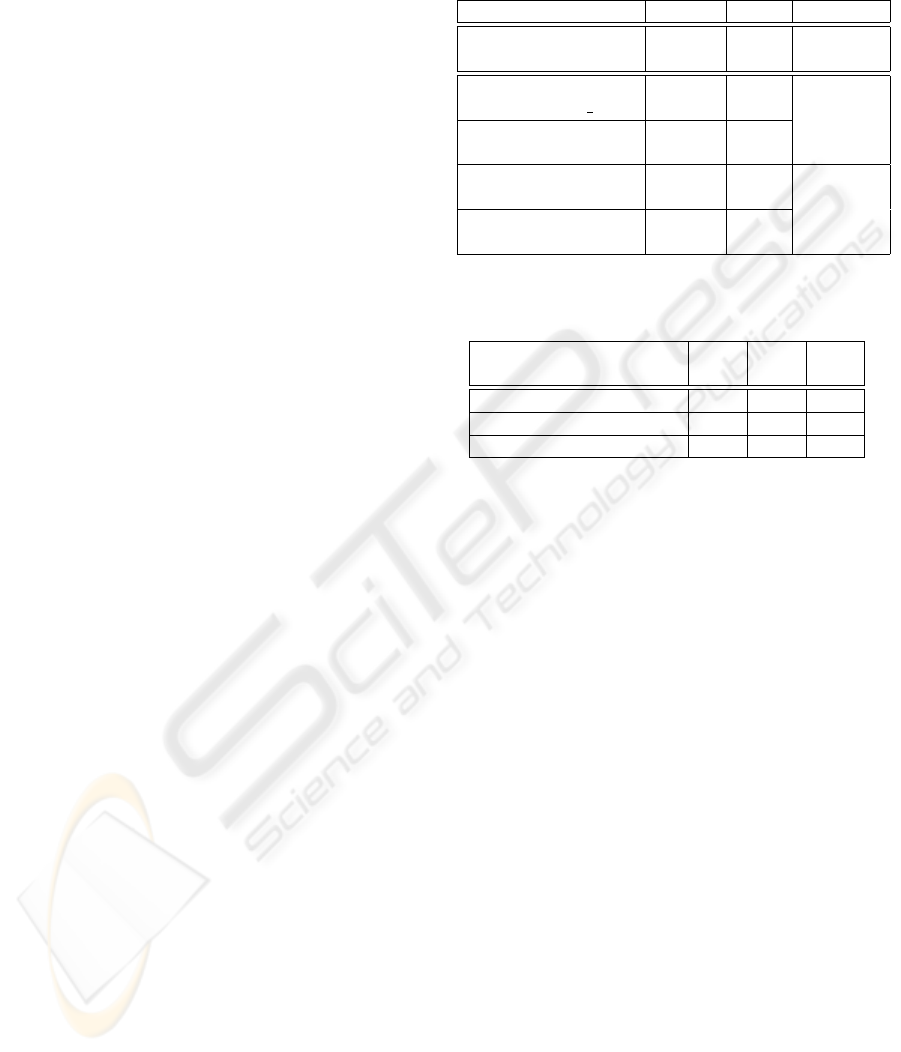
best speech/non-speech discrimination is achieved
with Teager energy and its first derivative and the
best music/non-music one with instantaneous energy.
In the second one, called “fusion B”, we choose
three parameterizations for each discrimination task
(speech/non-speech and music/non-music). Then,
the outputs of these classifiers are merged using the
majority voting strategy.
We assume that these parameterizations are well
performing methods, bring diversity and produce
different kinds of mistakes. Combination of such
experts should reduce overall classification error and
as a consequence emphasize correct outputs.
For every discrimination task, the three parameter-
izations are chosen as follows: we select the best
static feature, the best “dynamic feature” ( static
components plus derivatives) and the best long-term
one. According to our experiments, we obtain:
For speech/non-speech task:
• coif-1 instantaneous energy with 5 bands,
• coif-1 Teager energy with 7 bands with first deriv-
atives,
• variance on 1 second computed on coif-1 Teager
energy with 7 bands.
For music/non-music task:
• coif-1 instantaneous energy with 7 bands,
• coif-1 Teager energy with 7 bands with first deriv-
atives,
• variance on 1 second computed on coif-1 instanta-
neous energy with 7 bands.
Table 5 shows the results of the three discrimination
tasks using both fusion approaches. Besides, Table 5
mentions the error rate obtained by the best classifier
for the global discrimination task (first line). For fu-
sion A, only the global discrimination error rate must
be considered: we can notice a non significant im-
provement. In the other hand, fusion B slightly im-
proves the speech/non-speech and music/non-music
discriminations. Moreover,it provides a significant
decrease of the global classification error rate.
To conclude the experimental part, Table 6 shows
the classification results using the best fusion of clas-
sifiers. Compared to Table 1 (MFCC parameters), a
significant reduction of misclassified segments is ob-
served.
6 CONCLUSION
In this paper, we propose new features based
on wavelet decomposition of the audio signal for
speech/music discrimination.
These features are obtained by computing different
Table 5: Error rates (%) for the 3 discrimination tasks using
the fusion of classifiers.
Param. M/NM S/NS GR
best feature GR
coif-1 7b E+∆
15.0 3.4 17.4
best feature S/NS
coif-1, 7bds, T
E+∆
– 2.7 Fusion A
best feature M/NM
coif-1, 7bds, E
14.5 – 17.0
majority vote with
3 classifiers S/NS
– 2.5 Fusion B
majority vote with
3 classifiers M/NM
14.0 – 16.1
Table 6: Frame distribution (%) for global discrimination
task using the best fusion of classifiers.
`
`
`
`
`
`
`
`
`
`
`
labelled
recognized
S SM M
S 76.9 22.5 0.5
SM 8.9 86.3 4.6
M 0.2 4.1 94.3
energies on wavelet coefficients. Compared to the
MFCC parametrization, the wavelet decomposition
gives a non-uniform time resolution for the different
frequency bands. Moreover, this parameterization is
more robust to signal non-stationarity and allows to
obtain a more compact representation of the signal.
We have tested these new features on a difficult real-
world corpus composed of broadcast programs with
superimposed segments, speech with music or songs
with an effect of “fade in-fade out’.
The new parameterization gives better results than
MFCC-based one for speech/music discrimination.
Best improvements are obtained for the music/non-
music discrimination task, with a relative gain of 40%
in error rate. Moreover, Teager energy feature based
on coif-1 wavelet seems to be a robust feature for
discrimination between speech, music and speech on
music.
Another interesting point is that the proposed para-
meterizations use a reduced number of coefficients to
represent the signal compared to MFCC one.
Finally, the fusion between the classifiers using the
three best speech/non-speech, music/non-music para-
meterizations improves the speech/music discrimina-
tion results. At last, for the speech/music/speech on
music discrimination task, a relative gain of 39% in
error rate is obtained, compared to MFCC parameters.
SPEECH/MUSIC DISCRIMINATION BASED ON WAVELETS FOR BROADCAST PROGRAMS
155