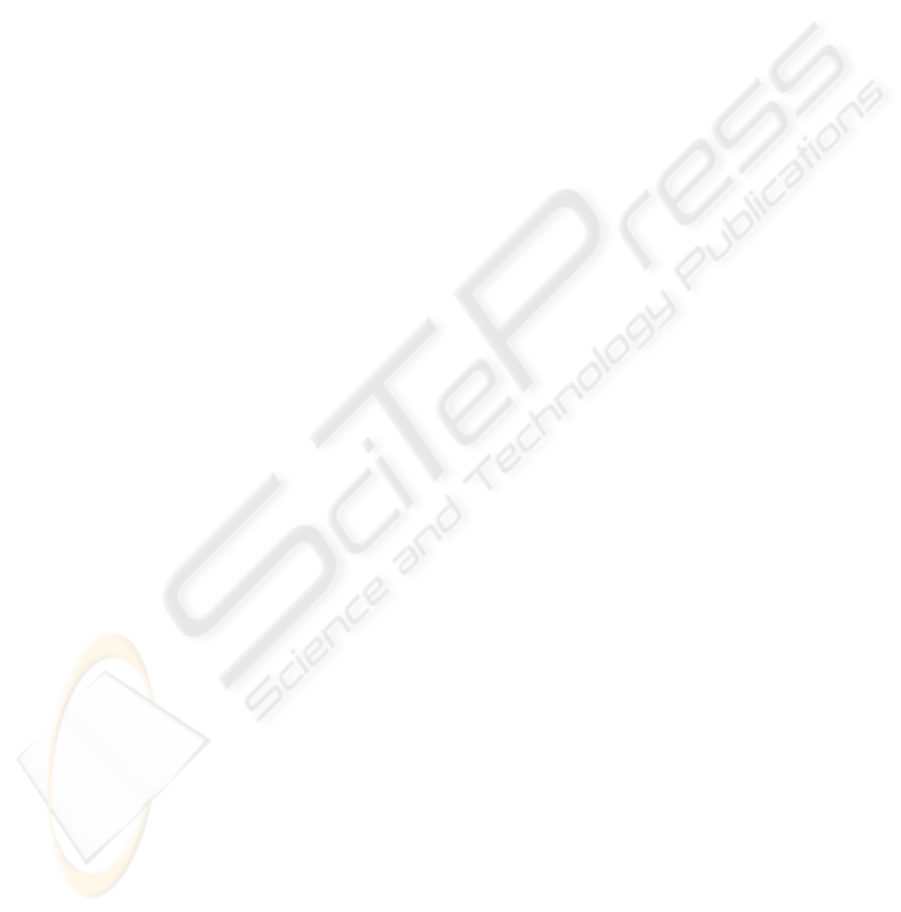
4.2 Customer Service and Customer
Management
CRM has built a bridge for enterprise and customers,
and becomes more efficient and rapid by data
mining. With the right care and service, customers
will be high-value, and their profitability will be
optimized. After mining the customer’s hobbies and
interests, managers can improve business process.
This will reduce the cost, and raise the benefit.
Based on the information of customers, data mining
can help segment the prospective customers, seek
high profitable ones. Furthermore, it can create a
solution to solve the problems, such as which
customers are mostly to churn? The marketing tools
merged with data mining have a mighty ability to
predict the market, and business managers can
implement the marketing campaign (Groth R, 1999).
Companies can evaluate the marketing message to
be delivered to customers and identify high-value
ones. With personalized services, the relationship
between company and customers is kept tightly.
4.3 Prediction and Marketing
Forecast and exploit the market is a crucial matter in
improving economic benefit. CRM system can
provide online service for customers, and the
enterprise may quickly process the feedback about
product function and selling service, and catch the
information about interaction record, transaction
history and request. After that, marketing and
serving automatically will be come true, so the
quality and efficiency of the actions has been
improved. Web mining is helpful for identifying the
profitable customers, improving the respond rate and
reducing the cost. We can plan and predict the
marketing campaigns after mining the customer
data, implement positive selling and cross-selling,
then provide the manifold and intelligent service. So
that the business process has been expand and the
quality and efficiency of the actions has been
improved.
4.4 Credit Evaluation and Fraud
Detection
Based on the transaction records, we can score the
customer’s credit rate, and then carry out selling and
preferential service, enhance the abilities of market
responding. In insurance, managers can analyze the
action and feature of customers through building the
evaluation model of customer. Then, they survey the
instance and detect fraud, to discover who claims for
compensation with perjury and predict a potentially
fraudulent transaction. In telecommunication, data
mining can predict and track cellular fraud for
telecommunications, this control and reduces the
unexpected risk effectively.
5 FUTURE RESEARCH
CRM is the core parts of current company, how to
manage the customer relationship becomes a key of
business competition. In order to take the lead of
economic society, the company should make full use
of the information technologies, and utilize its
vantages and features. Data warehouse and data
mining have mighty abilities to analyze the
information, this provide a strong science evidence
and technological support. The integrated CRM
system will play an important role in commercial
activity; and we believe that it will have a widely
development market and application prospect.
ACKNOWLEDGEMENTS
The work in this paper was supported by National
Natural Science Foundation of China (60175022,
40571128, and 40572166)
.
REFERENCES
Adam R. (2001). CRM Shifts to Data Mining to Keep
Customers, Global Finance, 15 (11), 97-98.
Alex B, Smith,K(2000). Building data mining applications
for CRM. McGraw-Hill Companies,Inc.
Allan R. (2002). Integrating ERP Can Overcome CRM
Limits J, Software Magazine, 22 (1), 29-32.
Gabrielle Gagnon. (1999). Data Warehousing: An
Overview, PC Magazine, 18 (5), 245-246.
Gottgtroy M, Gottgtroy P. (2003). Ontologies, CRM, data
mining: How to integrate? Management Information
Systems, 7, 307-316.
Groth R. (1999). Data Mining: Building competitive
advantage,Prentice-Hall Ptr.
Lariviere Bart, Van Den poel Dirk. (2005). Predicting
customer retention and profitability by using random
forests and regression forests techniques, Expert
Systems with Applications, 29(2): 472-484.
Lawyer J, Chowdhury S. (2004). Best practices in Data
Warehousing to support business initiatives and needs.
Proc. of the 37th Annual Hawaii International
Conference on System Sciences, 3499-3507.
Nordine Melab. (2001). Data mining: A key contribution
to E-business. Information & Communications
Technology Law, 10(3), 309-318.
Ron K, Foster P. (2001). Applications of Data Mining to
Electronic Commerce, Data Mining and Knowledge
Discovery, 5(1/2), 1-7.
ICEIS 2006 - ARTIFICIAL INTELLIGENCE AND DECISION SUPPORT SYSTEMS
290