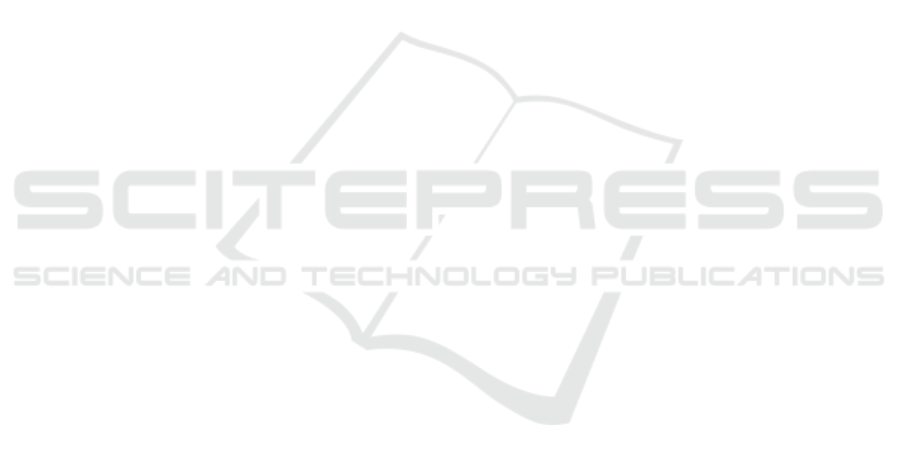
an essential part of the process. Studies have found
that in a majority of the cases, data is not in the de-
sired condition, and measurements mixed with vari-
ous kinds of errors are generated by the meters.
This paper was focused on the progressive clean-
ing of data while analyzing the impact of data errors
on the performance of a specific filter, namely, peak
consumer identification and SFRES consumption pro-
files. During the progressive cleaning process, vari-
ous sources of errors, such as mistakes made by op-
erators, hardware failures, and context-dependent er-
rors, were identified. In addition, systematic ways
of removing the main contributing errors (meter unit
inconsistencies, the meter resets, spikes, duplicated
records, and duplicated datastreams) were provided
and more complex errors were characterized, as well.
The results of cleaning data and application of
the filter (performing peak detection tasks) were pre-
sented, and the cleaning process’s significance was
demonstrated. Also, the sensitivity of the outputs to
the errors in the data and the parameters of the peak
detection filter was examined.
To conclude, data cleaning is an essential part of
big data application in smart meter measurement anal-
ysis. However, prior knowledge of the state of data
quality and the sensitivity of the results to different
types of error is required. Smart meter data analysis
is still in its early stages and can benefit considerably
from further research. Some possible extensions of
the work were presented in this paper. The data qual-
ity should be evaluated using other physical charac-
teristics of the water supply infrastructure, assuming
feasibility of acquiring them, such as pressure infor-
mation of various key nodes, mass balancing of the
consumption and production, using bulk meter data of
the network. Many possible errors in the datastreams
have been detected in this work; however, other filters
can detect other potential errors. Examples of such
filters can be: “does the hourly consumption profile
of different customer categories follow the expected
minimum and maximum load?”
The other extension is to examine the effect of
quantized meters on data quality and devise clean-
ing methods that can deal with such error types more
effectively. In addition, missing data points, an in-
evitable aspect of every smart system, were analyzed,
compensating their effects. As a future project, sim-
ilar to the procedure performed for errors in this pa-
per, missing data can be characterized more system-
atically.
REFERENCES
Aijun, A., Ning, S., Chan, C., Cercone, N., and Ziarko, W.
(1996). Discovering rules for water demand predic-
tion: An enhanced rough-set approach. Engineering
Applications of Artificial Intelligence, 9:645–653.
Alquthami, T., Alsubaie, A., and Anwer, M. (2019). Impor-
tance of smart meters data processing – case of saudi
arabia. In 2019 International Conference on Elec-
trical and Computing Technologies and Applications
(ICECTA), pages 1–5.
Arregui, Francisco and Cabrera, E and Cobacho, Ricardo
and Garc
´
ıa-Serra, Jorge (2005). Key factors affecting
water meter accuracy. In Leakage 2005, pages 1–10,
Portugal. Leakage 2005.
Avni, N., Fishbain, B., and Shamir, U. (2015). Water con-
sumption patterns as a basis for water demand model-
ing. Water Resources Research, 51(10):8165–8181.
Beal, C., Stewart, R. A., Huang, T., and Rey, E. (2011).
SEQ residential end use study. Australian Water As-
sociation, 38(1):80–84.
Beal, Cara and Stewart, Rodney A. and Huang, T. and Rey,
E. (2011). South East Queensland residential end use
study: Final Report. Journal of the Australian Water
Association, 38(1):80–84.
Chen, J., Chen, Y., Du, X., Li, C., Lu, J., Zhao, S., and
Zhou, X. (2013). Big data challenge: a data man-
agement perspective. Frontiers of computer Science,
7(2):157–164.
Courtney, M. (2014). How utilities are profiting from Big
Data analytics. Engineering and Technology Maga-
zine.
D’Alberto, P. and Dasdan, A. (2010). On the Weakenesses
of Correlation Measures used for Search Engines’ Re-
sults. Cornell University Library. Access on: 2014-
12-15.
Farhangi, H. (2010). The path of the smart grid. IEEE
power and energy magazine, 8(1).
Fielding, K. S., Spinks, A., Russell, S., McCrea, R., Stew-
art, R. A., and Gardner, J. (2013). An experimental
test of voluntary strategies to promote urban water de-
mand management. Journal of Environmental Man-
agement, 114(0):343–351.
Heinrich, Matthias (2007). Water End Use and Efficiency
Project (WEEP): Final Report. Technical report,
BRANZ Ltd., Judgeford, New Zealand. BRANZ
Study Report 159.
Hsia, S.C. and Hsu, S.W. and Chang, Y.J. (2012). Remote
monitoring and smart sensing for water meter system
and leakage detection. IET Wireless sensor systems,
2(4):402–408.
Jia, L. and Kim, J. and Thomas, R.J. and Tong, L.
(2014). Impact of data quality on real-time locational
marginal price. IEEE Transactions on Power Systems,
29(2):627–636.
Kaisler, Stephen and Armour, Frank and Espinosa, J Al-
berto and Money, William (2013). Big data: Issues
and challenges moving forward. In System Sciences
(HICSS), 46th International Conference on, pages
995–1004, Hawaii. IEEE.
ICAART 2022 - 14th International Conference on Agents and Artificial Intelligence
442