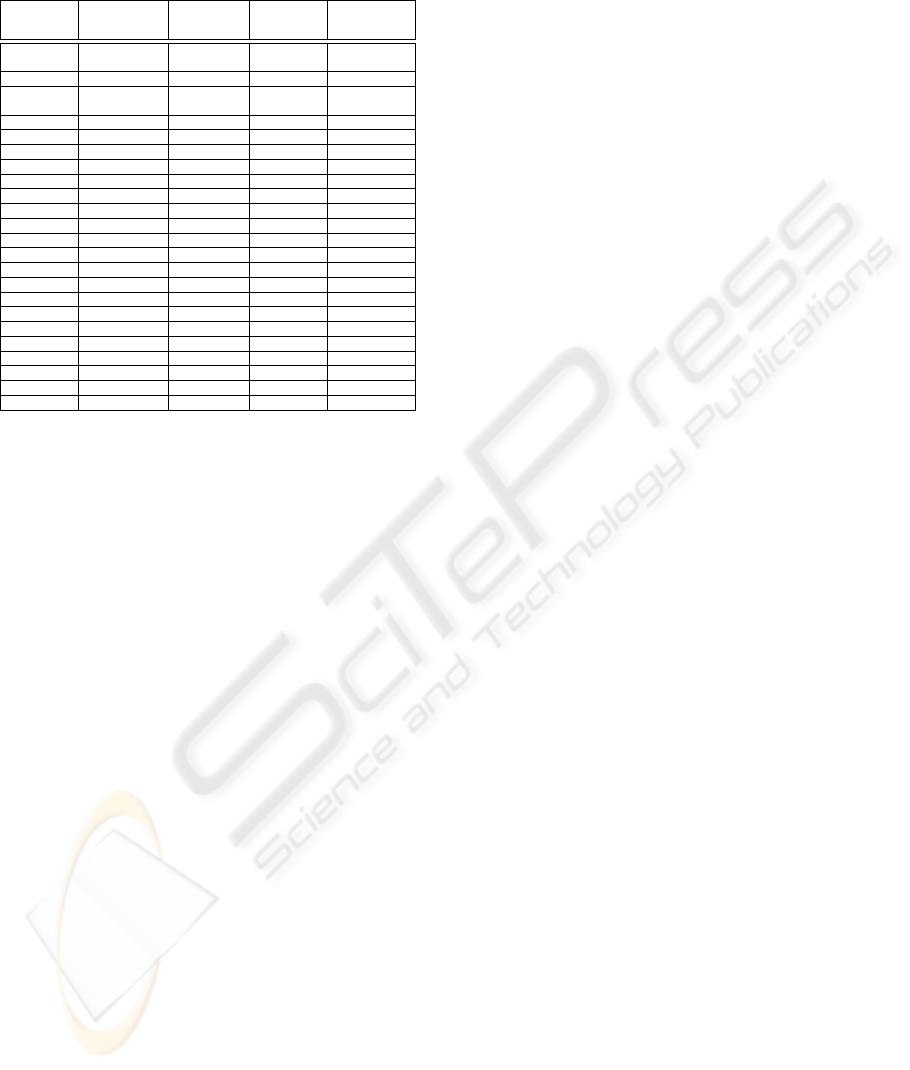
Table 6: Cluster comparison from the relative distance view
(IMs represented by their orders).
R
1
∩ R
1
R
2
∩ R
2
R
1
∩ R
2
R
1
∩ R
2
R
1
∩ R
1
∩
R
2
∩ R
2
0,2,5,10,21 0,2,5,8,10, 0,2,5,10,21 0,2,5,10,21 0,2,5,10,21
21,25,27,29
1,9 1,9
3,19,23, 3
28,30
4 4
6 6,31
7,17 7,17,19,23,28 7,17 7,17 7,17
8,14,18
9,13,22
11,12 11,12,16 11,12 11,12,16 11,12
13
14,18 14,18 14,18 14,18
15 15,34,35
19,23,28,30 19,23,28 19,23,28
20,27
22
24
25,29
26 26
27,29
31
32 32 32 32 32
33 33 33 33 33
34,35 34,35 34,35 34,35
an important method. By calculating the dissimilar-
ity between 36 IMs, we have determined eight stable
clusters of IMs as eight different aspects found from
the two opposite datasets.
