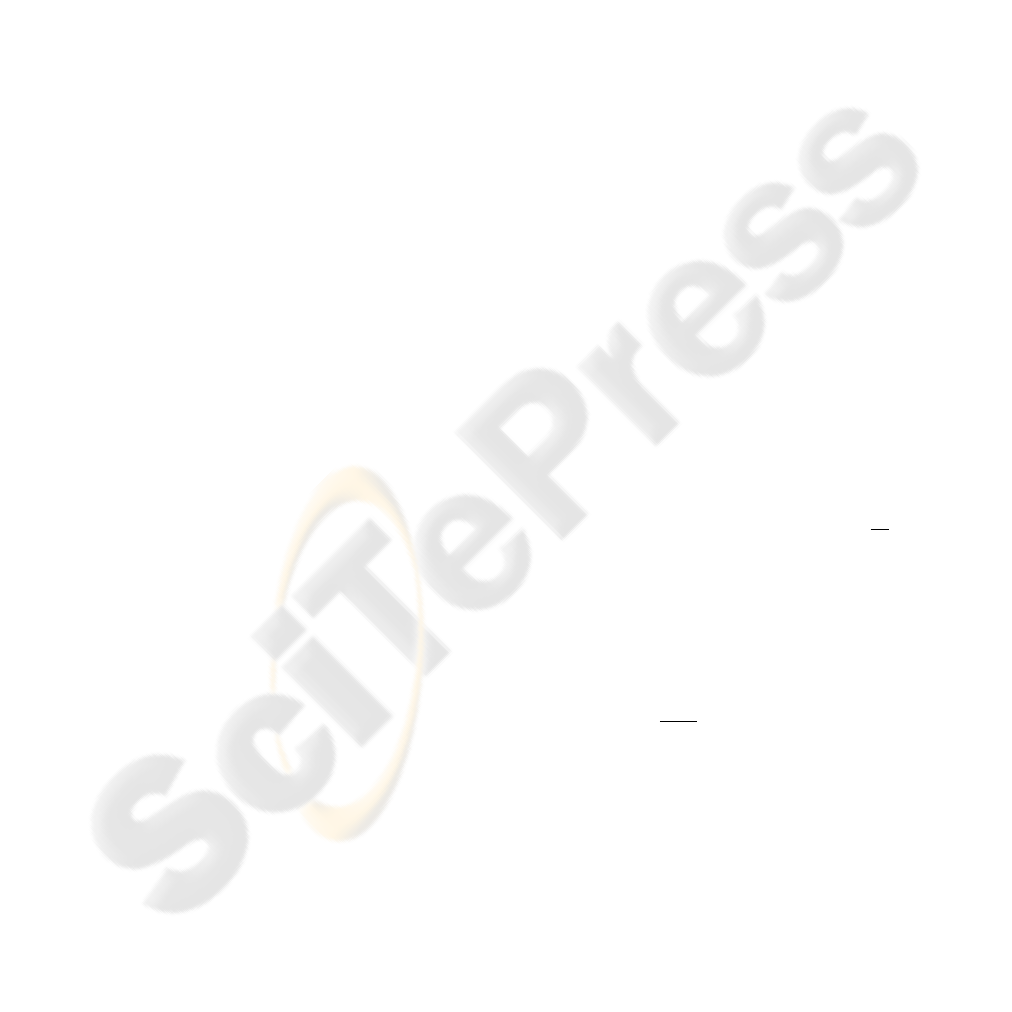
door WLAN channel is commonly statistically mod-
elled, where the modelling approaches concentrate on
the NLOS transmission paths forming numerous and
variously reflected waves (”rich scattering”). To ful-
fill these assumptions the LOS component must be
distinctly attenuated by shadowing effects or when as-
suming high reflection factors ξ
2
≈ 1 the path lengths
of the reflected waves must be in the range of the LOS
path length. In this case the propagation loss of direct
and reflected signal components range in the same or-
der of magnitude and hence the reflection’s impact
rises. Usually those assumptions are less valid for in
- room scenarios than for outdoor transmission with
large distances between Tx and Rx. Therefore a sim-
ilar modelling strategy is regularly performed in mo-
bile communications. When in addition narrow trans-
mission bandwidths are presumed the channel can be
treated flat and information is provided only on the
magnitude level distribution which especially in the
case of high mobility can be modelled by a Rayleigh
process (Paetzold, 2002). In this case the entries of
the channel transfer matrix are assumed to be uncor-
related, zero mean, i.i.d. complex Gaussian random
variables, a case which describes a convenient trans-
mission channel for MIMO applications as the ex-
pected value of its capacity is high (Telatar, 1995) as
long as the receive signal correlation is kept low. Ac-
cording to (Jakes, 1974) the latter condition can be
achieved by antenna spacings of at least about half -
wavelength (λ/2) what therefore has become a com-
mon design issue for MIMO antenna arrays. When
the direct LOS signal component gets present this fact
can be incorporated leading to the well known Rice
propagation model (Paetzold, 2002). This statistical
approach is widely used when discussing early indoor
transmission systems and it is included in the popu-
lar, cluster based channel model introduced by Saleh
(Saleh and Valenzuela, 1987).
