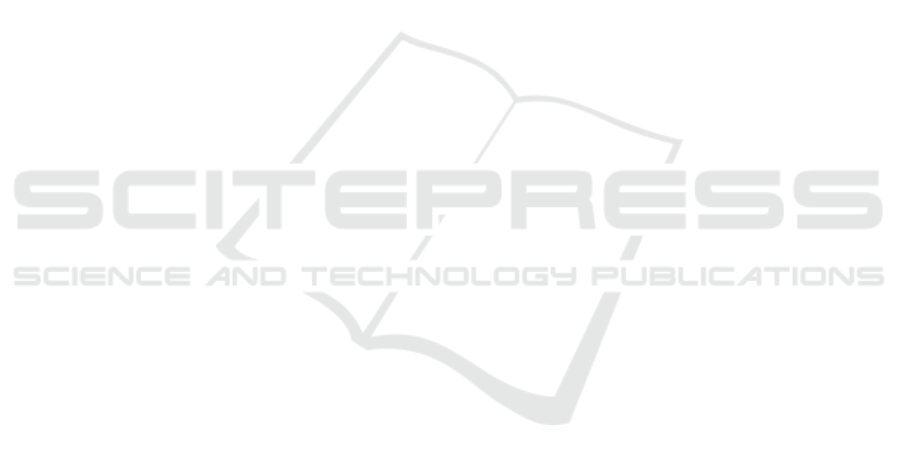
ACKNOWLEDGEMENTS
Our work has been supported in part by JSPS
KAKENHI Grant-in-Aid for Scientific Research (C)
17K00361, and (C) 20K12008.
We would like to express our sincere gratitude to
Kitagawa Hirofumi, Takahashi Hisashi, Matsuno Ed-
uardo, Takamura Ryota, and Takahashi Yasutake, for
development of eye-in-hand system, which helped us
to focus on our VDD project.
REFERENCES
Cha, Y.-J., Choi, W., Suh, G., Mahmoudkhani, S., and
B
¨
uy
¨
uk
¨
ozt
¨
urk, O. (2018). Autonomous structural vi-
sual inspection using region-based deep learning for
detecting multiple damage types. Computer-Aided
Civil and Infrastructure Engineering, 33(9):731–747.
Chin, R. T. and Harlow, C. A. (1982). Automated visual
inspection: A survey. IEEE transactions on pattern
analysis and machine intelligence, (6):557–573.
Czimmermann, T., Ciuti, G., Milazzo, M., Chiurazzi, M.,
Roccella, S., Oddo, C. M., and Dario, P. (2020).
Visual-based defect detection and classification ap-
proaches for industrial applications-a survey. Sensors,
20(5):1459.
Elsken, T., Metzen, J. H., Hutter, F., et al. (2019). Neural
architecture search: A survey. J. Mach. Learn. Res.,
20(55):1–21.
Ghiasi, G., Lin, T.-Y., and Le, Q. V. (2019). Nas-fpn:
Learning scalable feature pyramid architecture for ob-
ject detection. In Proceedings of the IEEE/CVF Con-
ference on Computer Vision and Pattern Recognition,
pages 7036–7045.
Huang, S.-H. and Pan, Y.-C. (2015). Automated visual
inspection in the semiconductor industry: A survey.
Computers in industry, 66:1–10.
Jiao, L., Zhang, F., Liu, F., Yang, S., Li, L., Feng, Z., and
Qu, R. (2019). A survey of deep learning-based object
detection. IEEE Access, 7:128837–128868.
Kumar, A. (2008). Computer-vision-based fabric defect de-
tection: A survey. IEEE transactions on industrial
electronics, 55(1):348–363.
Lin, T.-Y., Doll
´
ar, P., Girshick, R., He, K., Hariharan, B.,
and Belongie, S. (2017). Feature pyramid networks
for object detection. In Proceedings of the IEEE con-
ference on computer vision and pattern recognition,
pages 2117–2125.
Liu, L., Ouyang, W., Wang, X., Fieguth, P., Chen, J., Liu,
X., and Pietik
¨
ainen, M. (2020). Deep learning for
generic object detection: A survey. International jour-
nal of computer vision, 128(2):261–318.
Luo, Q., Fang, X., Liu, L., Yang, C., and Sun, Y. (2020).
Automated visual defect detection for flat steel sur-
face: A survey. IEEE Transactions on Instrumentation
and Measurement, 69(3):626–644.
Natarajan, V., Hung, T.-Y., Vaikundam, S., and Chia, L.-
T. (2017). Convolutional networks for voting-based
anomaly classification in metal surface inspection. In
2017 IEEE International Conference on Industrial
Technology (ICIT), pages 986–991. IEEE.
Neogi, N., Mohanta, D. K., and Dutta, P. K. (2014). Re-
view of vision-based steel surface inspection systems.
EURASIP Journal on Image and Video Processing,
2014(1):1–19.
Newman, T. S. and Jain, A. K. (1995). A survey of auto-
mated visual inspection. Computer vision and image
understanding, 61(2):231–262.
Russell, B. C., Torralba, A., Murphy, K. P., and Freeman,
W. T. (2008). Labelme: a database and web-based
tool for image annotation. International journal of
computer vision, 77(1-3):157–173.
Tumer, I. and Bajwa, A. (1999). A survey of aircraft engine
health monitoring systems. In 35th joint propulsion
conference and exhibit, page 2528.
Xie, X. (2008). A review of recent advances in surface de-
fect detection using texture analysis techniques. EL-
CVIA: electronic letters on computer vision and image
analysis, pages 1–22.
Zhao, Z., Qi, H., Qi, Y., Zhang, K., Zhai, Y., and Zhao,
W. (2020). Detection method based on automatic vi-
sual shape clustering for pin-missing defect in trans-
mission lines. IEEE Transactions on Instrumentation
and Measurement, 69(9):6080–6091.
Zou, Z., Shi, Z., Guo, Y., and Ye, J. (2019). Object detection
in 20 years: A survey. CoRR, abs/1905.05055.
ICPRAM 2022 - 11th International Conference on Pattern Recognition Applications and Methods
400