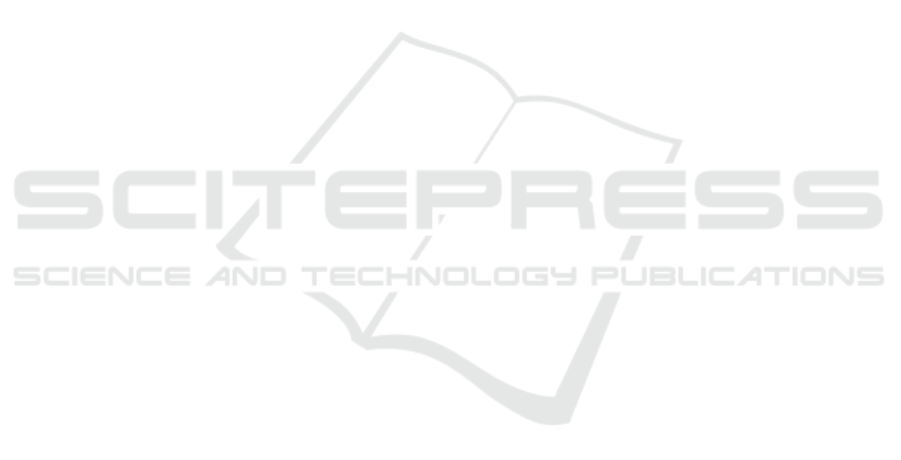
ACKNOWLEDGEMENTS
This work was supported by JSPS Kakenhi Grant
Number 20H04295, 20K20406, and 20K20625. This
research also was funded by the University of Sci-
ence, VNU-HCM, Vietnam under grant number
CNTT 2021-11.
REFERENCES
Anderson, P., He, X., Buehler, C., Teney, D., Johnson, M.,
Gould, S., and Zhang, L. (2018). Bottom-up and top-
down attention for image captioning and visual ques-
tion answering. In Proceedings of the IEEE Con-
ference on Computer Vision and Pattern Recognition
(CVPR).
Devlin, J., Chang, M.-W., Lee, K., and Toutanova, K.
(2019). BERT: Pre-training of deep bidirectional
transformers for language understanding. In Proceed-
ings of the 2019 Conference of the North American
Chapter of the Association for Computational Lin-
guistics: Human Language Technologies, Volume 1
(Long and Short Papers), pages 4171–4186.
Dosovitskiy, A., Beyer, L., Kolesnikov, A., Weissenborn,
D., Zhai, X., Unterthiner, T., Dehghani, M., Minderer,
M., Heigold, G., Gelly, S., Uszkoreit, J., and Houlsby,
N. (2021). An image is worth 16x16 words: Trans-
formers for image recognition at scale. In Interna-
tional Conference on Learning Representations.
Goyal, Y., Khot, T., Summers-Stay, D., Batra, D., and
Parikh, D. (2017). Making the V in VQA matter: Ele-
vating the role of image understanding in Visual Ques-
tion Answering. In Proceedings of the IEEE Con-
ference on Computer Vision and Pattern Recognition
(CVPR).
Gurari, D., Li, Q., Stangl, A. J., Guo, A., Lin, C., Grau-
man, K., Luo, J., and Bigham, J. P. (2018). Vizwiz
grand challenge: Answering visual questions from
blind people. In Proceedings of the IEEE Conference
on Computer Vision and Pattern Recognition (CVPR).
Johnson, J., Hariharan, B., van der Maaten, L., Fei-Fei, L.,
Lawrence Zitnick, C., and Girshick, R. (2017). Clevr:
A diagnostic dataset for compositional language and
elementary visual reasoning. In Proceedings of the
IEEE Conference on Computer Vision and Pattern
Recognition (CVPR).
Le, T., Nguyen, H. T., and Nguyen, M. L. (2020). Integrat-
ing transformer into global and residual image feature
extractor in visual question answering for blind peo-
ple. In 2020 12th International Conference on Knowl-
edge and Systems Engineering (KSE), pages 31–36.
Le, T., Nguyen, H. T., and Nguyen, M. L. (2021a). Multi
visual and textual embedding on visual question an-
swering for blind people. Neurocomputing, 465:451–
464.
Le, T., Nguyen, H. T., and Nguyen, M. L. (2021b). Vi-
sion and text transformer for predicting answerability
on visual question answering. In 2021 IEEE Interna-
tional Conference on Image Processing (ICIP), pages
934–938.
Lin, Y., Meng, Y., Sun, X., Han, Q., Kuang, K., Li, J., and
Wu, F. (2021). Bertgcn: Transductive text classifica-
tion by combining gnn and bert. In Proceedings of the
59th Annual Meeting of the Association for Computa-
tional Linguistics.
Parmar, N., Vaswani, A., Uszkoreit, J., Kaiser, L., Shazeer,
N., Ku, A., and Tran, D. (2018). Image transformer.
In Proceedings of the 35th International Conference
on Machine Learning, volume 80 of Proceedings of
Machine Learning Research, pages 4055–4064.
Ren, S., He, K., Girshick, R., and Sun, J. (2015). Faster
r-cnn: Towards real-time object detection with re-
gion proposal networks. In Cortes, C., Lawrence,
N., Lee, D., Sugiyama, M., and Garnett, R., editors,
Advances in Neural Information Processing Systems,
volume 28, page 91–99. Curran Associates, Inc.
Tan, H. and Bansal, M. (2019). LXMERT: Learning cross-
modality encoder representations from transformers.
In Proceedings of the 2019 Conference on Empirical
Methods in Natural Language Processing and the 9th
International Joint Conference on Natural Language
Processing (EMNLP-IJCNLP), pages 5100–5111.
Tan, M. and Le, Q. (2019). EfficientNet: Rethinking model
scaling for convolutional neural networks. In Chaud-
huri, K. and Salakhutdinov, R., editors, Proceedings of
the 36th International Conference on Machine Learn-
ing, volume 97 of Proceedings of Machine Learning
Research, pages 6105–6114. PMLR.
Wang, T., Huang, J., Zhang, H., and Sun, Q. (2020). Vi-
sual commonsense r-cnn. In IEEE/CVF Conference
on Computer Vision and Pattern Recognition (CVPR).
Yang, Z., Dai, Z., Yang, Y., Carbonell, J., Salakhutdinov,
R. R., and Le, Q. V. (2019). Xlnet: Generalized
autoregressive pretraining for language understand-
ing. In Wallach, H., Larochelle, H., Beygelzimer,
A., d'Alch
´
e-Buc, F., Fox, E., and Garnett, R., editors,
Advances in Neural Information Processing Systems,
volume 32. Curran Associates, Inc.
Yao, L., Mao, C., and Luo, Y. (2019). Graph con-
volutional networks for text classification. In The
Thirty-Third AAAI Conference on Artificial Intelli-
gence, AAAI 2019, The Thirty-First Innovative Ap-
plications of Artificial Intelligence Conference, IAAI
2019, The Ninth AAAI Symposium on Educational Ad-
vances in Artificial Intelligence, EAAI 2019, pages
7370–7377.
Yu, Z., Yu, J., Cui, Y., Tao, D., and Tian, Q. (2019). Deep
modular co-attention networks for visual question an-
swering. In Proceedings of the IEEE Conference on
Computer Vision and Pattern Recognition (CVPR),
pages 6281–6290.
Object-less Vision-language Model on Visual Question Classification for Blind People
187