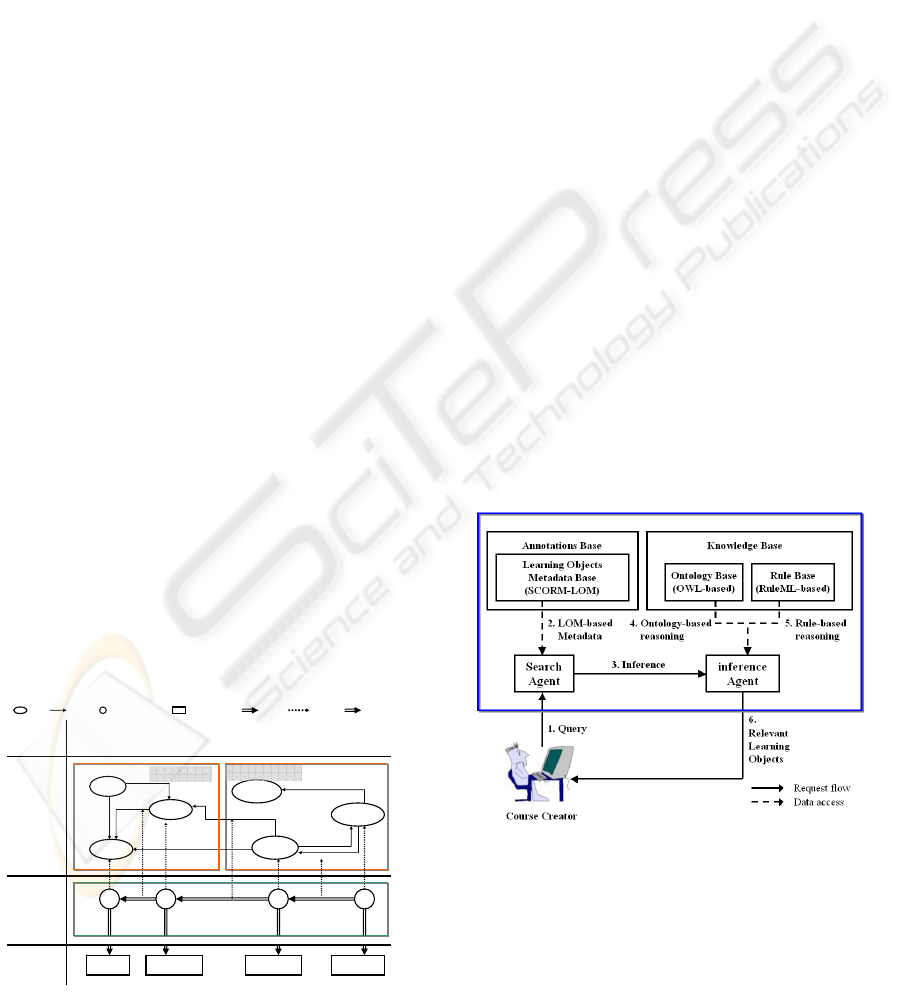
individual learning object, based on what metadata
and knowledge the learning object has shown so far.
Secondly, because the content or category of a
learning object may keep changing, dynamic finding
provides more up-to-date suggestions than a static
design. Thirdly, as the number of learning objects
may be large, adding suggestion links may become
cumbersome for the course developer. Lastly, it can
also be used at run-time to help in the decision of
what content model component to deliver to the
learner.
This paper is organized as follows. The next section
presents the multi-layered semantic framework.
Section 3 gives the architecture of SLOAR. In
Section 4, we illustrate how the SLOAR can employ
Semantic Web technologies to provide different
approaches for finding relevant learning objects.
2 MULTI-LAYERED SEMAMTIC
FRAMEWORK FOR LOM
In this paper, we propose a novel integration
approach to combine the first four layers of
Semantic Web stack, including URI layer (learning
objects), XML layer (Metadata, LOM), Ontology
layer (OWL), and Rule layer (Rule Markup
Language, RuleML), as shown in Figure 1.
The URI layer is composed of learning objects,
which can be identified by URI. The XML layer is
composed of LOM metadata that are XML-based
metadata for describing learning objects. The
Ontology layer provides OWL-based ontologies,
which can enhance LOM to Semantic-based
metadata, hence, improve reasoning capabilities of
LOM. The Rule layer supports more complex
inference than the Ontology layer, and builds
RuleML rules on top of OWL ontologies. The
ontology is based on description logics to provide
sound and decidable reasoning. In contrast, the rule
is a logic program, which can complement ontology
to support more complex rule-based inferences.
ml:XML
ml:HTML
ml:XHTML
Markup Ontology
property
ml:application
cu-1 cu-2
if jm:XMLParser(ml:XML,ml:JAXP) and jm:using(jm:JAXP,jm:DOM)
then jm:treeMode(ml:XML,jm:DOM)
cu-4
Ontology Layer
(OWL-based
ontology base)
Rules Layer
(RuleML)
XML Layer
(SCORM-LOM
classification,
relation
Metadata)
learning object ID relationclass
ml:standard
instance
URI Layer
(Learning
Objects)
JAXP for XML XML Advance
XHTML
Introduction
entity
http://../jaxp.htm http://../xml.htm http://../html.htm
Java DOM
http://../jdom.htm
cu-3
ml:isversionOf
ml:XMLParser
Java Ontology
jm:treeMode
jm:DOM
jm:Java
jm:API
jm:JAXP
jm:API
jm:using
learning object
Figure 1: Multi-layered semantic framework.
3 SYSTEM ARCHITECTURE
The basic function of SLOAR is to provide the
information of relevant learning objects for course
creators. It supports three different approaches for
finding relevant learning objects, including LOM-
based metadata, ontology-based reasoning, and rule-
based inference. In SLOAR, each learning object is
associated with a classification metadata to quote
extra semantic from a specific ontology class and is
associated with a relation metadata to quote extra
semantic from a specific ontology property. These
ontologies are implemented in OWL that can be
integrated into LOM, and as a result, the semantic
capabilities of LOM were greatly improved.
The core components of SLOAR include the
annotation base, knowledge base, search agent, and
inference agent. The flow-oriented SLOAR
architecture is depicted in Figure 2, as described in
the following:
˙ Annotations base: is a learning object
annotations repository that is composed of LOMs. A
LOM is an XML document containing a set of
markup elements to describe the learning objects..
˙ Knowledge base: is developed by the Semantic
Web standard to support reasoning tasks. The
knowledge is grouped into two categories: ontology
layer inference using OWL-based ontologies and
rules layer inference using RuleML logic program.
˙ Search agent: is a search engine that supports for
a XPath query on the learning objects metadata base.
˙ Inference agent: is an intelligent agent that is
implemented based on a JESS-based rule engine
(JESS, 2005) and supports a XSLT processor.
Figure 2: SLOAR Architecture.
The information flow of the SLOAR, as listed in
the Figure 2, can be realized and started as follows.
1. The requester sends a learning object with URL
to the search engine.
2. This step is the LOM-based metadata approach.
The search agent relies on the request to query
the LOM Base to finding all relevant LOM-
ICEIS 2006 - SOFTWARE AGENTS AND INTERNET COMPUTING
182