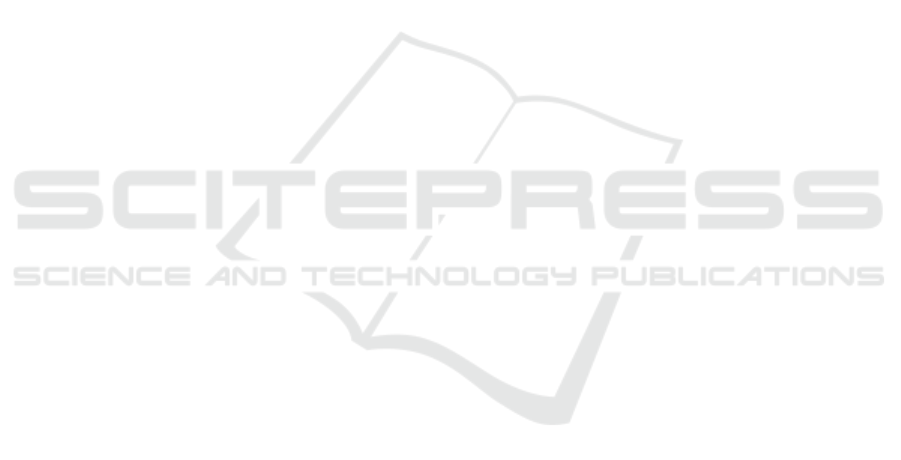
6 CONCLUSION AND FUTURE
WORK
The effect of using trajectories over raw sensor data
in unsupervised classification for HAR is striking.
Clustering algorithms using either trajectory time se-
ries or trajectory images outperform the sensor-based
variants. The potential power of unsupervised clas-
sification in activity recognition for videos was al-
ready indicated by (Niebles et al., 2008). The ap-
proaches used in such methods could be powerful
tools for trajectory image clustering and should be
explored further. Slightly different sensor setups or
using sensors from a different manufacturer can be
achieved through transfer learning from the origi-
nal synthetic Archive of Motion Capture as Surface
Shapes (AMASS) dataset(Mahmood et al., 2019).
Furthermore, euclidean distance in high-dimensional
space should be mitigated, for example, by using L
norms (Aggarwal et al., 2001).
REFERENCES
Abedin, A., Motlagh, F., Shi, Q., Rezatofighi, H., and
Ranasinghe, D. (2020). Towards Deep Clustering of
Human Activities from Wearables. In Proceedings of
the 2020 International Symposium on Wearable Com-
puters, ISWC ’20, page 1–6, New York, NY, USA.
Association for Computing Machinery.
Aggarwal, C. C., Hinneburg, A., and Keim, D. A. (2001).
On the Surprising Behavior of Distance Metrics in
High Dimensional Space. In Van den Bussche, J. and
Vianu, V., editors, Database Theory — ICDT 2001,
pages 420–434, Berlin, Heidelberg. Springer Berlin
Heidelberg.
Ariza Colpas, P., Vicario, E., De-La-Hoz-Franco, E.,
Pineres-Melo, M., Oviedo-Carrascal, A., and Patara,
F. (2020). Unsupervised Human Activity Recognition
Using the Clustering Approach: A Review. Sensors,
20(9).
Bai, L., Yeung, C., Efstratiou, C., and Chikomo, M. (2019).
Motion2Vector: Unsupervised Learning in Human
Activity Recognition Using Wrist-Sensing Data. In
Adjunct Proceedings of the 2019 ACM International
Joint Conference on Pervasive and Ubiquitous Com-
puting and Proceedings of the 2019 ACM Inter-
national Symposium on Wearable Computers, Ubi-
Comp/ISWC ’19 Adjunct, page 537–542, New York,
NY, USA. Association for Computing Machinery.
Bishop, C. M. (2007). Pattern Recognition and Ma-
chine Learning (Information Science and Statistics).
Springer, 1 edition.
Chen, K., Zhang, D., Yao, L., Guo, B., Yu, Z., and Liu,
Y. (2021). Deep Learning for Sensor-Based Human
Activity Recognition: Overview, Challenges, and Op-
portunities. ACM Comput. Surv., 54(4).
Ester, M., Kriegel, H.-P., Sander, J., and Xu, X. (1996). A
Density-Based Algorithm for Discovering Clusters in
Large Spatial Databases with Noise. In Proceedings
of the Second International Conference on Knowledge
Discovery and Data Mining, KDD’96, pages 226–
231. AAAI Press.
Huang, Y., Kaufmann, M., et al. (2018). Deep Iner-
tial Poser: Learning to Reconstruct Human Pose
from Sparse Inertial Measurements in Real Time.
ACM Transactions on Graphics, (Proc. SIGGRAPH
Asia), 37:185:1–185:15. Two first authors contributed
equally.
Ji, X., Henriques, J. F., and Vedaldi, A. (2019). Invariant
Information Clustering for Unsupervised Image Clas-
sification and Segmentation. In Proceedings of the
IEEE International Conference on Computer Vision
(ICCV), pages 9865–9874.
Konak, O., Wegner, P., and Arnrich, B. (2020). IMU-Based
Movement Trajectory Heatmaps for Human Activity
Recognition. Sensors, 20(24).
Li, F., Qiao, H., and Zhang, B. (2018). Discriminatively
Boosted Image Clustering with Fully Convolutional
Auto-Encoders. Pattern Recognition, 83:161–173.
Lloyd, S. (2019). Least Square Quantization in PCM. In
Proceedings of the IEEE Transactions on Information
Theory, volume 28, pages 129–137.
Loper, M., Mahmood, N., Romero, J., Pons-Moll, G., and
Black, M. J. (2015). SMPL: A Skinned Multi-Person
Linear Model. ACM Trans. Graphics (Proc. SIG-
GRAPH Asia), 34(6):248:1–248:16.
Mahmood, N., Ghorbani, N., Troje, N. F., Pons-Moll, G.,
and Black, M. J. (2019). AMASS: Archive of Motion
Capture as Surface Shapes. In International Confer-
ence on Computer Vision, pages 5442–5451.
Min, E., Guo, X., Liu, Q., Zhang, G., Cui, J., and Long, J.
(2018). A Survey of Clustering With Deep Learning:
From the Perspective of Network Architecture. IEEE
Access, 6:39501–39514.
M
¨
uller, M. (2007). Dynamic Time Warping. Information
Retrieval for Music and Motion, 2:69–84.
Niebles, J., Wang, H., and Fei-Fei, L. (2008). Unsupervised
Learning of Human Action Categories Using Spatial-
Temporal Words. International Journal for Computer
Vision, 3(79):299–318.
Pearson, K. (1901). On Lines and Planes of Closest Fit to
Systems of Points in Space. Philosophical Magazine,
2:559–572.
Sheng, T. and Huber, M. (2020). Unsupervised Embed-
ding Learning for Human Activity Recognition Using
Wearable Sensor Data.
Vinh, N. X., Epps, J., and Bailey, J. (2009). Information
Theoretic Measures for Clusterings Comparison: Is
a Correction for Chance Necessary? In Proceed-
ings of the 26th Annual International Conference on
Machine Learning, ICML ’09, page 1073–1080, New
York, NY, USA. Association for Computing Machin-
ery.
Wang, Y., Yao, H., and Zhao, S. (2015). Auto-Encoder
Based Dimensionality Reduction. Neurocomputing,
184.
Unsupervised Activity Recognition Using Trajectory Heatmaps from Inertial Measurement Unit Data
311