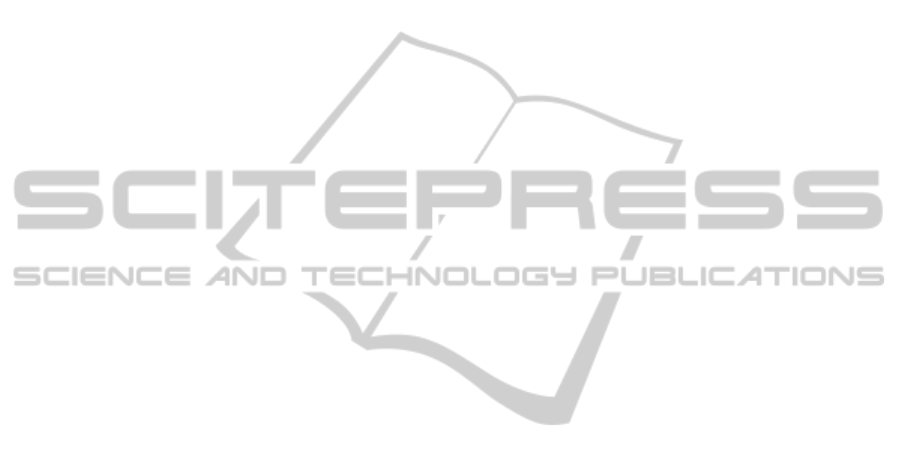
fication model indicates that genre is the leading fac-
tor (over n-gram length and Web page profile size) in
predicting the outcome of the classification. Although
genre is an influential factor in predicting the classifi-
cation performance, a specific hypothesis about which
genres can be better classified than othershas not been
developed. The variability between genres is likely to
be caused by a factor that has not been explored as
part of the current research, such as the length of the
Web pages, or the homogeneity of each genre.
4.4 The Effect of Noise
As shown in Tables 2, 4, and 6, the addition of 750
noise Web pages to the Syracuse corpus resulted in a
slight decrease in the precision of the centroid clas-
sifier, and a slight increase in the recall. Thus, the
noise Web pages are less likely to be mislabeled as be-
longing to another genre than are the non-noise Web
pages. Of the 1985 non-noise Web pages, an aver-
age of 170 pages (8.6%) were erroneously labeled as
noise Web pages by the centroid model; of the 750
noise Web pages, an average of 3 pages (0.4%) were
erroneously labeled as non-noise pages. The number
of Web pages erroneously labeled as noise increases
from 3.6% to 13.3% as the n-gram length was in-
creased from 2 to 4, whereas the number of noise
pages erroneously given genre labels decreases from
0.85% to 0.03% as the n-gram length was increased
from 2 to 4. This suggests that the proportion of noise
Web pages expected to appear in a corpus could influ-
ence the choice of the n-gram length to be used.
5 CONCLUSIONS
The major contribution of this study is to show that
byte n-gram Web page representations can be used
effectively, with more than one classification model,
to classify Web pages by genre, even when the Web
pages belong to more than one genre or to no known
genre, and when the number of Web pages in each
genre is quite variable. The results of these experi-
ments also showed that in general, as the length of
the n-grams used to represent the Web pages was
increased, the classification performance for each
model decreased. The results also indicated that over
the range of 15 to 50, the number of n-grams used to
represent each Web page has only a slight impact on
the classification results.
REFERENCES
Crowston, K. and Williams, M. (1997). Reproduced and
Emergent Genres of Communication on the World-
Wide Web. In Proc. 30th Hawaii Intl. Conf. on System
Sciences, pages 30–39.
Finn, A. and Kushmerick, N. (2006). Learning to Classify
Documents According to Genre. Journal of Ameri-
can Society for Information Science and Technology,
57(11):1506–1518.
Jebari, C. (2008). Refined and Incremental Centroid-based
Approach for Genre Categorization of Web Pages. In
Proc. 17th Intl. World Wide Web Conf.
Kanaris, I. and Stamatatos, E. (2009). Learning to Rec-
ognize Webpage Genres. Information Processing &
Management, 45(5):499–512.
Keˇselj, V., Peng, F., Cercone, N., and Thomas, T. (2003). N-
gram-based author profiles for authorship attribution.
In Proc. Conf. Pacific Association for Computational
Linguistics, pages 255–264.
Levering, R., Cutler, M., and Yu, L. (2008). Using Vi-
sual Features for Fine-Grained Genre Classification of
Web Pages. In Proc. 41st Hawaii Intl. Conf. on System
Sciences. IEEE Computer Society.
Mason, J., Shepherd, M., and Duffy, J. (2009a). An N-
gram Based Approach to Automatically Identifying
Web Page Genre. In Proc. 42nd Hawaii Intl. Conf.
on System Sciences.
Mason, J., Shepherd, M., and Duffy, J. (2009b). Classifying
Web Pages by Genre: A Distance Function Approach.
In Proc. 5th Intl. Conf. on Web Information Systems
and Technologies.
Mason, J., Shepherd, M., and Duffy, J. (2009c). Classifying
Web Pages by Genre: An n-gram Based Approach. In
Proc. Intl. Conf. on Web Intelligence.
Mason, J., Shepherd, M., Duffy, J., Keˇselj, V., and Wat-
ters, C. (2010). An n-gram Based Approach to Multi-
labeled Web Page Genre Classification. In Proc. 43rd
Hawaii Intl. Conf. on System Sciences.
Meyer zu Eissen, S. and Stein, B. (2004). Genre Classifi-
cation of Web Pages. In Proc. 27th German Conf. on
Artificial Intelligence. Springer.
Rosso, M. (2008). User-based identification of Web gen-
res. Journal of the American Society for Information
Science and Technology, 59(7).
Santini, M. (2008). Zero, Single, or Multi? Genre of Web
Pages Through the Users’ Perspective. Information
Processing and Management, 44(2):702–737.
Shepherd, M., Watters, C., and Kennedy, A. (2004). Cyber-
genre: Automatic Identification of Home Pages on the
Web. Journal of Web Engineering, 3(3&4):236–251.
Stein, B. and Meyer zu Eissen, S. (2008). Retrieval Mod-
els for Genre Classification. Scandinavian Journal of
Information Systems, 20(1):93–119.
Vidulin, V., Luˇstrek, M., and Gams, M. (2007). Training
the Genre Classifier for Automatic Classification of
Web Pages. In Proc. 29th Intl. Conf. on Information
Technology Interfaces, pages 93–98.
WEBIST 2011 - 7th International Conference on Web Information Systems and Technologies
594