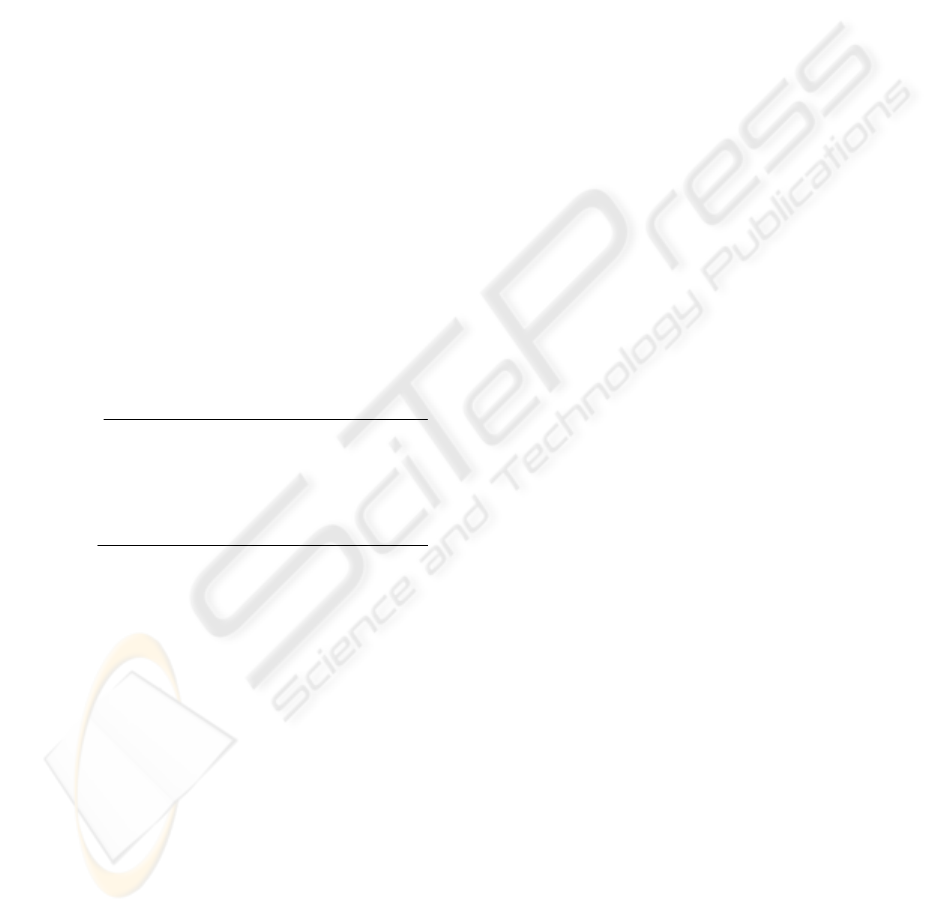
• If the key idea and the witness sentence share
one same class term, add 1 point to the score of
the key idea;
SADIM displays the key ideas corresponding to
each witness sentence on the basis of the already
calculated score. Depending to the user's choice the
system matches each witness sentence to a chosen
key idea.
5 ASSESMENT OF SADIM
The first assessment of SADIM is based on a test
corpus that contains 990 witness sentences (WS) and
390 key ideas (KI). The corpus contains sentences of
two types:
- Type 1: Presence of a Common term between KI
terms and those of WS.
- Type 2: Absence of common terms between terms
of WS and the KI but there can be some semantic
ties between these terms.
In this assessment we determine the recall and
the precision measures that are extensively used in
the domain of information research. We are going to
adapt these measures to our diagnosis method in the
following way:
(Number of KI correctly generated with SADIM to WS/ number of KI
correctly generated with the expert to W
S)
(Number of KI correctly generated with SADIM to WS/ Number of KI
correctly generated with SADIM to WS
)
Results of type 1 sentences
For this type of sentences, the results are as follows:
Recall = 94% Precision = 90%
Results of type 2 sentences
For this type of sentences the results are as follows:
Recall = 64% Precision = 50%
According to the previous results we can identify a
general recall and precision of the order:
General recall = 79% General precision = 70%
6 CONCLUSION
In this paper we started with a presentation of the
concept of diagnosis of the management
engineering, then we gave a brief overview of the
methods of knowledge extraction from the textual
data.
In a latter step we exposed a method of
knowledge extraction that permits to solve
insufficiencies of the SEGESE tool. This method has
led to the emergence of the SADIM system.
We finally made an experimentation of SADIM
in order to give evidence to our method contribution.
As perspectives we intend to spread the
application of our approach into other domains and
to integrate the training and ontological techniques
in SADIM.
REFERENCES
Amarnath, G. and al., 2003. “Towards a formalization of
disease-specific ontologies for neuroinformatics,”
Neural Netwoks, Special issue, No. 16, pp. 1277-1292.
Bourigault, D., 1994. “Terminology extraction software
for the aid of text-based acquisition knewledge,”
Thesis deposited in the school of Higher Studies
Sciences Paris, 1994.
Chevallet, J., 2003. “Language processing for Knowledge
extraction for information research,” Third scientific
days of young research GEI03.
David S., Plante P., 1990. Termino version 1.0. Rapport de
recherche du Centre d'Analyse de Textes
parOrdinateurs, Université du Québec à Montréa
l.
Daille B., 2002. “Mixed approach for terminology
automatic extraction: lexical statistics and linguistic
filters,” Doctorate Thesis, University of Paris VII.
Daille B., Fabre C. and Sebillot P,., 2002. “Applications of
computational Morphology: many morphologies,” P.
BOUCHER, 2002. ISBN 1-57473-125-4.
Jouis, C. 1995. “SEEK, Knowledge acquisition software
using linguistic background without using knowledge
on the external world,” Days concerns acquisition,
validation and training, Grenoble..
Kim, J. and Moldovan D., 1993. “Palka: a system for
lexical knowledge acquisition,” in proceeding of the
second international conference on information and
knowledge management, pages 124-131, Washington,
United States..
Kolsi J. , Belguith L., and Abdelmajid B., 2005.
“Conception and development aid system of
management engineering diagnosis,” Third scientific
days of young research GEI05,.
Oueslati, R., 1999.“Corpus-based knowledge acquisition
aid,” Doctorate Thesis, University Louis
PasteuStrasbourg.
Savall H. and Zardet V., 1989. “Mastering the hidden
coasts and performances: periodically negociable
activity contract,” preface: Marc andré Lanselle,
Edition Economica, edition 2, 351 P.
Savall H. and Zardet V., 2004. “Research in management
science: Qualimetric, seeing complex object,” preface:
BOJE David (USA), Edition Economica, 432p.
∑
∑
=
i
i
i
WSexpert to e with thgenerated correctly KI ofNumber
WS toSADIM with generated correctly KI ofNumber
i
Recall
Precision
∑
∑
=
i
i
i
i
WS toSADIM with generated correctly KI ofNumber
WS toSADIM with generated correctly KI ofNumber
ICEIS 2006 - ARTIFICIAL INTELLIGENCE AND DECISION SUPPORT SYSTEMS
334