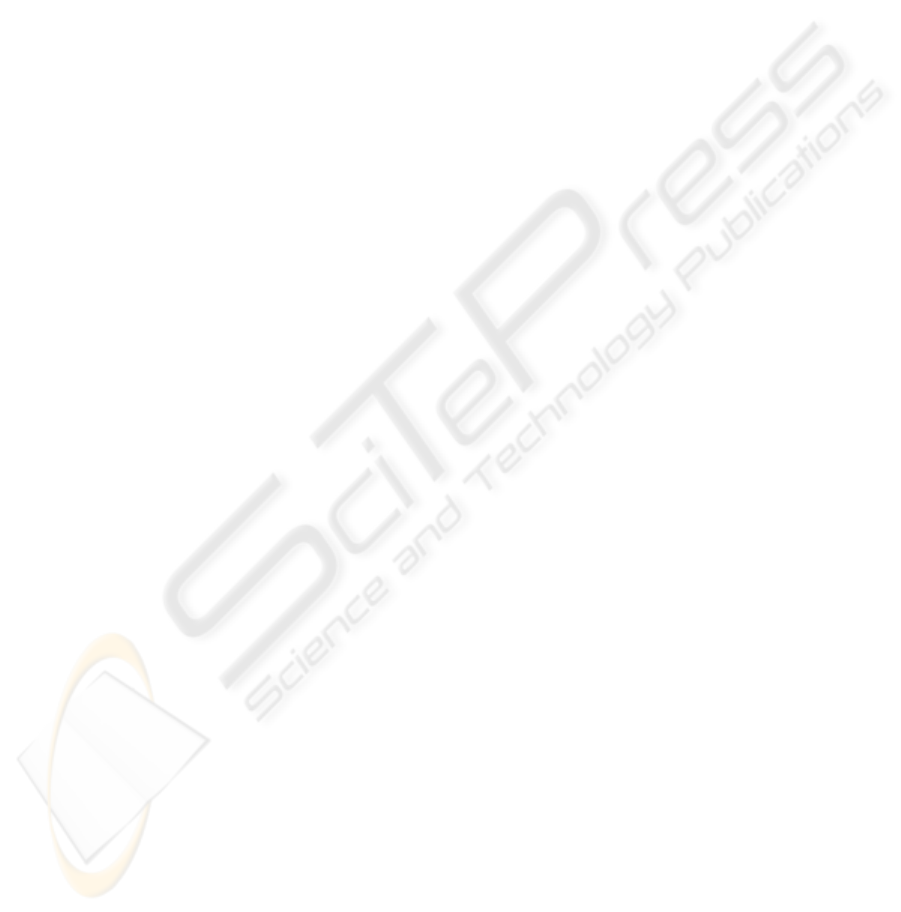
3 DEVELOPMENTS
Many improvement are programmed in our project,
in all phases of its operation.
An improvement is that of using eventual XML
content information for the search (Bellini Nesi
2001, Haus Longari 2002) and that of using text
information from the URL by means of techniques
from Natural Language Processing, to be added to
the content information obtained by the signal: the
context of the sound file, description, annotations
and similar may in fact add useful information on it.
Other features to be used as means for
classification and search will be added, from the
large number identified by the literature (Peeters,
Rodet 2002); an example is the kind of thumbnails
recently introduced by one of the authors
(Evangelista & Cavaliere 2005).
A second modality of search will also be
implemented, based on histogram similarity using
the Kullback-Leibler divergence or other measure.
In this case the user will provide an example file or
an entire class of files for the search; files are then
searched for, which provide best fit to the statistical
distribution of the parameters in the example file.
We are also working to an improvement of the
program, consisting in a parallel version of it;
parallelism will be achieved by a master computer
which will divide the burden of annotation in chunks
and will send tasks to slave computers (these mostly
are in the LAN, but also might reside in any position
in the network); these slaves, as soon as the user in
them decides to open to parallel processing, will
signal its presence in the net and will be waiting for
the completion of the task. The maarester in fact will
receive the address of the slaves which are ready
and will send to it a specific task. The granularity of
these tasks is easily identified in the analysis of the
different sound files: the master just sends the
address of the files in the Internet: the slave will
download the sound file and, in turn, send back the
computed sound parameters to be stored in the
archive for further search.
