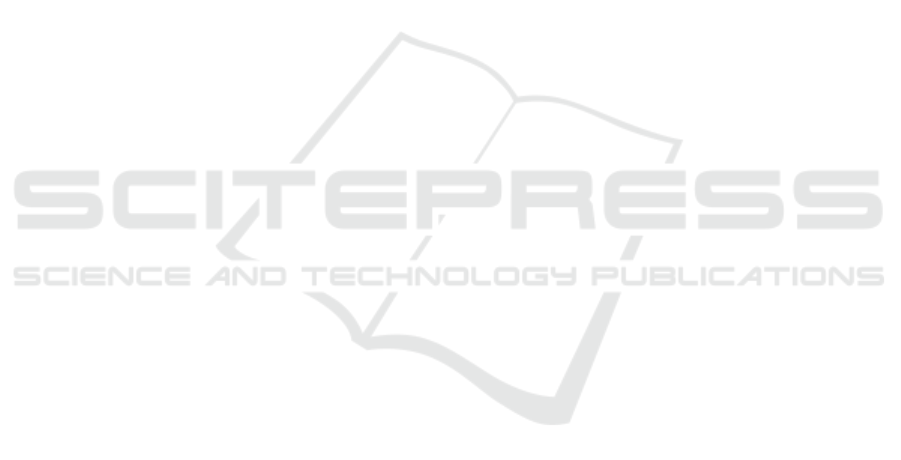
curacy is a highly preferred metric in binary classifi-
cation, it is subjected to be biased if there is a class-
imbalance. Therefore it is given less importance com-
pared to the metrics where true positive rate is in-
volved. The least importance is given to the metrics
which focus on negative class because they are not
so important in medical diagnosis. Table 4 presents
the ranking order of classifiers based on the impor-
tance weights in Table 5. It can be observed from Ta-
ble 4 that TOPSIS, VIKOR, and PROMETHEE has
assigned first rank to same classifier for all the five
datasets after assigning importance to metrics. The
ranks assigned by SAW is almost similar to TOP-
SIS, VIKOR, and PROMETHEE whereas the ranks
assigned by MEW are quite dissimilar. In between
Table 3 and 4, the consistent ranking can be observed
for the top performed classifiers by all the MCDM
methods in case of Table 4. By this, we can iden-
tify the fact that correctly weighting the criteria to a
particular problem will result in a similar ranking by
most of the MCDM method. In Table 4, for diabetes
dataset, logistic regression, random forest and na
¨
ıve
bayes are the top ranked classifiers by various MCDM
methods, whereas random forest is followed by logis-
tic regression for both liver and breast datasets. Na
¨
ıve
bayes is followed by logistic regression, and random
forest is followed by logistic regression for heart and
hepatitis datasets respectively.
It can observed from Table 4 that, SVM, lin-
ear model, neural network, and decision tree has not
given preference compared to random forest, logis-
tic regression and na
¨
ıve bayes. By considered the
majority voting, this work concludes the classifier
which is ranked as one by TOPSIS, VIKOR, and
PROMETHEE can be chosen as optimal for corre-
sponding datasets. Therefore, random forest classifier
is chosen as the optimal for liver and breast datasets,
logistic regression for diabetes and hepatitis dataset,
and finally na
¨
ıve bayes classifier for heart dataset.
4 CONCLUSION
This work focuses on providing a preference or-
der of classifiers based on the importance of perfor-
mance metrics which is recorded as ScaPMI value.
A brief overview on various performance metrics and
their evaluation focus is provided so that the task of
assigning ScaPMI value to metrics becomes easier.
Moreover, the preference order of classifiers gener-
ated based on equal ScaPMI value to all the metrics
aid decision-maker in attaining baseline knowledge.
Decision-makers can generate the desired preference
order of classifiers by varying the ScaPMI value of
metrics with the knowledge attained from the prefer-
ence order by equal ScaPMI values. Since, all the
datasets in this work are from medical domain, high
ScaPMI value is given to recall and less ScaPMI value
is given to cross-entropy loss. The classifier to which
most of the MCDM methods has assigned the first
rank is considered as an optimal classifier for corre-
sponding dataset.
As a further extension, this work intends to in-
corporate statistical methods to verify the significance
of the difference among the ranking orders generated
by various MCDM methods. Moreover, an enhanced
study of the performance metrics and the evaluation
focus supports in modeling better criteria and their
weights to rank the classifiers precisely. A series of
experiments on non-medical datasets with multiple
weighting strategies helps in verifying the rationality
and the effectiveness of the ranking methods.
REFERENCES
Asadabadi, M. R., Chang, E., and Saberi, M. (2019). Are
mcdm methods useful? a critical review of analytic
hierarchy process (ahp) and analytic network process
(anp). Cogent Engineering, 6(1):1623153.
Baccour, L. (2018). Amended fused topsis-vikor for classi-
fication (atovic) applied to some uci data sets. Expert
Systems with Applications, 99:115–125.
Brans, J.-P. and De Smet, Y. (2016). Promethee methods.
In Multiple criteria decision analysis, pages 187–219.
Springer.
Christopher, J. (2019). The science of rule-based classifiers.
In 2019 9th International Conference on Cloud Com-
puting, Data Science & Engineering (Confluence),
pages 299–303. IEEE.
Guhathakurata, S., Saha, S., Kundu, S., Chakraborty, A.,
and Banerjee, J. S. (2021). South asian countries are
less fatal concerning covid-19: a fact-finding proce-
dure integrating machine learning & multiple criteria
decision-making (mcdm) technique. Journal of The
Institution of Engineers (India): Series B, pages 1–15.
Kavya, R., Christopher, J., Panda, S., and Lazarus, Y. B.
(2021). Machine learning and xai approaches for al-
lergy diagnosis. Biomedical Signal Processing and
Control, 69:102681.
Naser, M. and Alavi, A. (2020). Insights into performance
fitness and error metrics for machine learning. arXiv
preprint arXiv:2006.00887.
Osisanwo, F., Akinsola, J., Awodele, O., Hinmikaiye, J.,
Olakanmi, O., and Akinjobi, J. (2017). Supervised
machine learning algorithms: classification and com-
parison. International Journal of Computer Trends
and Technology (IJCTT), 48(3):128–138.
Peteiro-Barral, D., Remeseiro, B., M
´
endez, R., and Penedo,
M. G. (2017). Evaluation of an automatic dry eye test
using mcdm methods and rank correlation. Medical &
biological engineering & computing, 55(4):527–536.
ICAART 2022 - 14th International Conference on Agents and Artificial Intelligence
90