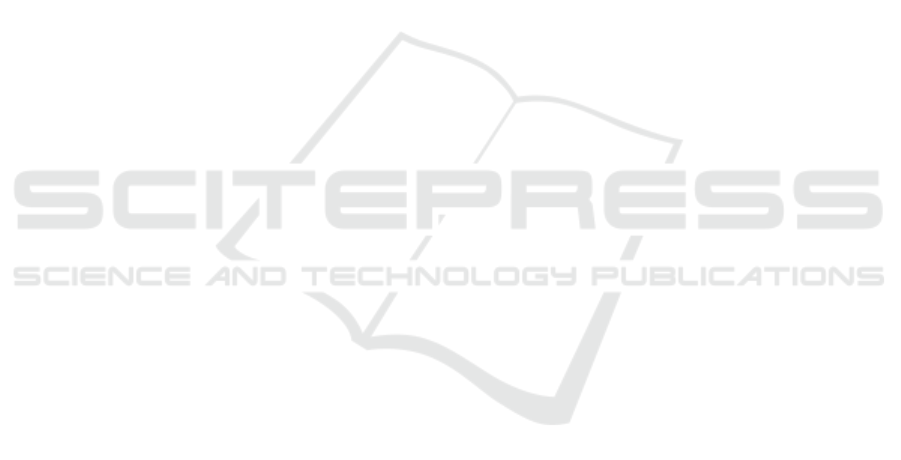
implement new tasks and generate new data. We envi-
sion that our dataset can be a useful basis for training
object detection methods and develop motion fore-
casting and action recognition algorithms in the con-
text of HRC.
We plan to further research algorithms for motion
forecasting in the industrial context with the use of
the proposed dataset. Therefore, we not only want
to use hand motion information to predict the future,
but combine it with semantic information. Above all,
we want to address problems inherent in time series
classification and other approaches, such as limitation
to short time horizons and the lack of generalizability
with respect to variations in the scene.
REFERENCES
Aksoy, E. E., Tamosiunaite, M., and W
¨
org
¨
otter, F. (2015).
Model-free incremental learning of the semantics of
manipulation actions. Robotics and Autonomous Sys-
tems, 71:118–133.
Cao, Z., Hidalgo Martinez, G., Simon, T., Wei, S., and
Sheikh, Y. A. (2019). Openpose: Realtime multi-
person 2d pose estimation using part affinity fields.
IEEE Transactions on Pattern Analysis and Machine
Intelligence.
Colgate, J. E. and Peshkin, M. A. (1999). Cobots. US Patent
No. 5952796A.
Dallel, M., Havard, V., Baudry, D., and Savatier, X.
(2020). InHARD - Industrial Human Action Recogni-
tion Dataset in the Context of Industrial Collaborative
Robotics. In 2020 IEEE International Conference on
Human-Machine Systems (ICHMS), pages 1–6.
Diller, C., Funkhouser, T. A., and Dai, A. (2020). Forecast-
ing characteristic 3d poses of human actions. CoRR,
abs/2011.15079.
Dreher, C. R. G., W
¨
achter, M., and Asfour, T. (2020).
Learning Object-Action Relations from Bimanual Hu-
man Demonstration Using Graph Networks. IEEE
Robotics and Automation Letters, 5(1):187–194.
Dutta, A. and Zisserman, A. (2019). The VIA Annotation
Software for Images, Audio and Video. In Proceed-
ings of the 27th ACM International Conference on
Multimedia, pages 2276–2279, Nice France. ACM.
Fragkiadaki, K., Levine, S., and Malik, J. (2015). Recur-
rent network models for kinematic tracking. CoRR,
abs/1508.00271.
He, K., Gkioxari, G., Doll
´
ar, P., and Girshick, R. (2018).
Mask R-CNN. arXiv:1703.06870 [cs].
Ji, Y., Yang, Y., Shen, F., Shen, H. T., and Li, X. (2020).
A Survey of Human Action Analysis in HRI Applica-
tions. IEEE Transactions on Circuits and Systems for
Video Technology, 30(7):2114–2128.
Lasota, P. A. (2017). A multiple-predictor approach to hu-
man motion prediction. IEEE International Confer-
ence on Robotics and Automation (ICRA).
Li, C., Zhang, Z., Lee, W. S., and Lee, G. H. (2018). Con-
volutional sequence to sequence model for human dy-
namics. CoRR, abs/1805.00655.
Luo, R. and Berenson, D. (2015). A framework for un-
supervised online human reaching motion recognition
and early prediction. In 2015 IEEE/RSJ International
Conference on Intelligent Robots and Systems (IROS),
pages 2426–2433.
Luo, R. and Mai, L.-C. (2019). Human Intention Infer-
ence and On-Line Human Hand Motion Prediction for
Human-Robot Collaboration. IEEE/RSJ International
Conference on Intelligent Robots and Systems (IROS).
Martinez, J., Black, M. J., and Romero, J. (2017). On
human motion prediction using recurrent neural net-
works. CoRR, abs/1705.02445.
Matheson, E., Minto, R., Zampieri, E. G. G., Faccio, M.,
and Rosati, G. (2019). Human–Robot Collaboration
in Manufacturing Applications: A Review. Robotics,
8(4):100.
Perez-D’Arpino, C. and Shah, J. A. (2015). Fast target
prediction of human reaching motion for coopera-
tive human-robot manipulation tasks using time se-
ries classification. In 2015 IEEE International Con-
ference on Robotics and Automation (ICRA), pages
6175–6182, Seattle, WA, USA. IEEE.
Quigley, M., Gerkey, B., Conley, K., Faust, J., Foote,
T., Leibs, J., Berger, E., Wheeler, R., and Ng, A.
(2009). ROS: an open-source Robot Operating Sys-
tem. page 6.
Salvador, S. and Chan, P. (2007). FastDTW: Toward Ac-
curate Dynamic Time Warping in Linear Time and
Space. page 11.
Savitzky, A. and Golay, M. J. E. (1964). Smoothing and
differentiation of data by simplified least squares pro-
cedures. Analytical Chemistry, 36(8):1627–1639.
Simon, T., Joo, H., Matthews, I., and Sheikh, Y. (2017).
Hand keypoint detection in single images using mul-
tiview bootstrapping. In CVPR.
Tsai, R. and Lenz, R. (1989). A new technique for fully au-
tonomous and efficient 3D robotics hand/eye calibra-
tion. IEEE Transactions on Robotics and Automation,
5(3):345–358.
Wang, Z., Wang, B., Liu, H., and Kong, Z. (2017). Re-
current convolutional networks based intention recog-
nition for human-robot collaboration tasks. In 2017
IEEE International Conference on Systems, Man, and
Cybernetics (SMC), pages 1675–1680, Banff, AB.
IEEE.
Zanchettin, A. M. and Rocco, P. (2017). Probabilistic
inference of human arm reaching target for effec-
tive human-robot collaboration. In 2017 IEEE/RSJ
International Conference on Intelligent Robots and
Systems (IROS), pages 6595–6600, Vancouver, BC.
IEEE.
Zhang, F., Bazarevsky, V., Vakunov, A., Tkachenka, A.,
Sung, G., Chang, C., and Grundmann, M. (2020).
Mediapipe hands: On-device real-time hand tracking.
CoRR, abs/2006.10214.
CoAx: Collaborative Action Dataset for Human Motion Forecasting in an Industrial Workspace
105