyes analysis: Slackened eyes (D
1
== S), Open eyes
(D
1
== C
+
), Eyes slightly closed (D
1
== C
−
),
Eyebrows analysis: Slackened eyebrows (D
2
== S),
Raised inner eyebrows (D
2
== C
+
), Lowered inner eye-
brows (D
2
== C
−
),
Mouth analysis: Closed mouth (D
4
== S), Open mouth
(D
4
== C
+
), Mouth corners pulled downwards (D
5
==
C
+
), Mouth corners pulled up (D
5
== C
−
).
Figure 4 presents an example of the information dis-
played during the analysis of a facial expression se-
quence and of the corresponding facial features be-
haviors. This example shows the results on the frame
44 from one Smile sequence composed of 120 frames.
It corresponds to the apex of the expression. The in-
terface is divided into five different regions: on top
left, the current frame to be analysed; on top mid-
dle the result of the TBM classification on this frame
(here this is a Joy expression with a piece of evidence
equal to 100%); on top right, the results of the tem-
poral classification which corresponds to the classifi-
cation of the sequence since the beginning until the
current frame (here Joy sequence); on bottom left,
the current states of the characteristic distances and
their pieces of evidence; on bottom right, the results
of the analysis of the facial features behaviors with
their pieces of evidence.
7.2 Frame-by-Frame Classification
The aim of this section is to discuss the improvement
of the frame-by-frame classification induced by the
introduction of the smoothing as well as the tempo-
ral information (conditional pieces of evidence). The
analysis of the frame-by-frame classification on the
facial expression sequences shows that the mean im-
provement of the results according to our previous
works are 6.9%. However, the most interesting re-
sults are observed on the sensitivity of the method to
transitory states when the facial feature behavior does
not correspond to neither the Neutral state nor one
apex expression. Indeed in the studied sequences the
subject begins at neutral state, then reaches the apex
of the studied expression and finally comes back to
the neutral state. Before and after the apex, the sub-
ject exhibits some transitory facial expressions. In
these cases, even a human observer cannot classify
them or hesitates between different expressions. Our
system exhibits a similar behavior as can be seen in
Figure3 where top right frame and bottom left frame
correspond to transitory states between the Neutral
and Joy expression. However this temporal combi-
nation is only based on the information contained in
the previous frame. This is not sufficient to recognize
the asynchronous temporal behavior of the facial fea-
tures during a facial expression sequence. This can
be overcome by a dynamic recognition of the facial
expression sequences.
7.3 Classification in Video Sequence
Figure 5 presents an example of a dynamic classi-
fication (based on the characteristic distances evolu-
tion) on a Surprise sequence. The original sequence
has 120 frames where the subject evolves from Neu-
tral, reaches Surprise and comes back to Neutral.We
give selected frames to convey our results in Figure 5
which shows the evolution over time of the facial fea-
tures behaviors and of the facial expressions.
In the first frame the subject is in the Neutral state. At
this time the system cannot give any temporal classi-
fication results. In the second frame we can observe
the sensitivity of the system to recover the behavior
of the facial features. Based on the states of the char-
acteristic distances, the frame-by-frame classification
confidence is 80% that it corresponds to a Surprise
expression and 20%, to a doubt between Surprise and
DYNAMIC FACIAL EXPRESSION UNDERSTANDING BASED ON TEMPORAL MODELLING OF
TRANSFERABLE BELIEF MODEL
99