The practice of our project has collected its first
encouraging results, showing that it has configured a
complete set of tools, which, installed in a Local
Area Network, in a studio or also classroom or
Research Laboratory, allows easily the efficient
paradigm of a parallel archive with distributed
storage and also distributed processing.
Also we realized that in spite of the use of high
level interpreted languages the efficiency of the
program is quite satisfying, while easiness of
prototyping lets experiment easily new solutions: on
the other end a compiled version of the Sound
Browser speeds up both search and classification.
REFERENCES
Bellini, P. Nesi, P., 2001 WEDELMUSIC format: an
XML music notation format for emerging applications
Proceedings of the First International Conference on
Web Delivering of Music.
Burred JJ, A Lerch 2004 Hierarchical Automatic Audio
Signal Classification Journal of the Audio Engineering
Society. Vol. 52, No. 7/8.
Evangelista G., Cavaliere S. 2005. Event Synchronous
Wavelet transform approach to the extraction of
Musical Thumbnails, Proc. of the DAFX05
International Conference on Digital Audio Effects
Madrid, Spain.
Foote, J. 1999. An overview of audio information
retrieval. ACM Multimedia Systems, 7:2–10.
Haus G, Longari M, 2002 Towards a Symbolic/Time-
Based Music language based on XML
Proc. First International IEEE Conference on Musical
Applications Using XML (MAX2002), New York.
Lu L., Hao J., and HongJiang Z., 2001. A robust audio
classification and segmentation method. In Proc. ACM
Multimedia, Ottawa, Canada.
Pachet F, La Burthe A, Zils A, Aucouturier JJ - Popular
music access: The Sony music browser Journal of the
American Society for Information Science and and
Technology, Volume 55, Issue 12 , Pages 1037 – 1044.
Panagiotakis C, Tziritas G, 2005. A Speech/Music
Discriminator Based on RMS and Zero-Crossings -
IEEE Transactions on Multimedia.
Peeters G., Rodet X., 2002. Automatically selecting signal
descriptors for sound classification. In Proceedings of
ICMC 2002, Goteborg, Sweden.
Rossignol S., Rodet X., 1998. et al. Features extraction
and temporal segmentation of acoustic signals. In
Proc. Int. Computer Music Conf. ICMC, pages 199–
202. ICMA.
Scheirer E., Slaney M., 1997. Construction and evaluation
of a robust multifeature speech/music discriminator. In
Proc. Int. Conf. on Acoustics, Speech and Signal
Processing ICASSP, pages 1331–1334. IEEE.
Tzanetakis, G. Cook, P., 2000, MARSYAS: a framework
for audio analysis . Organised Sound,
CambridgeUnivPress 4(3), pages 169-177.
Tzanetakis G. and Cook P., 2002. Musical Genre
Classification of Audio Signals IEEE Transactions on
Speech and Audio Processing, VOL. 10, NO. 5, JULY
p. 293.
Vinet H, Herrera P, Pachet F. , 2002. The Cuidado Project:
New Applications Based on Audio and Music Content
Description Proc. ICMC.
Wold E., Blum T., Keislar D., and Wheaton J., 1996.
Content-based classification, search and retrieval of
audio. IEEE Multimedia, 3(2).
Zhang T. and Kuo J., 2001. Audio Content Analysis for
online Audiovisual Data Segmentation and
Classification IEEE Transactions on Speech and
Audio Processing (4):441–457, May.
Zölzer U. (ed.). 2002. DAFX - Digital Audio Effects. John
Wiley & Sons.
SIGMAP 2006 - INTERNATIONAL CONFERENCE ON SIGNAL PROCESSING AND MULTIMEDIA
APPLICATIONS
338