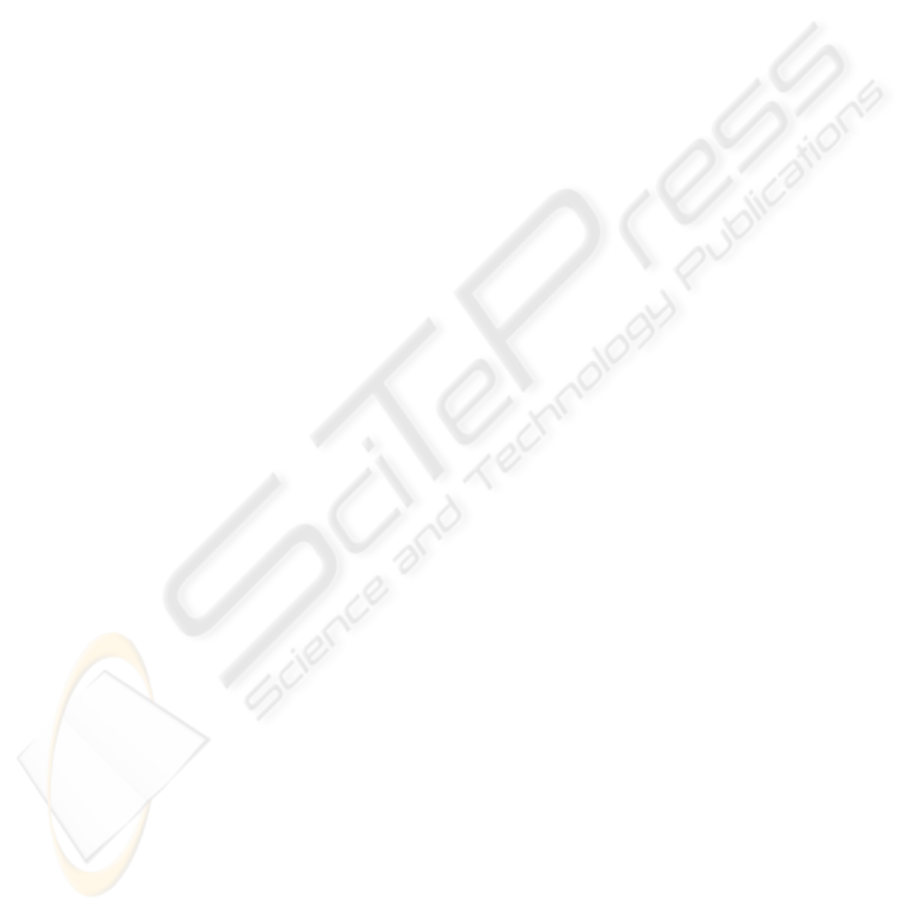
which the application domain is covered by the ontol-
ogy depends significantly on the interviewed experts,
different domain experts might often present different
views. On the other hand, the manual approach has
one big advantage, since it also captures the most spe-
cific concepts that the enterprise actually uses. Also,
the more abstract concepts at the upper level give an
intuitive idea of the scope of the ontology.
Neither of the approaches produce many errors in
the ontology, according to the evaluations, but some
improvements can be made in both methods. Im-
provements of the automatic method could be to eval-
uate the patterns more thoroughly. The patterns could
also be enriched with more axioms and natural lan-
guage descriptions. Improvements of the manual ap-
proach could be to use a larger set of knowledge ac-
quisition methods to elicit more complex structures
from the document sources and domain experts.
The main conclusion, which can be drawn is that
the ontology engineering approaches each have both
strengths and weaknesses and complement each other
well. This might suggest that a combination of the ap-
proaches could give the best results, but it is too early
to state this firmly, since the methods have only been
tested in parallel for one single case. The next step
is to repeat this experiment in other cases in order to
be able to generalise these results and perhaps arrive
at some solution for combining the approaches. Also
the resulting ontologies complement each other quite
well. In this particular project a possible combination
of the two ontologies for generating the application
ontology needed will be investigated.
