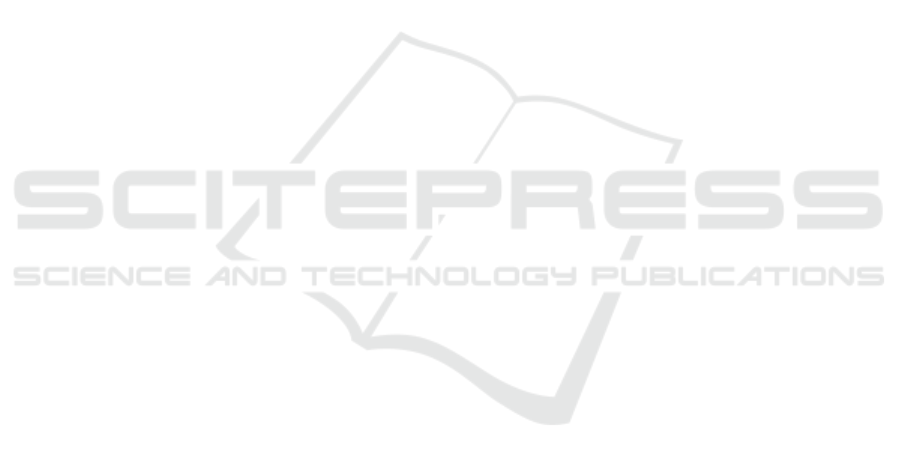
on Computer Vision and Pattern Recognition (CVPR
2009), 20-25 June 2009, Miami, Florida, USA, pages
248–255. IEEE Computer Society. 3, 7
Goodfellow, I. J., Pouget-Abadie, J., Mirza, M., Xu, B.,
Warde-Farley, D., Ozair, S., Courville, A. C., and
Bengio, Y. (2014). Generative adversarial nets. In
Ghahramani, Z., Welling, M., Cortes, C., Lawrence,
N. D., and Weinberger, K. Q., editors, Advances in
Neural Information Processing Systems 27: Annual
Conference on Neural Information Processing Sys-
tems 2014, December 8-13 2014, Montreal, Quebec,
Canada, pages 2672–2680. 4, 7
He, K., Zhang, X., Ren, S., and Sun, J. (2016). Deep
residual learning for image recognition. In 2016
IEEE Conference on Computer Vision and Pattern
Recognition, CVPR 2016, Las Vegas, NV, USA, June
27-30, 2016, pages 770–778. IEEE Computer Society.
3
Hoffman, J., Tzeng, E., Park, T., Zhu, J., Isola, P., Saenko,
K., Efros, A. A., and Darrell, T. (2018). Cycada:
Cycle-consistent adversarial domain adaptation. In
Dy, J. G. and Krause, A., editors, Proceedings of the
35th International Conference on Machine Learning,
ICML 2018, Stockholmsm
¨
assan, Stockholm, Sweden,
July 10-15, 2018, volume 80 of Proceedings of Ma-
chine Learning Research, pages 1994–2003. PMLR.
2, 6
Hoffman, J., Wang, D., Yu, F., and Darrell, T. (2016). Fcns
in the wild: Pixel-level adversarial and constraint-
based adaptation. CoRR, abs/1612.02649. 2
Hong, W., Wang, Z., Yang, M., and Yuan, J. (2018).
Conditional generative adversarial network for struc-
tured domain adaptation. In 2018 IEEE Confer-
ence on Computer Vision and Pattern Recognition,
CVPR 2018, Salt Lake City, UT, USA, June 18-22,
2018, pages 1335–1344. Computer Vision Foundation
/ IEEE Computer Society. 2
Karras, T., Aila, T., Laine, S., and Lehtinen, J. (2018).
Progressive growing of gans for improved quality, sta-
bility, and variation. In 6th International Conference
on Learning Representations, ICLR 2018, Vancouver,
BC, Canada, April 30 - May 3, 2018, Conference
Track Proceedings. OpenReview.net. 3
Ke, Z., Wang, D., Yan, Q., Ren, J. S. J., and Lau, R.
