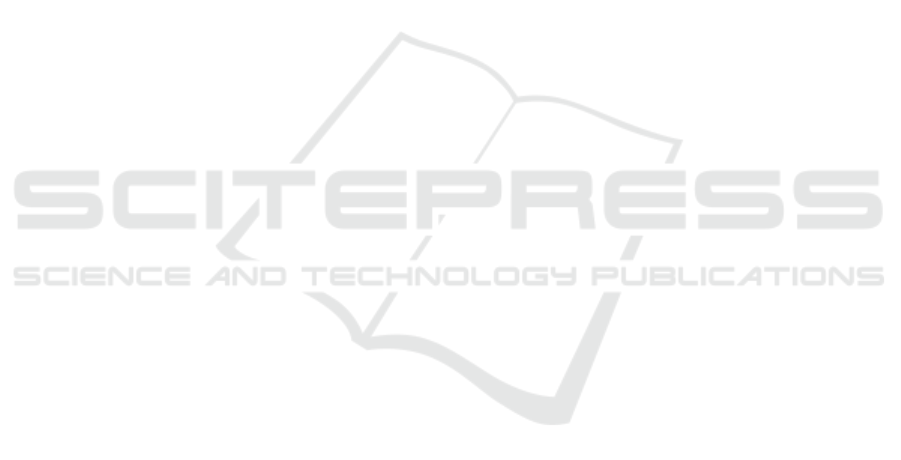
5 CONCLUSIONS
In this paper, a computational geometry-based algo-
rithm is proposed for selecting the optimal number
of channels which is based on non-linear separabil-
ity measurement. The proposed algorithm is evalu-
ated on both BCI competition IV datasets IIa and IIb.
In this work, an effect of low NLM metric (λ) value
on high accuracy has been investigated by using the
EEG channels that are used to compute such λ. Al-
though, the most effective channels out of the 10 ini-
tial channels have been selected, yet the potential of
all 25 channels has not been explored. Based on pro-
posed NLMCS() algorithm, we are motivated to apply
clustering to compute the λ value for clusters between
two classes. In future, the work will be extended for
all channels and a subject independent model will be
built on features taken from the selected channels de-
sign for use in self evolving neural network for EEG
classification for improved accuracy.
REFERENCES
Abdulkader, S. N., Atia, A., and Mostafa, M.-S. M. (2015).
Brain computer interfacing: Applications and chal-
lenges. Egyptian Informatics Journal, 16(2):213–230.
Alam, M. N., Ibrahimy, M. I., and Motakabber, S. (2021).
Feature extraction of eeg signal by power spectral den-
sity for motor imagery based bci. In 2021 8th Interna-
tional Conference on Computer and Communication
Engineering (ICCCE), pages 234–237. IEEE.
Baig, M. Z., Aslam, N., and Shum, H. P. (2020). Filtering
techniques for channel selection in motor imagery eeg
applications: a survey. Artificial intelligence review,
53(2):1207–1232.
Das, A. and Suresh, S. (2015). An effect-size based chan-
nel selection algorithm for mental task classification
in brain computer interface. In 2015 IEEE Interna-
tional Conference on Systems, Man, and Cybernetics,
pages 3140–3145. IEEE.
Davoudi, A., Ghidary, S. S., and Sadatnejad, K. (2017). Di-
mensionality reduction based on distance preservation
to local mean for symmetric positive definite matrices
and its application in brain–computer interfaces. Jour-
nal of neural engineering, 14(3):036019.
Elizondo, D. (2006). The linear separability problem: Some
testing methods. IEEE Transactions on neural net-
works, 17(2):330–344.
Elizondo, D. A., Birkenhead, R., Gamez, M., Garcia, N.,
and Alfaro, E. (2012). Linear separability and classifi-
cation complexity. Expert Systems with Applications,
39(9):7796–7807.
Gaur, P., Pachori, R. B., Wang, H., and Prasad, G. (2018).
A multi-class eeg-based bci classification using multi-
variate empirical mode decomposition based filtering
and riemannian geometry. Expert Systems with Appli-
cations, 95:201–211.
Gonzalez, A., Nambu, I., Hokari, H., Iwahashi, M., and
Wada, Y. (2013). Towards the classification of single-
trial event-related potentials using adapted wavelets
and particle swarm optimization. In 2013 IEEE In-
ternational Conference on Systems, Man, and Cyber-
netics, pages 3089–3094. IEEE.
Kirar, J. S. and Agrawal, R. (2017). Composite kernel sup-
port vector machine based performance enhancement
of brain computer interface in conjunction with spa-
tial filter. Biomedical Signal Processing and Control,
33:151–160.
Miao, M., Zeng, H., Wang, A., Zhao, C., and Liu, F.
(2017). Discriminative spatial-frequency-temporal
feature extraction and classification of motor im-
agery eeg: An sparse regression and weighted na
¨
ıve
bayesian classifier-based approach. Journal of neuro-
science methods, 278:13–24.
Nicolas-Alonso, L. F. and Gomez-Gil, J. (2012). Brain
computer interfaces, a review. sensors, 12(2):1211–
1279.
Qiu, Z., Jin, J., Lam, H.-K., Zhang, Y., Wang, X., and Ci-
chocki, A. (2016). Improved sffs method for channel
selection in motor imagery based bci. Neurocomput-
ing, 207:519–527.
Shan, H., Xu, H., Zhu, S., and He, B. (2015). A novel
channel selection method for optimal classification in
different motor imagery bci paradigms. Biomedical
engineering online, 14(1):1–18.
Shenoy, H. V. and Vinod, A. P. (2014). An iterative opti-
mization technique for robust channel selection in mo-
tor imagery based brain computer interface. In 2014
IEEE International Conference on Systems, Man, and
Cybernetics (SMC), pages 1858–1863. IEEE.
Xie, X., Yu, Z. L., Lu, H., Gu, Z., and Li, Y. (2016).
Motor imagery classification based on bilinear sub-
manifold learning of symmetric positive-definite ma-
trices. IEEE Transactions on Neural Systems and Re-
habilitation Engineering, 25(6):504–516.
Zhang, C. and Eskandarian, A. (2020). A computation-
ally efficient multiclass time-frequency common spa-
tial pattern analysis on eeg motor imagery. In 2020
42nd Annual International Conference of the IEEE
Engineering in Medicine & Biology Society (EMBC),
pages 514–518. IEEE.
Zhang, J., Chen, M., Hu, S., Cao, Y., and Kozma, R. (2016).
Pnn for eeg-based emotion recognition. In 2016 IEEE
international conference on Systems, Man, and Cy-
bernetics (SMC), pages 002319–002323. IEEE.
Zhang, R., Zong, Q., Dou, L., and Zhao, X. (2019). A novel
hybrid deep learning scheme for four-class motor im-
agery classification. Journal of neural engineering,
16(6):066004.
Zhou, B., Wu, X., Ruan, J., Zhao, L., and Zhang, L. (2019).
How many channels are suitable for independent com-
ponent analysis in motor imagery brain-computer in-
terface. Biomedical Signal Processing and Control,
50:103–120.
ICPRAM 2022 - 11th International Conference on Pattern Recognition Applications and Methods
178