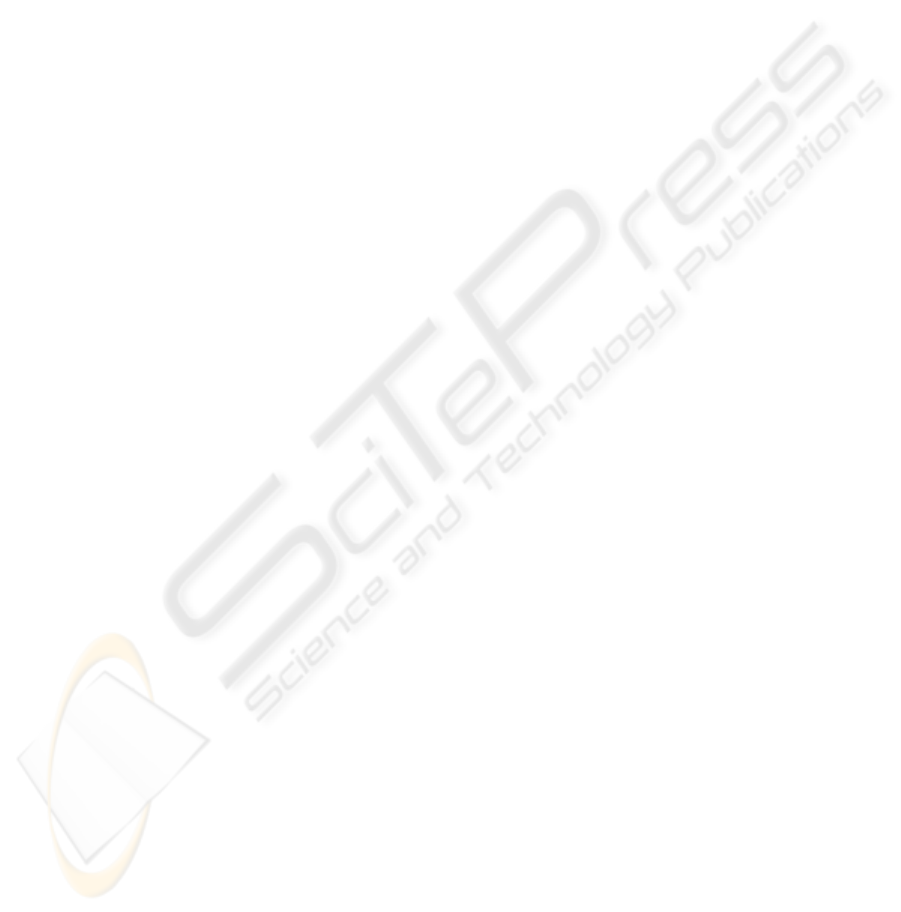
the properties of the spectra in each class. With this method only four binary
classifiers were employed and only two proteins where misclassified. In addition the
system was able to classify correctly all of the proteins belong to either β, or α/β or
“others” class. The experimental determination of additional protein CD spectra is in
progress. This will, hopefully, provide a more balanced training set and so enable
more accurate prediction of protein structures.
References
1. Wallace, B.A. and R.W. Janes, Synchrotron radiation circular dichroism spectroscopy of
proteins: secondary structure, fold recognition and structural genomics. Current Opinion in
Chemical Biology, 2001. 5(5): p. 567-571.
2. Whitford, D., Proteins : structure and function. 2005, Chichester, West Sussex, England ;
Hoboken, NJ: John Wiley & Sons c2005.
3. Fasman Gerald, D., Circular dichroism and the conformational analysis of biomolecules.
1996, New York ; London: Plenum Press.
4. Manavalan, P. and W.C. Johnson, Sensitivity of Circular-Dichroism to Protein Tertiary
Structure Class. Nature, 1983. 305(5937): p. 831-832.
5. Venyaminov, S.Y. and K.S. Vassilenko, Determination of Protein Tertiary Structure Class
from Circular Dichroism Spectra. Analytical Biochemistry, 1994. 222(1): p. 176.
6. Scholkopf, B. and A.J. Smola, Learning with kernels : support vector machines,
regularization, optimization, and beyond. Adaptive computation and machine learning.
2002, Cambridge, MA ; London: MIT Press.
7. Branden, C. and J. Tooze, Introduction to protein structure. 1999, New York: Garland
c1999.
8. Murzin, A.G., et al., SCOP: A Structural Classification of Proteins Database for the
Investigation of Sequences and Structures. Journal of Molecular Biology, 1995. 247(4): p.
536.
9. Pearl, F., et al., The CATH Domain Structure Database and related resources Gene3D and
DHS provide comprehensive domain family information for genome analysis. Nucleic
Acids Research, 2005. 33(Supp): p. D247-D251.
10. Broomhead, D.S. and D. Lowe, Multi-Variable Function Interpolation and Adaptive
network. Complex System, 1988: p. 2:321.
11. Bishop, C.M., Neural networks for pattern recognition. 1995, Oxford: Clarendon Press
c1995.
12. Orr, M.J.L., Introduction to Radial Basis Function Networks. 1996, University of
Edinburgh: Edinburgh, Scotland, UK.
13. Orr, M.J.L. Optimising the Widths of RBFs Radial Basis Functions. in Fifth Brazilian
Symposium on Neural Networks. 1998. Belo Horizonte, Brazil.
14. Vapnik, V., The nature of statistical learning theory. 1995, New York ; London: Springer.
15. Shawe-Taylor, J. and N. Cristianini, Kernel methods for pattern analysis. 2004, Cambridge,
UK ; New York: Cambridge University Press.
16. Friedman, J., Another approach to polychotomous classification. Technical report Stanford
University, UA, 1996.
17. Platt, N.C.J. and J. Shawe-Taylor, Large magin dags for multiclass classification. Technical
report, Microsoft Research, Redmond, US, 1999.
18. Chapelle, O., et al., Choosing Multiple Parameters for Support Vector Machines. Machine
Learning, 2001. 46(1/3): p. 131-160.
67