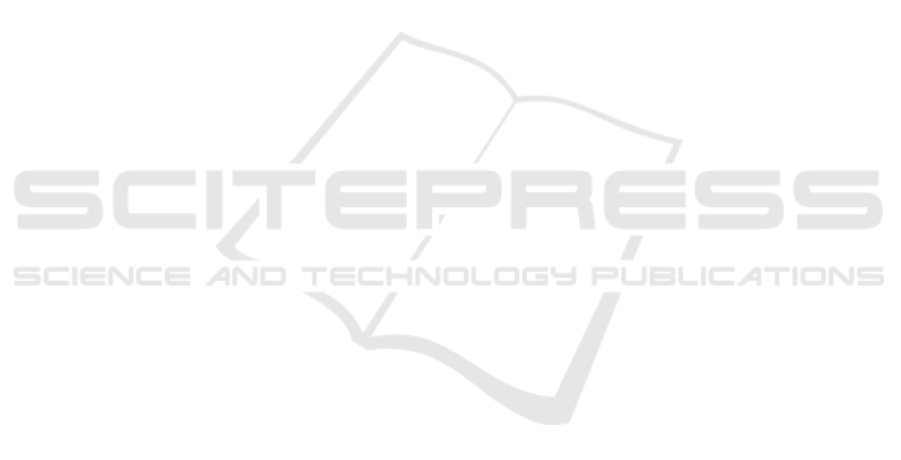
In this work, we evaluated patching images to
improve the results on the classification of lung X-
ray images into normal, pneumonia, and COVID-19
classes. Our classification model, which consists of
performing an ensemble of the expert models of each
patch, presented an accuracy of 90.67% in the test set,
allowing us to help in the task of classifying the X-ray
images between COVID-19, pneumonia, and normal.
The results obtained by our method surpassed the re-
sults achieved by another method in the literature ap-
plied on COVIDx, version 7.
As future works, the evaluation of more prepro-
cessing steps, as data augmentation, as well as dif-
ferent ensemble techniques, can help the model to
achieve better results.
ACKNOWLEDGEMENTS
This research was supported by s
˜
ao Paulo Research
Foundation (FAPESP) [grant numbers 2015/11937-
9 and 2017/12646-3] and the National Council for
Scientific and Technological Development (CNPq)
[grant numbers 161015/2021-2 and 304380/2018-0].
REFERENCES
Amyar, A., Modzelewski, R., Li, H., and Ruan, S. (2020).
Multi-task deep learning based CT imaging analysis
for COVID-19 pneumonia: Classification and seg-
mentation. Computers in Biology and Medicine,
126:104037.
Bai, H. X., Hsieh, B., Xiong, Z., Halsey, K., Choi, J. W.,
Tran, T. M. L., Pan, I., Shi, L.-B., Wang, D.-C., Mei,
J., Jiang, X.-L., Zeng, Q.-H., Egglin, T. K., Hu, P.-
F., Agarwal, S., Xie, F.-F., Li, S., Healey, T., Ata-
lay, M. K., and Liao, W.-H. (2020). Performance
of radiologists in differentiating COVID-19 from non-
COVID-19 viral pneumonia at chest CT. Radiology,
296(2):E46–E54.
Barstugan, M., Ozkaya, U., and Ozturk, S. (2020). Coro-
navirus (COVID-19) Classification using CT Images
by Machine Learning Methods. arXiv:2003.09424,
pages 1–10.
Chowdhury, M. E., Rahman, T., Khandakar, A., Mazhar,
R., Kadir, M. A., Mahbub, Z. B., Islam, K. R., Khan,
M. S., Iqbal, A., Al Emadi, N., Reaz, M. B. I., and
Islam, M. T. (2020). Can AI help in screening viral
and COVID-19 pneumonia? IEEE Access, 8:132665–
132676.
