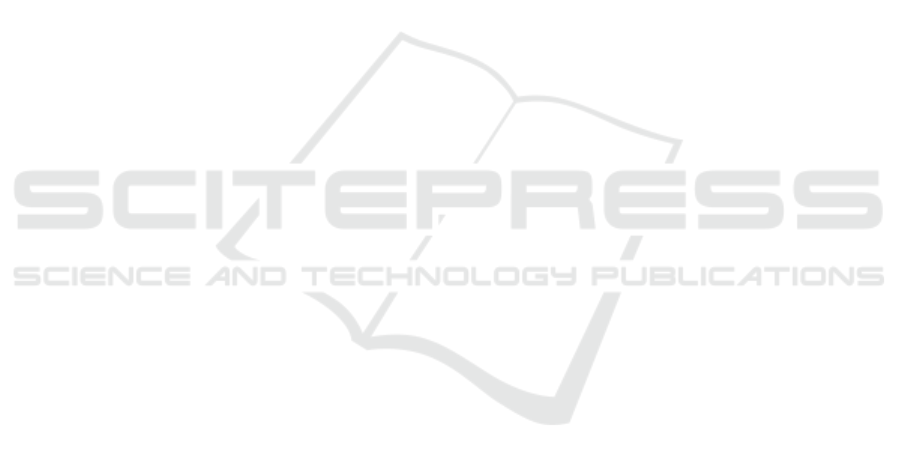
ducing prototypes of practical applications.
Future work for monocular depth video applica-
tions could address problems like flickering frames
and scale variance in a real-time manner. Addition-
ally, it would be interesting to add network function-
ality to predict infinite distance points and mask out
the sky. Another approach to be investigated would
be to see if training a network for gray-scale image
depth prediction would lead to better results with the
infrared-mode output at night.
REFERENCES
Baig, M. H. and Torresani, L. (2016). Coupled depth learn-
ing. In 2016 IEEE Winter Conference on Applications
of Computer Vision (WACV), pages 1–10.
Bhat, S. F., Alhashim, I., and Wonka, P. (2020). Ad-
abins: Depth estimation using adaptive bins. CoRR,
abs/2011.14141.
Casser, V., Pirk, S., Mahjourian, R., and Angelova,
A. (2018). Depth prediction without the sensors:
Leveraging structure for unsupervised learning from
monocular videos.
Choi, S., Min, D., Ham, B., Kim, Y., Oh, C., and
Sohn, K. (2015). Depth analogy: Data-driven ap-
proach for single image depth estimation using gradi-
ent samples. IEEE Transactions on Image Processing,
24(12):5953–5966.
Ciubotariu, G., Tomescu, V.-I., and Czibula, G. (2021).
Enhancing the performance of image classification
through features automatically learned from depth-
maps. In International Conference on Computer Vi-
sion Systems, pages 68–81. Springer.
Dai, Q., Li, F., Cossairt, O., and Katsaggelos, A. K. (2021).
Adaptive illumination based depth sensing using deep
learning. arXiv preprint arXiv:2103.12297.
Eigen, D., Puhrsch, C., and Fergus, R. (2014). Depth map
prediction from a single image using a multi-scale
deep network.
Furukawa, R., Sagawa, R., and Kawasaki, H. (2017). Depth
estimation using structured light flow — analysis of
projected pattern flow on an object’s surface. In 2017
IEEE International Conference on Computer Vision
(ICCV), pages 4650–4658.
Garg, R., BG, V. K., Carneiro, G., and Reid, I. (2016). Un-
supervised cnn for single view depth estimation: Ge-
ometry to the rescue.
Geiger, A., Lenz, P., and Urtasun, R. (2012). Are we ready
for autonomous driving? the kitti vision benchmark
suite. In 2012 IEEE Conference on Computer Vision
and Pattern Recognition, pages 3354–3361.
Godard, C., Aodha, O. M., and Brostow, G. J. (2017). Un-
supervised monocular depth estimation with left-right
consistency.
Hoiem, D., Efros, A. A., and Hebert, M. (2007). Recovering
surface layout from an image. International Journal
of Computer Vision, 75(1):151–172.
Hoyer, L., Dai, D., Chen, Y., Koring, A., Saha, S., and
Van Gool, L. (2021). Three ways to improve semantic
segmentation with self-supervised depth estimation.
In Proceedings of the IEEE/CVF Conference on Com-
puter Vision and Pattern Recognition, pages 11130–
11140.
Konrad, J., Wang, M., Ishwar, P., Wu, C., and Mukherjee,
D. (2013). Learning-based, automatic 2d-to-3d image
and video conversion. IEEE Transactions on Image
Processing, 22(9):3485–3496.
Ladicky, L., Shi, J., and Pollefeys, M. (2014). Pulling things
out of perspective. pages 89–96.
Lee, J. H., Han, M., Ko, D. W., and Suh, I. (2019). From big
to small: Multi-scale local planar guidance for monoc-
ular depth estimation. ArXiv, abs/1907.10326. Ac-
cessed: 2021-07-20.
Mahjourian, R., Wicke, M., and Angelova, A. (2018). Un-
supervised learning of depth and ego-motion from
monocular video using 3d geometric constraints.
Pillai, S., Ambrus, R., and Gaidon, A. (2018). Superdepth:
Self-supervised, super-resolved monocular depth esti-
mation.
Ranftl, R., Bochkovskiy, A., and Koltun, V. (2021). Vision
transformers for dense prediction. ArXiv preprint.
Saxena, A., Chung, S. H., and Ng, A. Y. (2005). Learning
depth from single monocular images. NIPS 18.
Song, M., Lim, S., and Kim, W. (2021). Monocular depth
estimation using laplacian pyramid-based depth resid-
uals. IEEE Transactions on Circuits and Systems for
Video Technology, pages 1–1.
Yang, Z., Wang, P., Wang, Y., Xu, W., and Nevatia, R.
(2018). Lego: Learning edge with geometry all at
once by watching videos.
Yang, Z., Wang, P., Xu, W., Zhao, L., and Nevatia, R.
(2017). Unsupervised learning of geometry with edge-
aware depth-normal consistency.
Yin, Z. and Shi, J. (2018). Geonet: Unsupervised learning
of dense depth, optical flow and camera pose.
Zhou, T., Brown, M., Snavely, N., and Lowe, D. G. (2017).
Unsupervised learning of depth and ego-motion from
video.
ICAART 2022 - 14th International Conference on Agents and Artificial Intelligence
680