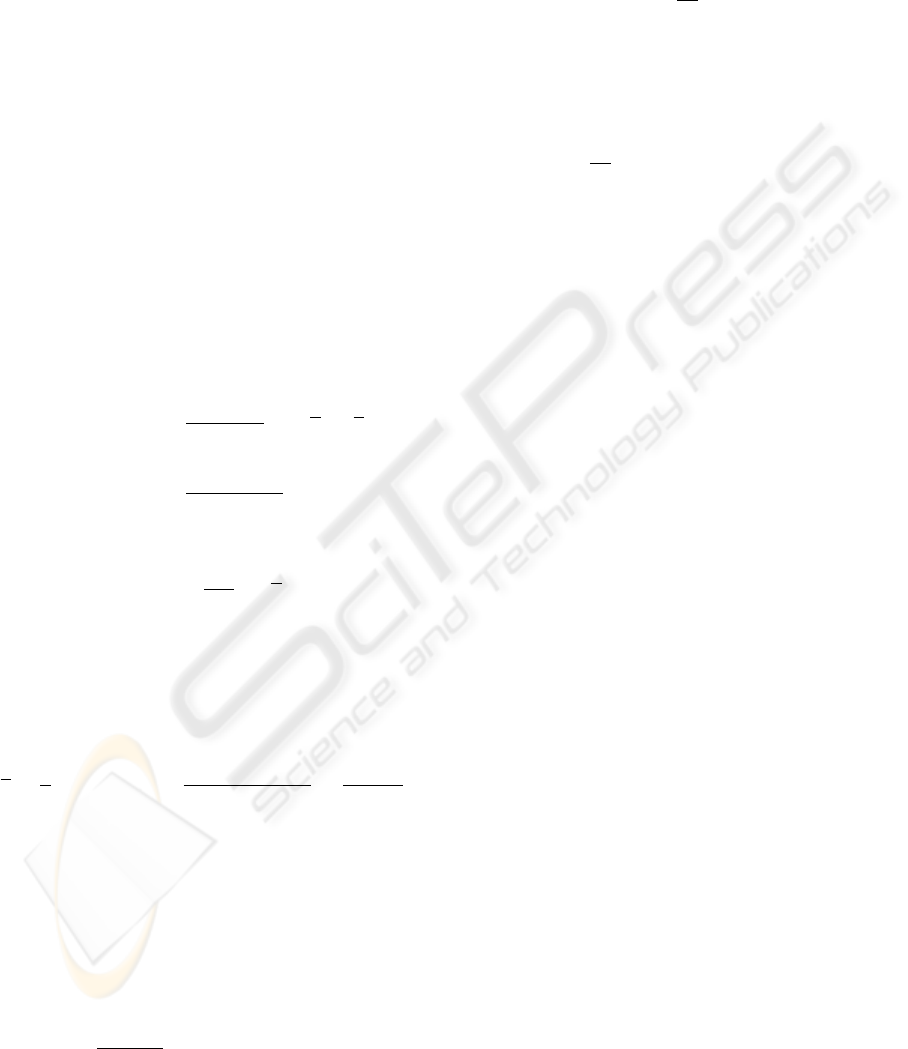
by the fluctuations in the period of the voltage. The
situation was depicted in (Vig, 2001) and (de la Rosa
et al., 2005).
The short-term stability measures most frequently
found on oscillator specification sheets is the two-
sample deviation, also called Allan deviation, σ
2
y
(τ)
(Howe et al., 1999), (Vig, 2001).
Classical variance in non-stationary noise
processes doesn’t converge to concrete values.
It diverges for some noise processes (de la Rosa
et al., 2005). This is the reason whereby non-classical
statistics are used to characterize short term instabil-
ity. AVAR and MVAR have proven their adequacy
in characterizing frequency phase and instabilities.
These easy-to-compute variances converge for all
noise processes observed in precision frequency
sources, have a straightforward relationship to power
law spectral density of noise processes, and are faster
and more accurate than the FFT (Lesage and Ayi,
1984).