ACKNOWLEDGEMENTS
This work is part of the research project Semantic
Structuring of Components for Model-basedSoftware
Engineering of Dependable Systems (SEMCO) based
on a grant from the Swedish KK-Foundation (grant
2003/0241). We thank all evaluation teams for con-
tributing to the results of this study. Also special
thanks to three anonymous reviewers for valuable
comments on how to improve this paper.
REFERENCES
Blomqvist, E. (2005). Fully Automatic Construction of
Enterprise Ontologies Using Design Patterns: Initial
Method and First Experiences. In Proc. of The 4th Intl
Conf. on Ontologies, DataBases, and Applications of
Semantics (ODBASE), Cyprus.
Brewster, C., Alani, H., Dasmahapatra, S., and Wilks, Y.
(2004). Data Driven Ontology Evaluation. In Proc.
of Intl Conf. on Language Resources and Evaluation,
Portugal.
Davies, I., Green, P., Milton, S., and Rosemann, M. (2003).
Using Meta Models for the Comparison of Ontolo-
gies. In Proc. of Eval. of Modeling Methods in Sys-
tems Analysis and Design Workshop-EMMSAD’03.
G´omez-P´erez, A. (1999). Evaluation of Taxonomic Knowl-
edge in Ontologies and Knowledge Bases. In Banff
Knowledge Acquisition for Knowledge-Based Sys-
tems, KAW’99, volume 2.
Guarino, N. (1998). Formal Ontology and Information Sys-
tems. In Proceedings of FOIS’98, pages 3–15.
Guarino, N. and Welty, C. (2002). Evaluating Ontological
Decisions with OntoClean. Communications of the
ACM, 45(2):61–65.
Hartmann, J., Spyns, P., Giboin, A., Maynard, D., Cuel, R.,
Suarez-Figueroa, M. C., and Sure, Y. (2005). D1.2.3
methods for ontology evaluation. Version 1.3.1,
Available at: http://knowledgeweb.semanticweb.org/,
Downloaded 2005-05-10.
Lozano-Tello, A. and G´omez-P´erez, A. (2004). ONTO-
METRIC: A Method to Choose the Appropriate On-
tology. Journal of Database Management, 15(2).
McGuinness, D., Fikes, R., Rice, J., and Wilder, S. (2000).
An Environment for Merging and Testing Large On-
tologies. In Proc. of the 7th Intl Conf. on Principles of
Knowledge Representation and Reasoning (KR2000),
Colorado, USA.
Navigli, R., Velardi, P., Cucchiarelli, A., and Neri, F.
(2004). Automatic Ontology Learning: Supporting a
Per-Concept Evaluation by Domain Experts. In Work-
shop on Ontology Learning and Population (ECAI
2004), Valencia, Spain.
Noy, N. F. and Musen, M. A. (2000). PROMPT: Algorithm
and Tool for Automated Ontology Merging and Align-
ment. In 17th National Conf. on AI (AAAI-2000),
Austin, Texas.
¨
Ohgren, A. and Sandkuhl, K. (2005). Towards a Methodol-
ogy for Ontology Development in Small and Medium-
Sized Enterprises. In IADIS Conference on Applied
Computing, Algarve, Portugal.
Porzel, R. and Malaka, R. (2004). A Task-based Approach
for ontology Evaluation. In Workshop on Ontology
Learning and Population (ECAI 2004).
Supekar, K., Patel, C., and Lee, Y. (2004). Character-
izing Quality of Knowledge on Semantic Web. In
Proceedings of AAAI Florida AI Research Symposium
(FLAIRS-2004), Miami Beach, Florida.
van Heijst, G., Schreiber, A. T., and Wielinga, B. J. (1997).
Using explicit ontologies for KBS development. Intl
Journal of Human-Computer Studies, 46(2-3).
Yao, H., Orme, A. M., and Etzkorn, L. (2005). Cohesion
Metrics for Ontology Design and Application. Jour-
nal of Computer Science, 1(1):107–113.
ONTOLOGY CONSTRUCTION IN AN ENTERPRISE CONTEXT: COMPARING AND EVALUATING TWO
APPROACHES
93