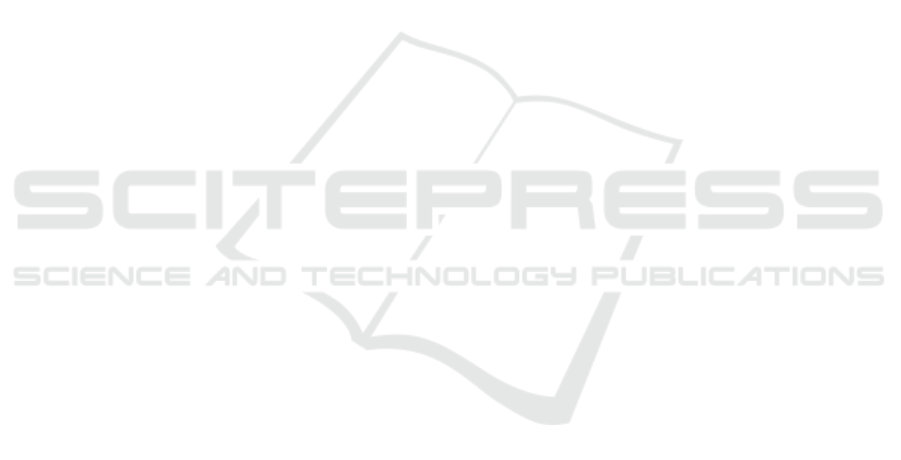
Raghavendra, R., Raja, K. B., Surbiryala, J., and Busch, C.
(2014). A low-cost multimodal biometric sensor to
capture finger vein and fingerprint. In IEEE Interna-
tional Joint Conference on Biometrics, pages 1–7.
Rebera, A. P. and Guihen, B. (2012). Biometrics for an age-
ing society societal and ethical factors in biometrics
and ageing. In 2012 BIOSIG - Proceedings of the In-
ternational Conference of Biometrics Special Interest
Group (BIOSIG), pages 1–4.
Rimar, D. (2020). Systemic Sclerosis in the Elderly, pages
207–228. Springer International Publishing, Cham.
Rodgers, J. L., Jones, J., Bolleddu, S. I., Vanthenapalli, S.,
Rodgers, L. E., Shah, K., Karia, K., and Panguluri,
S. K. (2019). Cardiovascular risks associated with
gender and aging. Journal of cardiovascular devel-
opment and disease, 6(2):19.
Rosdi, B. A., Shing, C. W., and Suandi, S. A. (2011). Finger
vein recognition using local line binary pattern. Sen-
sors, 11(12):11357–11371.
Shaheed, K., Liu, H., Yang, G., Qureshi, I., Gou, J., and
Yin, Y. (2018). A systematic review of finger vein
recognition techniques. Information, 9(9).
Tome, P., Raghavendra, R., Busch, C., Tirunagari, S., Poh,
N., Shekar, B. H., Gragnaniello, D., Sansone, C., Ver-
doliva, L., and Marcel, S. (2015). The 1st competition
on counter measures to finger vein spoofing attacks. In
2015 International Conference on Biometrics (ICB),
pages 513–518.
Tome, P., Vanoni, M., and Marcel, S. (2014). On the vul-
nerability of finger vein recognition to spoofing. In
2014 International Conference of the Biometrics Spe-
cial Interest Group (BIOSIG), pages 1–10.
Ton, B. T. and Veldhuis, R. N. J. (2013). A high quality
finger vascular pattern dataset collected using a cus-
tom designed capturing device. In 2013 International
Conference on Biometrics (ICB), pages 1–5.
United Nations (2019). World population prospects 2019.
https://population.un.org/wpp/Download/Standard/
Population/.
University of Pittsburgh (2020). Why do
older people heal more slowly? https:
//www.pitt.edu/pittwire/features-articles/
why-do-older-people-heal-more-slowly.
University of Reading (2017). Protect multimodal dataset.
http://projectprotect.eu/dataset/.
Vanoni, M., Tome, P., El Shafey, L., and Marcel, S. (2014).
Cross-database evaluation using an open finger vein
sensor. In 2014 IEEE Workshop on Biometric Mea-
surements and Systems for Security and Medical Ap-
plications (BIOMS) Proceedings, pages 30–35.
Wagh, D. P., Fadewar, H. S., and Shinde, G. N. (2020). Bio-
metric finger vein recognition methods for authentica-
tion. In Iyer, B., Deshpande, P. S., Sharma, S. C., and
Shiurkar, U., editors, Computing in Engineering and
Technology, pages 45–53, Singapore. Springer Singa-
pore.
Wang, K., Ma, H., Popoola, O., and Liu, J. (2011). Finger
vein recognition.
Wenming, Y., Huang, X., Zhou, F., and Liao, Q. (2014).
Fusion of finger vein and finger dorsal texture for per-
sonal identification based on comparative competitive
coding. Information Sciences, 268:20–32.
Xi, X., Yang, G., Yin, Y., and Meng, X. (2013). Finger
vein recognition with personalized feature selection.
Sensors, 13(9):11243–11259.
Yang, G., Xi, X., and Yin, Y. (2012). Finger vein recog-
nition based on a personalized best bit map. Sensors,
12(2):1738–1757.
Yang, J. and Shi, Y. (2014). Towards finger-vein image
restoration and enhancement for finger-vein recogni-
tion. Information sciences, 268:33–52.
Yang, L., Yang, G., Yin, Y., and Xi, X. (2018). Finger vein
recognition with anatomy structure analysis. IEEE
Transactions on Circuits and Systems for Video Tech-
nology, 28(8):1892–1905.
Yang, L., Yang, G., Yin, Y., and Zhou, L. (2014a). A survey
of finger vein recognition. In Sun, Z., Shan, S., Sang,
H., Zhou, J., Wang, Y., and Yuan, W., editors, Bio-
metric Recognition, pages 234–243, Cham. Springer
International Publishing.
Yang, W., Huang, X., Zhou, F., and Liao, Q. (2014b). Com-
parative competitive coding for personal identification
by using finger vein and finger dorsal texture fusion.
Information sciences, 268:20–32.
Yin, Y., Liu, L., and Sun, X. (2011). Sdumla-hmt: A mul-
timodal biometric database. In Sun, Z., Lai, J., Chen,
X., and Tan, T., editors, Biometric Recognition, pages
260–268, Berlin, Heidelberg. Springer Berlin Heidel-
berg.
Y
¨
uksel, A., Akarun, L., and Sankur, B. (2010). Biomet-
ric identification through hand vein patterns. In 2010
International Workshop on Emerging Techniques and
Challenges for Hand-Based Biometrics, pages 1–6.
Zhang, C., Li, X., Liu, Z., Zhao, Q., Xu, H., and Su, F.
(2013). The cfvd reflection-type finger-vein image
database with evaluation baseline. In Sun, Z., Shan,
S., Yang, G., Zhou, J., Wang, Y., and Yin, Y., edi-
tors, Biometric Recognition, pages 282–287, Cham.
Springer International Publishing.
Age Bias in Finger Vein Biometric Research
477