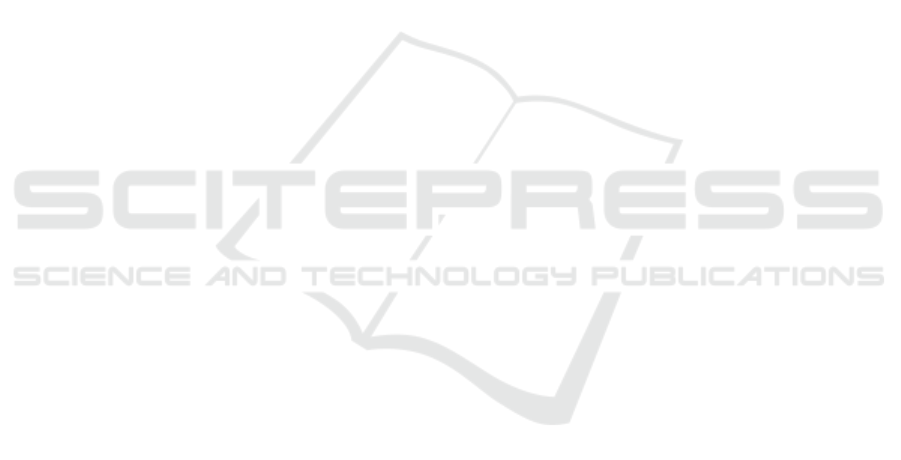
ics: A model of household demography. Journal of
Artificial Societies and Social Simulation, 16(1):8.
Germann, T. C., Kadau, K., Longini, I. M., and Macken,
C. A. (2006). Mitigation strategies for pandemic in-
fluenza in the united states. Proceedings of the Na-
tional Academy of Sciences, 103(15):5935–5940.
Heesterbeek, J. A. P. (2002). A brief history of r
0
and a recipe for its calculation. Acta biotheoretica,
50(3):189–204.
Heffernan, J. M., Smith, R. J., and Wahl, L. M. (2005). Per-
spectives on the basic reproductive ratio. Journal of
the Royal Society Interface, 2(4):281–293.
Hoertel, N., Blachier, M., Blanco, C., Olfson, M., Massetti,
M., Rico, M., Limosin, F., and Leleu, H. (2020). A
stochastic agent-based model of the sars-cov-2 epi-
demic in france. Nature Medicine, 26:1–5.
Hunter, E., Mac Namee, B., and Kelleher, J. (2018). An
open-data-driven agent-based model to simulate in-
fectious disease outbreaks. PLOS ONE, 13(12):1–35.
Krumkamp, R., Kretzschmar, M., Rudge, J., Ahmad, A.,
Hanvoravongchai, P., Westenhöfer, J., Stein, M., Put-
thasri, W., and Coker, R. (2011). Health service re-
source needs for pandemic influenza in developing
countries: a linked transmission dynamics, interven-
tions and resource demand model. Epidemiology &
Infection, 139(1):59–67.
Meltzer, M. I., Cox, N. J., and Fukuda, K. (1999). The
economic impact of pandemic influenza in the united
states: priorities for intervention. Emerging infectious
diseases, 5(5):659.
National Statistics, ROC (Taiwan). Directorate General of
Budget, Accounting and Statistics (DGBAS) of Exec-
utive Yuan. https://eng.stat.gov.tw/. Online; Accessed:
2021 May.
Park, J.-E. and Ryu, Y. (2018). Transmissibility and severity
of influenza virus by subtype. Infection, Genetics and
Evolution, 65:288–292.
Perkins, T. A., Reiner, Jr., R. C., España, G., ten Bosch,
Q. A., Verma, A., Liebman, K. A., Paz-Soldan,
V. A., Elder, J. P., Morrison, A. C., Stoddard, S. T.,
Kitron, U., Vazquez-Prokopec, G. M., Scott, T. W.,
and Smith, D. L. (2019). An agent-based model
of dengue virus transmission shows how uncertainty
about breakthrough infections influences vaccination
impact projections. PLOS Computational Biology,
15(3):1–32.
Richardson, S., Hirsch, J. S., Narasimhan, M., Crawford,
J. M., McGinn, T., Davidson, K. W., , and the North-
well COVID-19 Research Consortium (2020). Pre-
senting characteristics, comorbidities, and outcomes
among 5700 patients hospitalized with covid-19 in the
new york city area. JAMA, 323(20):2052–2059.
Riley, S. (2007). Large-scale spatial-transmission models
of infectious disease. Science, 316(5829):1298–1301.
Taiwan, National Development Council, Executive Yuan
(2020). Population Projections for ROC (Tai-
wan): 2020 2070. https://pop-proj.ndc.gov.tw/
main_en/dataSearch.aspx?uid=78&pid=78&upn=
8D038F3F06D3982D. Accessed: 2021-05-21.
Tsai, M.-T., Chern, T.-C., Chuang, J.-H., Hsueh, C.-W.,
Kuo, H.-S., Liau, C.-J., Riley, S., Shen, B.-J., Shen,
C.-H., Wang, D.-W., and Hsu, T.-s. (2010). Efficient
simulation of the spatial transmission dynamics of in-
fluenza. PloS one, 5(11):e13292.
Tsay, C., Lejarza, F., Stadtherr, M., and Baldea, M. (2020).
Modeling, state estimation, and optimal control for the
us covid-19 outbreak. Scientific Reports, 10:10711.
Venkatramanan, S., Lewis, B., Chen, J., Higdon, D., Vul-
likanti, A., and Marathe, M. (2018). Using data-driven
agent-based models for forecasting emerging infec-
tious diseases. Epidemics, 22:43–49. The RAPIDD
Ebola Forecasting Challenge.
World Health Organization (2020). Decade of healthy
ageing (2021-2030). https://www.who.int/initiatives/
decade-of-healthy-ageing. Accessed: 2021-05-21.
Wright, D. W., Richardson, R. A., Edeling, W., Lakhlili,
J., Sinclair, R. C., Jancauskas, V., Suleimenova, D.,
Bosak, B., Kulczewski, M., Piontek, T., Kopta, P.,
Chirca, I., Arabnejad, H., Luk, O. O., Hoenen, O.,
Weglarz, J., Crommelin, D., Groen, D., and Coveney,
P. V. (2020). Building confidence in simulation: Ap-
plications of easyvvuq. Advanced Theory and Simu-
lations, 3(8):1900246.
Wu, J., Dhingra, R., Gambhir, M., and Remais, J. V.
(2013). Sensitivity analysis of infectious disease mod-
els: methods, advances and their application. Journal
of The Royal Society Interface, 10(86):20121018.
SIMULTECH 2021 - 11th International Conference on Simulation and Modeling Methodologies, Technologies and Applications
460