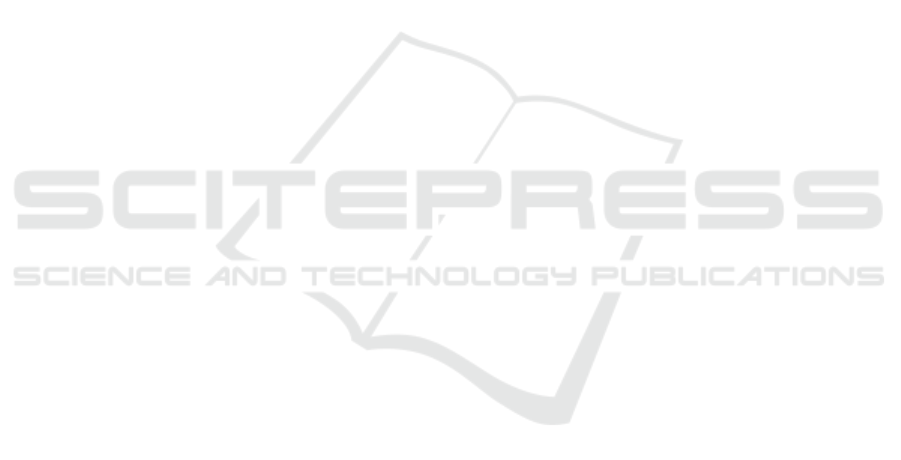
for privacy preserving data publishing: A comprehen-
sive survey. IEEE Access, PP:1–1.
Meyerson, A. and Williams, R. (2004). On the complex-
ity of optimal k-anonymity. Proceedings of the ACM
SIGACT-SIGMOD-SIGART Symposium on Principles
of Database Systems, 23.
Mohr, M., Becker, C., M
¨
oller, R., and Richter, M. (2021).
Towards collaborative predictive maintenance lever-
aging private cross-company data. In Reussner, R. H.,
Koziolek, A., and Heinrich, R., editors, INFOR-
MATIK 2020, pages 427–432. Gesellschaft f
¨
ur Infor-
matik, Bonn.
Narayanan, A. and Shmatikov, V. (2008). Robust de-
anonymization of large sparse datasets. In 2008 IEEE
Symposium on Security and Privacy (sp 2008), pages
111–125.
Nergiz, M. E., Atzori, M., and Clifton, C. (2007). Hiding
the presence of individuals from shared databases. In
Proceedings of the 2007 ACM SIGMOD International
Conference on Management of Data, SIGMOD ’07,
page 665–676, New York, NY, USA. Association for
Computing Machinery.
Ogburn, M., Turner, C., and Dahal, P. (2013). Homomor-
phic encryption. Procedia Computer Science, 20:502–
509. Complex Adaptive Systems.
Ohrimenko, O., Tople, S., and Tschiatschek, S. (2019).
Collaborative machine learning markets with data-
replication-robust payments.
Panahifar, F., Byrne, P., Salam, M., and Heavey, C.
(2018). Supply chain collaboration and firm’s perfor-
mance: The critical role of information sharing and
trust. Journal of Enterprise Information Management,
31:00–00.
Renaud, K., Volkamer, M., and Renkema-Padmos, A.
(2014). Why doesn’t jane protect her privacy? In
De Cristofaro, E. and Murdoch, S. J., editors, Pri-
vacy Enhancing Technologies, pages 244–262, Cham.
Springer International Publishing.
Sabt, M., Achemlal, M., and Bouabdallah, A. (2015).
Trusted execution environment: What it is, and what
it is not. In 2015 IEEE Trustcom/BigDataSE/ISPA,
volume 1, pages 57–64.
Salas, J. and Domingo-Ferrer, J. (2018). Some basics on
privacy techniques, anonymization and their big data
challenges. Mathematics in Computer Science, 12.
Saputra, Y. M., Hoang, D. T., Nguyen, D. N., Dutkiewicz,
E., Mueck, M. D., and Srikanteswara, S. (2019). En-
ergy demand prediction with federated learning for
electric vehicle networks.
