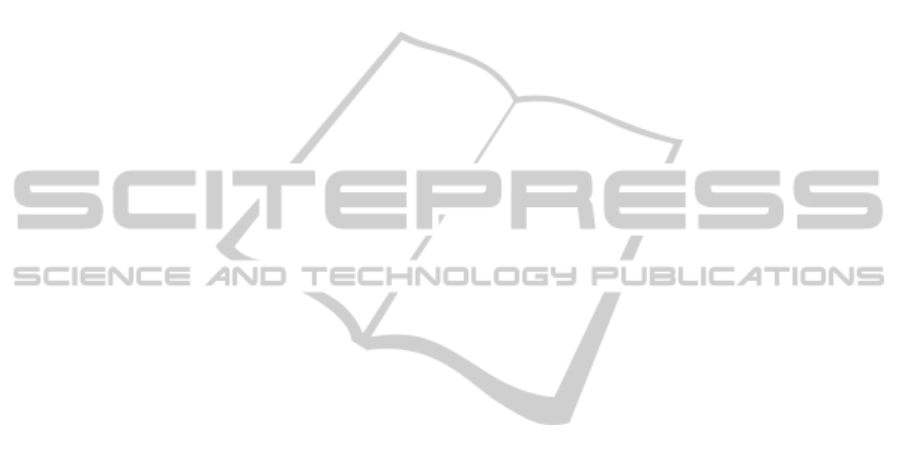
a selected cluster will give us better results than se-
lecting the fusion of the entire group of membership
functions. This is confirmed by calculating the mem-
bership function over the set-theoretic operations on
group E and cluster A. It is seen that the membership
grades in group E for small values (x≤10) are lower
than the membership grades in cluster A for the same
values.
For validation purposes, other clusters were ana-
lyzed and we obtained similar results but they are not
shown here due to space limits. Based on set-theoretic
operations, several membership functions were fu-
sioned to obtain a result membership function that
represents a trend or a suitable concept among several
opinions. Although, we have presented our results us-
ing the basic operations proposed by Zadeh, we have
evaluated our proposal with: 1) the Lukasiewicz t-
norm and t-conorm; and 2) the product and the proba-
bilistic sum. However, we obtained slightly different
results only for the t-norm over group E. More ad-
vanced fusion techniques are possible and subject to
further study. Here the use of union and intersection
operations are used to demonstrate the shape-based
similarity of membership functions.
5 CONCLUSIONS AND FURTHER
WORK
This paper proposed a novel method to annotate mem-
bership functions and build a string representation for
them. This string is used to detect similar-shaped
membership functions by string comparisons.
Similar membership functions were clustered con-
sidering that they represent a trend or a suitable con-
cept in a decision-making context. We proposed some
cluster characteristics to be taken into account for fur-
ther analysis. Additionally, for any selected cluster,
positive differences are obtained when comparing the
complete set of membership functions and a reduced
set grouped by shape-similarity.
The cluster selected for fusion is further processed
using set-theoretic operations. Other strategies are ex-
posed for further consideration.
The proposed similarity measure could be ex-
tended in order to take into account other shape char-
acteristics like the core length (e.g., a triangular mem-
bership function and a trapezoidal membership func-
tion with a tiny core).
This proposal settles some opportunities for future
work on different areas where diverse points of view
are present like group decision-making and suitability
analysis. Some application areas like fuzzy control,
based on this proposal (e.g., to find similarities on rule
engines) among other applications could be explored.
ACKNOWLEDGEMENTS
This research is supported by Escuela Superior
Polit
´
ecnica del Litoral (ESPOL) and it is financed by
Secretar
´
ıa de Educaci
´
on Superior en Ciencia y Tec-
nolog
´
ıa (SENESCYT) under Ph.D. studies 2012.
REFERENCES
Bouchon-Meunier, B. and Rifqi, M. (1996). Towards gen-
eral measures of comparison of objects. Fuzzy Sets
and Systems, 84:143–153.
Dice, L. R. (1945). Measures of the Amount of Ecologic
Association Between Species. Ecology, 26(3):297–
302.
Dubois, D. and Prade, H. (1980). Fuzzy Sets and Systems:
Theory and Applications. Academic Press, Inc.
Dujmovi
´
c, J. and De Tr
´
e, G. (2011). Multicriteria meth-
ods and logic aggregation in suitability maps. Inter-
national Journal of Intelligent Systems, 26(10):971–
1001.
Jaccard, P. (1908). Nouvelles recherches sur la distribution
florale. Bulletin de la Soci
`
ete Vaudense des Sciences
Naturelles, 44:223–270.
Johany
´
ak, Z. and Kov
´
acs, S. (2005). Distance based sim-
ilarity measures of fuzzy sets. Proceedings of SAMI,
1.
Kacprzyk, J., Fedrizzi, M., and Nurmi, H. (1992). Group
decision making and consensus under fuzzy prefer-
ences and fuzzy majority. Fuzzy Sets and Systems,
49(1):21–31.
Klir, G. J. and Yuan, B. (1995). Fuzzy Sets and Fuzzy Logic:
Theory and Applications. Prentice Hall.
Le Capitaine, H. (2012). A relevance-based learning model
of fuzzy similarity measures. IEEE Transactions on
Fuzzy Systems, 20(1):57–68.
Lee-Kwang, H., Song, Y.-S., and Lee, K.-M. (1994). Sim-
ilarity measure between fuzzy sets and between ele-
ments. Fuzzy Sets and Systems, 62(3):291–293.
Merig
´
o, J. and Casanovas, M. (2010). Decision mak-
ing with distance measures and linguistic aggregation
operators. International Journal of Fuzzy Systems,
12(3):190–198.
Rodr
´
ıguez, R. M., Mart
´
ınez, L., and Herrera, F. (2012).
Hesistant Fuzzy Linguistic Term Sets for Decision
Making. IEEE Transactions on Fuzzy Systems,
20(1):109–119.
Tversky, D. (1977). Features of similarities. Psychological
Review, 84:327–352.
Xuecheng, L. (1992). Entropy, distance measure and simi-
larity measure of fuzzy sets and their relations. Fuzzy
Sets and Systems, 52(3):305–318.
IJCCI2012-InternationalJointConferenceonComputationalIntelligence
408