W. H. (2019). Dual student: Breaking the limits
of the teacher in semi-supervised learning. In 2019
IEEE/CVF International Conference on Computer Vi-
sion, ICCV 2019, Seoul, Korea (South), October 27 -
November 2, 2019, pages 6727–6735. IEEE. 2
Kingma, D. P. and Ba, J. (2015). Adam: A method for
stochastic optimization. In Bengio, Y. and LeCun,
Y., editors, 3rd International Conference on Learning
Representations, ICLR 2015, San Diego, CA, USA,
May 7-9, 2015, Conference Track Proceedings. 7
Lee, C., Batra, T., Baig, M. H., and Ulbricht, D. (2019).
Sliced wasserstein discrepancy for unsupervised do-
main adaptation. In IEEE Conference on Computer
Vision and Pattern Recognition, CVPR 2019, Long
Beach, CA, USA, June 16-20, 2019, pages 10285–
10295. Computer Vision Foundation / IEEE. 6
Lee, D.-H. (2013). Pseudo-label: The simple and efficient
semi-supervised learning method for deep neural net-
works. In Workshop on challenges in representation
learning, ICML, volume 3, page 896. 2
Long, J., Shelhamer, E., and Darrell, T. (2015). Fully
convolutional networks for semantic segmentation. In
IEEE Conference on Computer Vision and Pattern
Recognition, CVPR 2015, Boston, MA, USA, June 7-
12, 2015, pages 3431–3440. IEEE Computer Society.
2
Mao, X., Li, Q., Xie, H., Lau, R. Y. K., Wang, Z., and Smol-
ley, S. P. (2017). Least squares generative adversarial
networks. In IEEE International Conference on Com-
puter Vision, ICCV 2017, Venice, Italy, October 22-29,
2017, pages 2813–2821. IEEE Computer Society. 7
Murez, Z., Kolouri, S., Kriegman, D. J., Ramamoorthi,
R., and Kim, K. (2018). Image to image translation
for domain adaptation. In 2018 IEEE Conference
on Computer Vision and Pattern Recognition, CVPR
2018, Salt Lake City, UT, USA, June 18-22, 2018,
pages 4500–4509. Computer Vision Foundation /
IEEE Computer Society. 2
Odena, A., Olah, C., and Shlens, J. (2017). Conditional
image synthesis with auxiliary classifier gans. In
Precup, D. and Teh, Y. W., editors, Proceedings of the
34th International Conference on Machine Learning,
ICML 2017, Sydney, NSW, Australia, 6-11 August
2017, volume 70 of Proceedings of Machine Learning
Research, pages 2642–2651. PMLR. 4
Pizzati, F., de Charette, R., Zaccaria, M., and Cerri, P.
(2020). Domain bridge for unpaired image-to-image
translation and unsupervised domain adaptation. In
IEEE Winter Conference on Applications of Computer
Vision, WACV 2020, Snowmass Village, CO, USA,
March 1-5, 2020, pages 2979–2987. IEEE. 2
Richter, S. R., Vineet, V., Roth, S., and Koltun, V. (2016).
Playing for data: Ground truth from computer games.
In Leibe, B., Matas, J., Sebe, N., and Welling, M., ed-
itors, Computer Vision - ECCV 2016 - 14th European
Conference, Amsterdam, The Netherlands, October
11-14, 2016, Proceedings, Part II, volume 9906 of
Lecture Notes in Computer Science, pages 102–118.
Springer. 6
Ros, G., Sellart, L., Materzynska, J., V
´
azquez, D., and
L
´
opez, A. M. (2016). The SYNTHIA dataset: A large
collection of synthetic images for semantic segmen-
tation of urban scenes. In 2016 IEEE Conference
on Computer Vision and Pattern Recognition, CVPR
2016, Las Vegas, NV, USA, June 27-30, 2016, pages
3234–3243. IEEE Computer Society. 6
Sankaranarayanan, S., Balaji, Y., Jain, A., Lim, S., and
Chellappa, R. (2018). Learning from synthetic data:
Addressing domain shift for semantic segmentation.
In 2018 IEEE Conference on Computer Vision and
Pattern Recognition, CVPR 2018, Salt Lake City, UT,
USA, June 18-22, 2018, pages 3752–3761. Computer
Vision Foundation / IEEE Computer Society. 2
Simonyan, K. and Zisserman, A. (2015). Very deep convo-
lutional networks for large-scale image recognition. In
Bengio, Y. and LeCun, Y., editors, 3rd International
Conference on Learning Representations, ICLR 2015,
ICAART 2022 - 14th International Conference on Agents and Artificial Intelligence
140