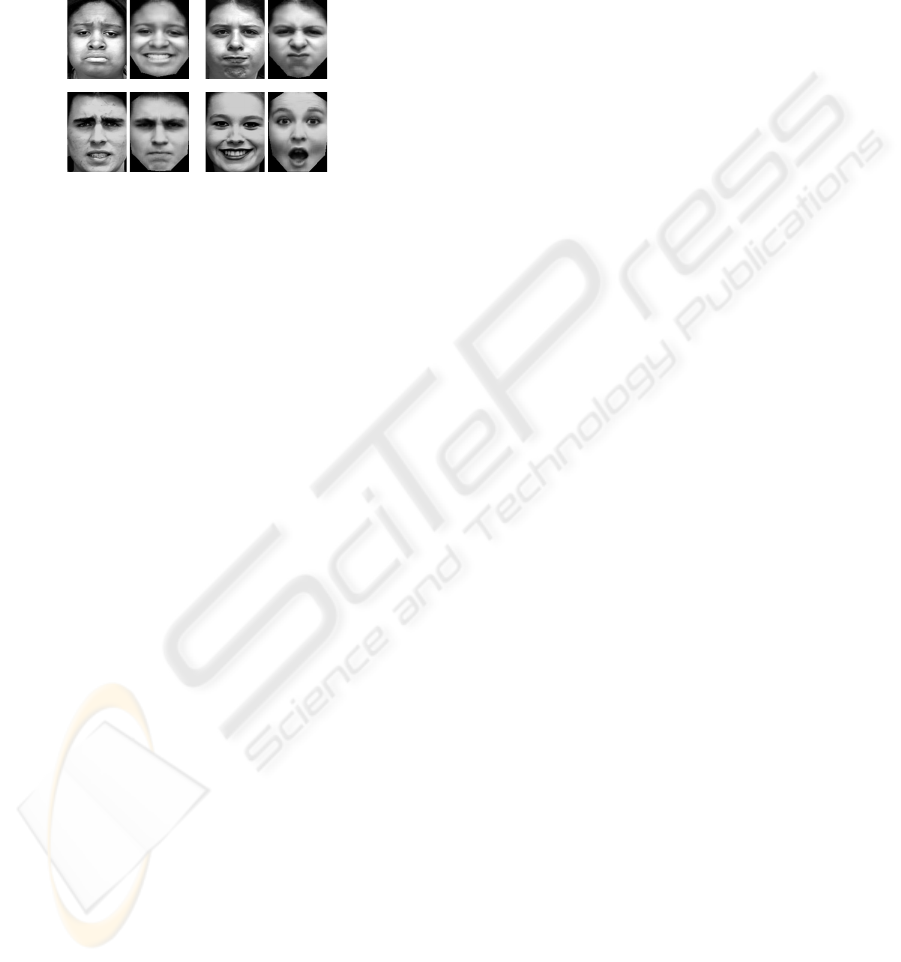
Figure 9 exhibits the capability of the proposed
method to synthesize different expressions with
diverse input-output modes. The input face image
contains arbitrary expression with unknown intensity
for a new person, and the output image is for any
target expression with any target intensity. The
experimental results further prove the effectiveness
of the unified framework of the proposed algorithm.
(a1) (a2) (b1) (b2)
(c1) (c2) (d1) (d2)
Figure 9: Synthesis results of arbitrary input-output pairs.
(a1)(b1)(c1)(d1): input faces with sadness, anger, fear, and
happiness respectively; (a2)(b2)(c2)(d2): synthesized
expressions of happiness, disgust, anger, and surprise.
6 CONCLUSION
In this paper, a novel facial expression synthesis and
recognition scheme is proposed under a general
framework. With intensity alignment, automatic
facial expression recognition and intensity
identification are performed by using Supervised
Locality Preserving Projections (SLPP), and facial
expression synthesis is implemented based on local
geometry preserving. Extensive experiments on the
Cohn-Kanade database illustrate the effectiveness of
the proposed method.
Future work may address the following aspects.
The first extension is to create an objective
evaluation of the facial expression synthesis. A
Gradient Mean Square Error (GMSE) is introduced
(Wang, 2003) to evaluate the synthesized face
image, however, the criteria is not in accord with the
subjective human observation, and will be failed if
the real expression image is not available. Another
focus is to explore more efficient appearance
features, which can deal with the illumination and
pose variations, for creating the generalized
expression manifold. And then synthesis of mixed
expressions needs to be considered so that any
natural expressions can be generated rather than only
creating a few basic expressions. Due to the inter-
dependence among basic expressions, the current
framework might need to be extended by dividing
the face into several relative-independent
subregions, consequently the reconstructions in each
subregion can be performed by the current approach
without changes, and spatial combinations of the
subregions will produce mixed effects of any
possible expressions.
REFERENCES
Roweis, S.T., Saul, L.K., 2000, Nonlinear Dimensionality
Reduction by Locally Linear Embedding, Science,
290, 2323-2326.
He, X., Niyogi, P., 2003, Locality Preserving Projections,
NIPS.
Ridder, D., et al., 2003, Supervised locally linear
embedding, Proc. of Artificial Neural Networks and
Neural Information Processing, ICANN/ICONIP.
Cheng, J., et al., 2005, Supervised kernel locality
preserving projections for face recognition,
Neurocomputing 67, 443-449.
Shan, C., et al., 2005, Appearance Manifold of Facial
Expression, ICCV workshop on HCI.
Hu, C., et al., 2004, Manifold based analysis of facial
expression, CVPRW on Face Processing in Video.
Chang, Y., et al., 2003, Manifold of Facial Expression,
Int. Workshop on AMFG.
Wang, H., Ahuja, N., 2003, Facial expression
decomposition, ICCV.
Tian, Y. , et al., 2001, Recognizing Action Units for Facial
Expression Analysis, IEEE Trans. on PAMI, 23, 97-
115.
Chandrasiri, N.P., et al., 2004, Interactive Analysis and
Synthesis of Facial Expressions based on Personal
Facial Expression Space, FGR.
Pantic, M., 2000, Automatic Analysis of Facial
Expressions: The State of the Art, IEEE Trans. on
PAMI, 22, 1424-1445.
Yeasin, M., et al., 2006, Recognition of Facial
Expressions and Measurement of Levels of Interest
From Video, IEEE Trans. on Multimedia, 8, 500-508.
Zhang, Q., et al., 2006, Geometry-Driven Photorealistic
Facial Expression Synthesis, IEEE Trans. on
Visualization and Computer Graphics, 12, 48-60.
Du, Y., Lin, X., 2002, Mapping Emotional Status to Facial
Expressions, ICPR.
Liu, Q., et al., 2005, A nonlinear approach for face sketch
synthesis and recognition, CVPR.
Tang, X., Wang, X., 2003, Face sketch synthesis and
recognition, ICCV.
Kanade, T.,
et al., 2000, Comprehensive Database for
Facial Expression Analysis, FGR.
Shan, C., et al., 2006, A Comprehensive Empirical Study
on Linear Subspace Methods for Facial Expression
Analysis, CVPRW.
Kouzani, A.Z., 1999, Facial Expression Synthesis, ICIP.
SIGMAP 2007 - International Conference on Signal Processing and Multimedia Applications
52