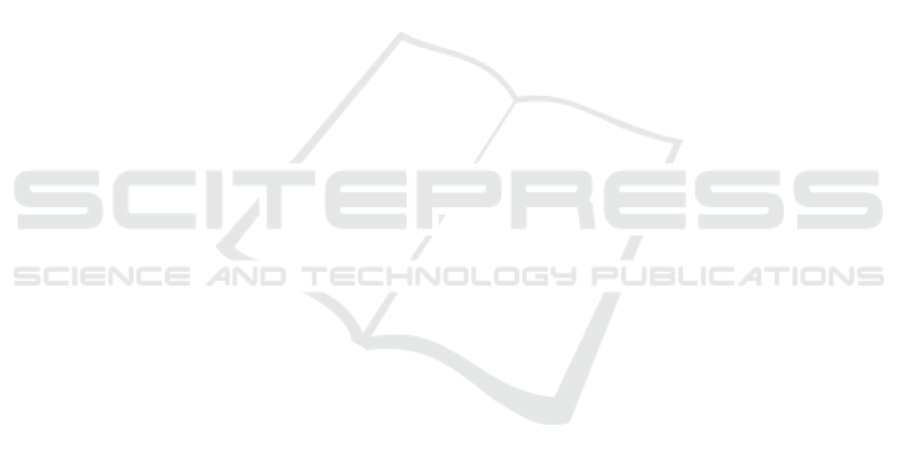
REFERENCES
ASAM (2021a). Asam opendrive. https://www.asam.net/
standards/detail/opendrive/. Accessed: 2021-08-10.
ASAM (2021b). Asam openscenario. https://www.asam.
net/standards/detail/openscenario/. Accessed: 2021-
08-10.
ASAM (2021c). Asam openscenario 2.0. https://www.
asam.net/project-detail/asam-openscenario-v20-1/.
Accessed: 2021-09-08.
Atorf, L. and Roßmann, J. (2018). Interactive analysis and
visualization of digital twins in high-dimensional state
spaces. In 2018 15th International Conference on
Control, Automation, Robotics and Vision (ICARCV),
pages 241–246. IEEE.
Bagschik, G., Menzel, T., and Maurer, M. (2018). Ontol-
ogy based scene creation for the development of au-
tomated vehicles. In 2018 IEEE Intelligent Vehicles
Symposium (IV), pages 1813–1820.
Ben Abdessalem, R., Nejati, S., Briand, L. C., and Stifter,
T. (2016). Testing advanced driver assistance systems
using multi-objective search and neural networks. In
2016 31st IEEE/ACM International Conference on
Automated Software Engineering (ASE), pages 63–74.
Feng, S., Feng, Y., Yu, C., Zhang, Y., and Liu, H. X.
(2021). Testing scenario library generation for con-
nected and automated vehicles, part i: Methodology.
IEEE Transactions on Intelligent Transportation Sys-
tems, 22(3):1573–1582.
Fremont, D. J., Dreossi, T., Ghosh, S., Yue, X.,
Sangiovanni-Vincentelli, A. L., and Seshia, S. A.
(2019). Scenic: A language for scenario specifica-
tion and scene generation. In Proceedings of the 40th
ACM SIGPLAN Conference on Programming Lan-
guage Design and Implementation, PLDI 2019, page
63–78, New York, NY, USA. Association for Comput-
ing Machinery.
Fremont, D. J., Kim, E., Pant, Y. V., Seshia, S. A., Acharya,
A., Bruso, X., Wells, P., Lemke, S., Lu, Q., and
Mehta, S. (2020). Formal scenario-based testing of
autonomous vehicles: From simulation to the real
world. In 2020 IEEE 23rd International Conference
on Intelligent Transportation Systems (ITSC), pages
1–8.
Goodfellow, I., Pouget-Abadie, J., Mirza, M., Xu, B.,
Warde-Farley, D., Ozair, S., Courville, A., and Ben-
gio, Y. (2014). Generative adversarial nets. Advances
in neural information processing systems, 27.
Hartjen, L., Philipp, R., Schuldt, F., Friedrich, B., and
Howar, F. (2019). Classification of driving maneuvers
in urban traffic for parametrization of test scenarios.
In 9. Tagung Automatisiertes Fahren.
Jiang, C., Qi, S., Zhu, Y., Huang, S., Lin, J., Yu, L.-F., Ter-
zopoulos, D., and Zhu, S.-C. (2018). Configurable 3d
scene synthesis and 2d image rendering with per-pixel
ground truth using stochastic grammars. International
Journal of Computer Vision, 126(9):920–941.
Khirodkar, R., Yoo, D., and Kitani, K. (2019). Domain ran-
domization for scene-specific car detection and pose
estimation. In 2019 IEEE Winter Conference on Ap-
plications of Computer Vision (WACV), pages 1932–
1940.
Menzel, T., Bagschik, G., and Maurer, M. (2018). Scenarios
for development, test and validation of automated ve-
hicles. In 2018 IEEE Intelligent Vehicles Symposium
(IV), pages 1821–1827. IEEE.
Roberts, G. O. (1996). Markov chain concepts related to
sampling algorithms. Markov chain Monte Carlo in
practice, 57:45–58.
Rossmann, J., Schluse, M., Schlette, C., Waspe, R.,
Van Impe, J., and Logist, F. (2013). A new approach
to 3d simulation technology as enabling technology
for erobotics. In 1st International Simulation Tools
Conference & EXPO, pages 39–46.
Sippl, C., Bock, F., Lauer, C., Heinz, A., Neumayer, T., and
German, R. (2019). Scenario-based systems engineer-
ing: An approach towards automated driving function
development. In 2019 IEEE International Systems
Conference (SysCon), pages 1–8.
Smith, A. F. and Roberts, G. O. (1993). Bayesian compu-
tation via the gibbs sampler and related markov chain
monte carlo methods. Journal of the Royal Statistical
Society: Series B (Methodological), 55(1):3–23.
Tobin, J., Fong, R., Ray, A., Schneider, J., Zaremba, W., and
Abbeel, P. (2017). Domain randomization for transfer-
ring deep neural networks from simulation to the real
world. In 2017 IEEE/RSJ International Conference on
Intelligent Robots and Systems (IROS), pages 23–30.
Ulbrich, S., Menzel, T., Reschka, A., Schuldt, F., and Mau-
rer, M. (2015). Defining and substantiating the terms
scene, situation, and scenario for automated driving.
In 2015 IEEE 18th International Conference on Intel-
ligent Transportation Systems, pages 982–988. IEEE.
Van den Meersche, K., Soetaert, K., and Van Oevelen, D.
(2009). xsample (): an r function for sampling lin-
ear inverse problems. Journal of Statistical Software,
30(Code Snippet 1).
Wachenfeld, W. and Winner, H. (2016). The release of
autonomous vehicles. In Autonomous driving, pages
425–449. Springer.
Wagner, S., Groh, K., Kuhbeck, T., Dorfel, M., and Knoll,
A. (2018). Using time-to-react based on naturalistic
traffic object behavior for scenario-based risk assess-
ment of automated driving. In 2018 IEEE Intelligent
Vehicles Symposium (IV), pages 1521–1528. IEEE.
Winner, H., Lemmer, K., Form, T., and Mazzega, J. (2019).
Pegasus—first steps for the safe introduction of auto-
mated driving. In Road Vehicle Automation 5, pages
185–195. Springer.
MODELSWARD 2022 - 10th International Conference on Model-Driven Engineering and Software Development
210