The eight stable clusters denote an interesting re-
lations between IMs because they remark the stable
behaviors.
REFERENCES
Agrawal, R., Mannila, H., Srikant, R., Toivonen, H., and
Verkano, A. (1996). Fast discovery of association
rules. In Advances in Knowledge Discovery in Data-
bases. AAAI/MIT Press.
Blanchard, J., Guillet, F., Gras, R., and Briand, H.
(2005a). Assessing rule interestingness with a prob-
abilistic measure of deviation from equilibrium. In
ASMDA’05, Proceedings of the 11th International
Symposium on Applied Stochastic Models and Data
Analysis.
Blanchard, J., Guillet, F., Gras, R., and Briand, H. (2005b).
Using information-theoretic measures to assess asso-
ciation rule interestingness. In ICDM’05, Proceed-
ings of the 5th IEEE International Conference on Data
Mining.
Blanchard, J., Kuntz, P., Guillet, F., and Gras, R. (2003).
Implication intensity: from the basic statistical defin-
ition to the entropic version (Chap. 28). In Statistical
Data Mining and Knowledge Discovery.
Carvalho, D. R., Freitas, A. A., and Ebecken, N. F. F.
(2003). A critical review of rule surprisingness mea-
sures. In Proceedings of Data Mining IV - Interna-
tional Confeference on Data Mining.
Carvalho, D. R., Freitas, A. A., and Ebecken, N. F. F.
(2005). Evaluating the correlation between objective
rule interestingness measures and real human interest.
In PKDD’05, the 9th European Conference on Prin-
ciples and Practice of Knowledge Discovery in Data-
bases.
Choi, D. H., Ahn, B. S., and Kim, S. H. (2005). Priori-
tization of association rules in data mining: Multiple
criteria decision approach. In ESA’05, Expert Sytems
with Applications.
Freitas, A. (1999). On rule interestingness measures. In
Knowledge-Based Systems, 12(5-6). Elsevier.
Gavrilov, M., Anguelov, D., Indyk, P., and Motwani, R.
(2000). Mining the stock market: which measure is
best? In KDD’00, Proceedings of the 6th Interna-
tional Conference on Knowledge Discovery and Data
Mining.
Gras, R., Briand, H., Peter, P., and Philipp
´
e, J. (1996). Im-
plicative statistical analysis. In IFCS’96, Proceedings
of the Fifth Conference of the International Federation
of Classification Societies. Springer-Verlag.
Hilderman, R. and Hamilton, H. (2001). Knowledge Dis-
covery and Measures of Interestingness. Kluwer Aca-
demic Publishers.
Huynh, X.-H., Guillet, F., and Briand, H. (2005). Clustering
interestingness measures with positive correlation. In
ICEIS’05, Proceedings of the 7th International Con-
ference on Enterprise Information Systems.
Kaufman, L. and Rousseeuw, P. (1990). Finding Groups in
Data: An Introduction to Cluster Analysis. Wiley.
Kl
¨
osgen, W. (1996). Explora: a multipattern and multistrat-
egy discovery assistant. In Advances in Knowledge
Discovery and Data Mining. AAAI/MIT Press.
Major, J. and Magano, J. (1995). Selecting among rules
induced from a hurricane database. In Journal of In-
telligent Information Systems 4(1).
Newman, D., Hettich, S., Blake, C., and Merz, C. (1998).
[UCI] Repository of machine learning databases,
http://www.ics.uci.edu/∼mlearn/MLRepository.html.
University of California, Irvine, Dept. of Information
and Computer Sciences.
Piatetsky-Shapiro, G. (1991). Discovery, analysis and pre-
sentation of strong rules. In Knowledge Discovery in
Databases. MIT Press.
Ross, S. (1987). Introduction to probability and statistics
for engineers and scientists. Wiley.
Tan, P.-N., Kumar, V., and Srivastava, J. (2004). Selecting
the right objective measure for association analysis. In
Information Systems 29(4). Elsevier.
DISCOVERING THE STABLE CLUSTERS BETWEEN INTERESTINGNESS MEASURES
201