Coelho, F. C., Lana, R. M., Cruz, O. G., Codeco, C. T., Vil-
lela, D., Bastos, L. S., y Piontti, A. P., Davis, J. T.,
Vespignani, A., and Gomes, M. F. (2020). Assess-
ing the potential impacts of COVID-19 in Brasil: Mo-
bility, Morbidity and Impact to the Health System.
medRxiv, pages 1–14.
Cohen, J. P., Morrison, P., and Dao, L. (2020). COVID-19
Image Data Collection. arXiv:2003.11597, pages 1–4.
Dosovitskiy, A., Beyer, L., Kolesnikov, A., Weissenborn,
D., Zhai, X., Unterthiner, T., Dehghani, M., Min-
derer, M., Heigold, G., Gelly, S., and Uszkoreit, Jakob
abd Houlsby, N. (2020). An image is worth 16x16
words: Transformers for image recognition at scale.
arXiv:2010.11929, pages 1–22.
Dubey, S., Biswas, P., Ghosh, R., Chatterjee, S., Dubey,
M. J., Chatterjee, S., Lahiri, D., and Lavie, C. J.
(2020). Psychosocial impact of COVID-19. Dia-
betes & Metabolic Syndrome: Clinical Research &
Reviews, 14(5):779–788.
Feng, H., Liu, Y., Lv, M., and Zhong, J. (2020). A case
report of COVID-19 with false negative RT-PCR test:
necessity of chest CT. Japanese Journal of Radiology,
38(5):409–410.
Gibson, U. E., Heid, C. A., and Williams, P. M. (1996).
A novel method for real time quantitative RT-PCR.
Genome Research, 6(10):995–1001.
He, K., Zhang, X., Ren, S., and Sun, J. (2016). Deep
residual learning for image recognition. In Conference
on Computer Vision and Pattern Recognition (CVPR),
pages 770–778. IEEE.
Krizhevsky, A., Sutskever, I., and Hinton, G. E. (2012).
ImageNet classification with deep convolutional neu-
ral networks. In Advances in Neural Information
Processing Systems (NIPS), pages 1097–1105. Curran
Associates, Inc.
Oliveira, G., Padilha, R., Dorte, A., Cereda, L., Miyazaki,
L., Lopes, M., and Dias, Z. (2020). COVID-19 X-
ray Image Diagnostic with Deep Neural Networks. In
2020 Brazilian Symposium on Bioinformatics (BSB),
pages 57–68. Springer.
Ozili, P. K. and Arun, T. (2020). Spillover of COVID-19:
impact on the Global Economy. SSRN, pages 1–27.
Radiological Society of North America (2019). RSNA
pneumonia detection challenge. https://www.kaggle.
com/c/rsna-pneumonia-detection-challenge.
Rahman, T., Khandakar, A., Qiblawey, Y., Tahir, A., Ki-
ranyaz, S., Abul Kashem, S. B., Islam, M. T., Al
Maadeed, S., Zughaier, S. M., Khan, M. S., and
Chowdhury, M. E. (2021). Exploring the effect of im-
age enhancement techniques on COVID-19 detection
using chest X-ray images. Computers in Biology and
Medicine, 132:104319.
Ronneberger, O., Fischer, P., and Brox, T. (2015). U-
Net: Convolutional networks for biomedical image
segmentation. In 18th International Conference on
Medical Image Computing and Computer-Assisted In-
tervention (MICCAI), pages 234–241. Springer.
Sato, G. T. S., da Silva Segundo, L. B., and Dias, Z. (2020).
Classification of musculoskeletal abnormalities with
convolutional neural networks. In 2020 Brazilian
Symposium on Bioinformatics (BSB), pages 69–80.
Springer.
Selvaraju, R. R., Cogswell, M., Das, A., Vedantam, R.,
Parikh, D., and Batra, D. (2017). Grad-CAM: Vi-
sual explanations from deep networks via gradient-
ICAART 2022 - 14th International Conference on Agents and Artificial Intelligence
566