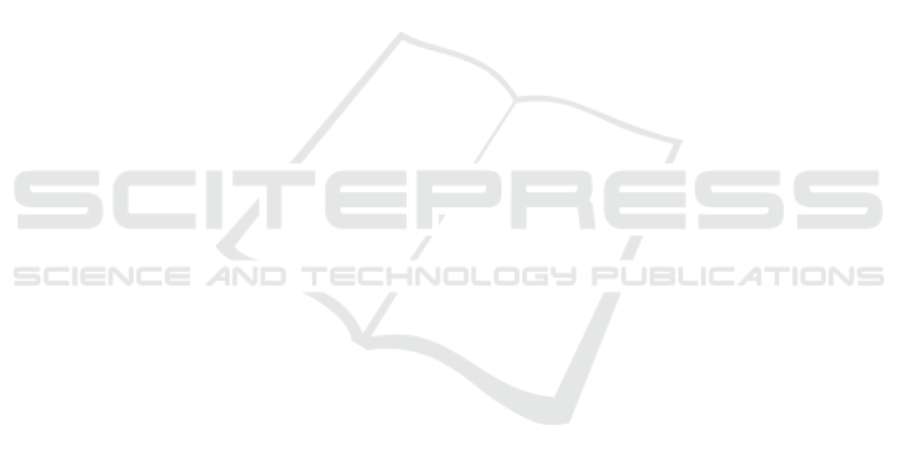
emotional dialogue generation (Liu et al., 2021; As-
ghar et al., 2020; Zhou and Wang, 2018) as well as
topical dialogue generation (Wang et al., 2020).
5.2 Personalized Dialogue
Over the past few years, there has been numerous
publications exploring various approaches to the task
of personalized dialogue generation. As mentioned
in the introduction, there are numerous approaches
to the task of personalized dialogue generation. A
popular approach typically involves conditioning di-
alogue responses on the dialogue context in addition
to textual persona descriptions (Lee et al., 2021; Na
et al., 2021; Liu et al., 2020; Majumder et al., 2020;
Madotto et al., 2019; Wolf et al., 2019; Zheng et al.,
2020; Chan et al., 2019; Song et al., 2019). This
approach focuses on generating responses which in-
corporate the provided persona information. Another
approach involves incorporating personality or per-
sona related metadata into the decoding process (Qian
et al., 2018). Some approaches also involve implicitly
learning personality user embeddings (Zheng et al.,
2019a; Wu et al., 2020; Al-Rfou et al., 2016; Li et al.,
2016). Another approach entails inferring the dia-
logue agent’s personality directly from the dialogue
history (Zheng et al., 2019b; Su et al., 2019). Typi-
cally, for this approach, the primary aim is to train the
agent to mimic the dialogue style of the interlocutor.
6 CONCLUSION
In this paper, we introduced DLVGen, a dual latent
variable model which models the potential responses
and the agent’s potential persona as latent Gaussian
distributions. Through our experiments, we find that
responses generated by DLVGen effectively incor-
porates persona information inferred from the dia-
logue context. We also introduced a variance reg-
ularization technique and lexical diversity selection
method which improves the quality of the generated
responses in terms of both persona consistency and
human-likeness. However, an area for improvement
is the relatively poor engagingness of the dialogue.
Encouraging the generation of persona consistent, di-
verse yet engaging open-domain dialogue is a poten-
tial avenue for future research. A possible approach
involves designing an objetcive function which ex-
plicitly accounts for the engagingness of the gener-
ated response. The dialogue model could then be
trained on both objective functions via a multi-task
learning framework.
REFERENCES
Al-Rfou, R., Pickett, M., Snaider, J., Sung, Y., Strope, B.,
and Kurzweil, R. (2016). Conversational contextual
cues: The case of personalization and history for re-
sponse ranking. CoRR, abs/1606.00372.
Asghar, N., Kobyzev, I., Hoey, J., Poupart, P., and Sheikh,
M. B. (2020). Generating emotionally aligned re-
sponses in dialogues using affect control theory.
Chan, Z., Li, J., Yang, X., Chen, X., Hu, W., Zhao, D.,
and Yan, R. (2019). Modeling personalization in con-
tinuous space for response generation via augmented
Wasserstein autoencoders. In Proceedings of the 2019
Conference on Empirical Methods in Natural Lan-
guage Processing and the 9th International Joint Con-
ference on Natural Language Processing (EMNLP-
IJCNLP), pages 1931–1940, Hong Kong, China. As-
sociation for Computational Linguistics.
Dinan, E., Logacheva, V., Malykh, V., Miller, A., Shus-
ter, K., Urbanek, J., Kiela, D., Szlam, A., Serban,
I., Lowe, R., Prabhumoye, S., Black, A. W., Rud-
nicky, A., Williams, J., Pineau, J., Burtsev, M., and
Weston, J. (2019). The second conversational intelli-
gence challenge (convai2).
Fergadiotis, G., Wright, H. H., and Green, S. B. (2015).
Psychometric evaluation of lexical diversity indices:
Assessing length effects. Journal of Speech, Lan-
guage, and Hearing Research, 58(3):840–852.
Kingma, D. P. and Welling, M. (2014). Auto-encoding vari-
ational bayes.
Lee, J. Y., Lee, K. A., and Gan, W. S. (2021). Generating
personalized dialogue via multi-task meta-learning. In
Proceedings of the 25th Workshop on the Semantics
and Pragmatics of Dialogue - Full Papers, Potsdam,
Germany. SEMDIAL.
Li, C., Gao, X., Li, Y., Peng, B., Li, X., Zhang, Y., and
Gao, J. (2020). Optimus: Organizing sentences via
pre-trained modeling of a latent space. In Proceed-
ings of the 2020 Conference on Empirical Methods in
Natural Language Processing (EMNLP), pages 4678–
4699, Online. Association for Computational Linguis-
tics.
Li, J., Galley, M., Brockett, C., Spithourakis, G., Gao, J.,
and Dolan, B. (2016). A persona-based neural conver-
sation model. In Proceedings of the 54th Annual Meet-
ing of the Association for Computational Linguistics
(Volume 1: Long Papers), pages 994–1003, Berlin,
Germany. Association for Computational Linguistics.
Liu, M., Bao, X., Liu, J., Zhao, P., and Shen, Y. (2021).
Generating emotional response by conditional varia-
tional auto-encoder in open-domain dialogue system.
Neurocomputing, 460:106–116.
Liu, Q., Chen, Y., Chen, B., Lou, J.-G., Chen, Z., Zhou,
B., and Zhang, D. (2020). You impress me: Dialogue
generation via mutual persona perception. In Proceed-
ings of the 58th Annual Meeting of the Association for
Computational Linguistics, pages 1417–1427, Online.
Association for Computational Linguistics.
Madotto, A., Lin, Z., Wu, C.-S., and Fung, P. (2019). Per-
sonalizing dialogue agents via meta-learning. In Pro-
DLVGen: A Dual Latent Variable Approach to Personalized Dialogue Generation
201