Contrarily, in the case of the in - room propagation
scenario discussed in the sequel a dominant LOS sig-
nal component that carries most of the energy is al-
ways present which in the consequence leads to cor-
related signals at the receiver inputs. Of course also in
indoor scenarios the LOS signal component ist some-
times obstructed and therefore diffracted by objects
but it is known from the theory of diffraction that no
significant losses arise as long as the obstructing ob-
stacle’s size stays below the first Fresnel zone’s di-
mension. For a typical WLAN center frequency of 2.4
GHz and distances of 2 m and 3 m between the obsta-
cle and the Tx and Rx, respectively, the size of the first
Fresnel zone is about 80 cm in diameter which is large
compared to typical furniture or persons. Besides, one
set of antennas (Tx or Rx) is typically mounted at the
ceiling which distinctly reduces the risk of obstruc-
tion. In these scenarios the simple approach of a λ/2
antenna spacing is no longer sufficient as it theoret-
ically can not exploit the maximum MIMO capacity
with respect to LOS. By geometrically optimizing the
antennas’ positions and spacings at Tx as well as Rx
resulting in proper phase angle relations among the
entries of the channel transfer matrix, it is possible to
make accessible the maximum MIMO capacity even
in the case of correlated channels. Coinciding it is
inevitable that the LOS signal component never ex-
ists without reflections which can hardly be included
in any strategy of constructing high - rank channel
transfer matrices. Obviously this effect gets more em-
inent when the power contributed by reflections rises
as it is the case for declining room dimensions. Be-
fore we answer the question to what extent the high
- rank LOS channel construction enhances the capac-
ity in such real - world scenarios the theoretic back-
ground in terms of channel modelling and capacity
calculation is summarized.
Capacity calculation: Contingent upon the consid-
ered transmission bandwidth our measurements cover
the case of nearly frequency flat channels. Neverthe-
less, a frequency selective channel model should be
assumed because it also includes the frequency flat
channel as a special case and it is necessary for our
way of evaluating the measured results as it will be ex-
plained in the sequel. According to our presumptions
mentioned above we consider the following, widely
deterministic description of the MIMO transmission
channel. For a single input - single output (SISO) fre-
quency selective, deterministic transmission channel
the equivalent baseband channel transfer function for
the time invariant case is given by
H(f) =
K−1
X
k=0
a
k
e
−j2πf τ
k
=
K−1
X
k=0
a
k
e
−j2π
L
k
λ
, (1)
where the sum is evaluated over the K different trans-
mission paths consisting of K − 1 reflected signal
parts and the LOS signal component is incorporated
by the index k = 0. The complex amplitude factor
a
k
includes the phase information φ, the path - loss
as well as the power - loss due to the reflection fac-
tor ξ
2
, i.e. a
k
=
ξλ
4π L
k
e
jφ
. The time τ
k
denotes the
signal part’s delay for the particular transmission path
which is clearly linked to its path length L
k
by the
speed of light c
0
, i.e. τ
k
= L
k
/c
0
. The right part of
the equation additionally introduces H(f ) depending
on the wavelength λ. For a non - mobile scenario a
k
and τ
k
are mainly constituted by the location’s geom-
etry, i.e. primarily by the Tx’s and Rx’s positioning
within the room as well as their arrangement in rela-
tion to the main reflection layers like walls, bottom,
ceiling or large scale objects. With increasing mobil-
ity it is more and more appropriate for the channel
to be modelled by some statistical process. In the ob-
served scenarios described in this paper the amount of
WINSYS 2006 - INTERNATIONAL CONFERENCE ON WIRELESS INFORMATION NETWORKS AND SYSTEMS
188