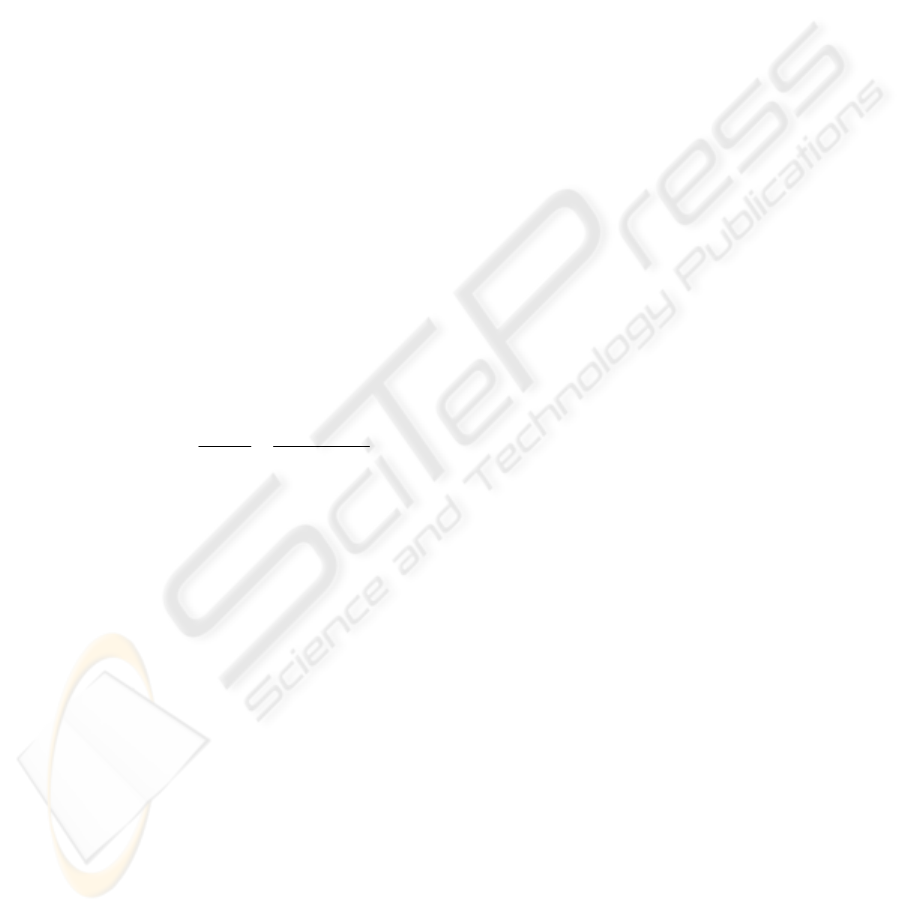
similarity parameter.
However, in fact it is almost impossible to judge whether the two processes
mentioned above are completely identical or not. Because the strict standard requests
that the two processes possess the completely same distribution function, which
means not only the arithmetic mean and variance, but also all the higher order
moments are identical. Thus a relative weakened standard is usually adopted to make
an approximately approached judgment to above equality. That is just checking
whether the average mean and variance, such as first moment and second moment, of
the equation (1) are equal or not.
Fig.1 shows the self-similarity of time series.
(a) A time series with self-similar is shown on the two windows with different
time-scale n
1
and n
2
.
(b) Amplify the smaller window with time-scale n
2
according to time-scales n
1
.
Let’s pay an attention to the two figures in (a) and (b).When the different amplified
multiple M
x
and M
y
are adopted respectively in X axis and Y axis, the fluctuation
curves are so close.
(c) In the possible distribution function P(y) for the two variables y
respectively from windows in (a). Here s
1
and s
2
shows the standard deviation of the
two distribution functions respectively.
(d) The log-log relationship between s and n which is the window size.
The parameter α in equation (1) needs to be abstracted from a given time series.
And the following is the calculation formula.
α=lnM
y
/
ln M
x
(2)
The following equation (3) will be created when put the two parameters M
x
=n
2
/n
1
,
M
y
=s
2
/s
1
into the corresponding position in the equation (2)
(3)
In order to analyze the self-similarity characteristic of given time series, the
following steps should be done.
1) Divide any given observation window into many same size and independent
subsets. To achieve the more reliable value, the average of s in the all subsets
should be calculated.
2) Repeat the above process continually. Then draw these s-n couples on the log-
log plot to estimate the self-similarity parameter α.
The method described above can be no more than applied to certain non-
stationary time series, especially to these with slow movement trend. This means not
all non-stable time series can be well handled with this method. Concerning the self-
similarity parameter, which is also named the Hurst parameter, of most of non-
stationary time series can be precisely estimated through Whittle Estimator method,
which is the practical application of maximal possibility method[4][5]. Whittle
Estimator can provide the confidence interval of the Hurst parameter.
Take the FGN (fractional Gaussian Noise) for example to show the application
of Whittle Estimator in the Hurst parameter estimation. If the data derive from the
FGN procession, the estimated value of Hurst can be gained through minimizing the
value of function Q (H). In the following equation (4), the H is equal to α
mentioned above.
12
12
lnln
lnln
ln
ln
nn
ss
M
M
x
y
−
−
==
α
133