The estimates of AVAR and MVAR for a given cali-
bration time τ for a m-data series of phase differences,
x, are given by equations 2 and 3, (Greenhall, 1988):
AV AR ≡ σ
2
y
(τ,m)=
1
2(m − 1)
m
j=2
y
j
− y
j−1
2
=
1
2τ
2
(m − 1)
m
j=2
∆
2
τ
x(jτ)
2
(2)
MVAR ≡
1
2τ
2
∆
2
τ
x
2
, (3)
where the bar over x denotes the average in the
time interval τ (averaging time), and ∆
2
τ
x = x
i+2
−
2x
i+1
+ x
i
, is the so called second difference of
x. The fractional frequency deviation is the relative
phase difference in an interval τ. It is defined by equa-
tion 4:
y =
1
τ
t
t−τ
y(s)ds =
x(t) − x(t − τ)
τ
=
∆
τ
x(t)
τ
.
(4)
Non-classical statistics estimators, defined above, in
equations 2 and 3, for non-stationary series charac-
terization, give an average dispersion of the fractional
frequency deviation due to the noise processes cou-
pled to the oscillator. As a consequence time do-
main instability (two-sample variance) is related to
the noise spectral density via (Rutman and Walls,
1991):
σ
2
y
(τ)=
2
(πν
0
τ)
2
f
h
0
S
φ
(f)sin
4
(πfτ)df , (5)
where ν
0
is the carrier frequency and f is the Fourier
frequency (the variable), and f
h
is the band-width of
the measurement system. S
φ
(f) is the spectral den-
sity of phase deviations, which is in turn related to
the spectral density of fractional frequency deviations
by(Rutman and Walls, 1991):
S
φ
(f)=
ν
2
0
f
2
S
y
(f), (6)
The classical power-law noise model is a sum of the
five common spectral densities. The model can be de-
scribed by the one-sided phase spectral density S
φ
(f)
via (IEE, 1988), (Greenhall, 1988):
S
φ
(f)=
ν
2
0
f
2
2
α=−2
h
α
f
α
= ν
2
0
4
β=0
h
β
f
β
, (7)
for 0 ≤ f ≤ f
h
. Where, again, f
h
is the high-
frequency cut-off of the measurement system (the
band-width); h
α
and h
β
are constants which rep-
resent, respectively, the independent characteristic
models of oscillator frequency and phase noise (Al-
lan, 1987), (IEE, 1988), (Greenhall, 1988).
For integer values (the most common case) we have
the following approximate expression:
σ
y
(τ) ∼ τ
µ/2
, (8)
where µ = −α − 1, for −3 ≤ α ≤ 1; and µ ≈−2
for α ≥ 1. In the case of the modified Allan variance,
the time-domain instability can be approximated via:
Modσ
y
(τ) ∼ τ
µ
(9)
Hereinafter we use expressions 8 and 9 for analyzing
noise in these work.
2.2 Time Domain Stability
Characterization Curves
Equations 8 and 9 are used to make the graphical
representation of σ
y
(τ) vs. τ , and lets us infer the
noise processes which causes frequency instability by
means of measuring the slope in a log-log graph (Rut-
man and Walls, 1991). These functional characteris-
tics of the independent processes are widely used in
modelling frequency instability of oscillators. Table
1 shows the experimental criteria adopted in the main
references. In the second column or MVAR we have
picked up two different criteria according to the ref-
erences (Rutman and Walls, 1991) and (Lesage and
Ayi, 1984), respectively. We have kept the notation in
the works (Rutman and Walls, 1991) and (Lesage and
Ayi, 1984) for µ/2 and µ
, respectively.
The five noise processes have been modelled and
VAR and MVAR have been calculated. Hereinafter
we show the simulation results of the time-series and
their associated VAR and MVAR graphs. From this
simulations we adopt the criteria depicted in the sec-
ond column of MVAR in table 1. Figures 1-5 show
ICEIS 2006 - ARTIFICIAL INTELLIGENCE AND DECISION SUPPORT SYSTEMS
190