Sarma, K. V., Harmon, S., Sanford, T., Roth, H. R., Xu, Z.,
Tetreault, J., Xu, D., Flores, M. G., Raman, A. G.,
Kulkarni, R., Wood, B. J., Choyke, P. L., Priester,
A. M., Marks, L. S., Raman, S. S., Enzmann, D.,
Turkbey, B., Speier, W., and Arnold, C. W. (2021).
Federated learning improves site performance in mul-
ticenter deep learning without data sharing. Jour-
nal of the American Medical Informatics Association,
28(6):1259–1264.
Schomakers, E.-M., Lidynia, C., and Ziefle, M. (2020). All
of me? users’ preferences for privacy-preserving data
markets and the importance of anonymity. Electronic
Markets, 30.
Shamir, A. (1979). How to share a secret. Commun. ACM,
22(11):612–613.
Sheller, M., Edwards, B., Reina, G., Martin, J., Pati, S.,
Kotrotsou, A., Milchenko, M., Xu, W., Marcus, D.,
Colen, R., and Bakas, S. (2020). Federated learning
in medicine: facilitating multi-institutional collabora-
tions without sharing patient data. Scientific Reports,
10.
Shepherd, C., Arfaoui, G., Gurulian, I., Lee, R. P., Markan-
tonakis, K., Akram, R. N., Sauveron, D., and Con-
chon, E. (2016). Secure and trusted execution: Past,
present, and future - a critical review in the context
of the internet of things and cyber-physical systems.
In 2016 IEEE Trustcom/BigDataSE/ISPA, pages 168–
177.
Stefanov, E., Dijk, M. V., Shi, E., Chan, T.-H. H., Fletcher,
C., Ren, L., Yu, X., and Devadas, S. (2018). Path
oram: An extremely simple oblivious ram protocol. J.
ACM, 65(4).
Sweeney, L. (2002). K-anonymity: A model for protect-
ing privacy. Int. J. Uncertain. Fuzziness Knowl.-Based
Syst., 10(5):557–570.
Torkzadehmahani, R., Nasirigerdeh, R., Blumenthal, D. B.,
Kacprowski, T., List, M., Matschinske, J., Sp
¨
ath, J.,
Wenke, N. K., Bihari, B., Frisch, T., Hartebrodt,
A., Hausschild, A.-C., Heider, D., Holzinger, A.,
H
¨
otzendorfer, W., Kastelitz, M., Mayer, R., Nogales,
C., Pustozerova, A., R
¨
ottger, R., Schmidt, H. H.
H. W., Schwalber, A., Tschohl, C., Wohner, A., and
Baumbach, J. (2020). Privacy-preserving artificial in-
telligence techniques in biomedicine.
Vaidya, J. and Clifton, C. (2003). Privacy-preserving k-
means clustering over vertically partitioned data. In
Proceedings of the Ninth ACM SIGKDD International
Conference on Knowledge Discovery and Data Min-
ing, KDD ’03, page 206–215, New York, NY, USA.
Association for Computing Machinery.
Woldaregay, A., Henriksen, A., Issom, D.-Z., Pfuhl, G.,
Sato, K., Richard, A., Lovis, C., Arsand, E., Rochat,
J., and Hartvigsen, G. (2020). User expectations and
willingness to share self-collected health data. Studies
in health technology and informatics, 270.
Wood, A., Altman, M., Bembenek, A., Bun, M., Gaboardi,
M., Honaker, J., Nissim, K., OBrien, D. R., Steinke,
T., and Vadhan, S. (2018). Differential privacy: A
primer for a non-technical audience. Vanderbilt Jour-
nal of Entertainment & Technology Law, 21(1):209–
275.
Zhang, J., Fang, Z., Zhang, Y., and Song, D. (2020). Zero
knowledge proofs for decision tree predictions and ac-
curacy. In Proceedings of the 2020 ACM SIGSAC
Conference on Computer and Communications Secu-
rity, CCS ’20, page 2039–2053, New York, NY, USA.
Association for Computing Machinery.
Zhou, J., Zhang, S., Lu, Q., Dai, W., Chen, M., Liu, X., Pirt-
tikangas, S., Shi, Y., Zhang, W., and Herrera-Viedma,
E. (2021). A survey on federated learning and its ap-
plications for accelerating industrial internet of things.
ICAART 2022 - 14th International Conference on Agents and Artificial Intelligence
588