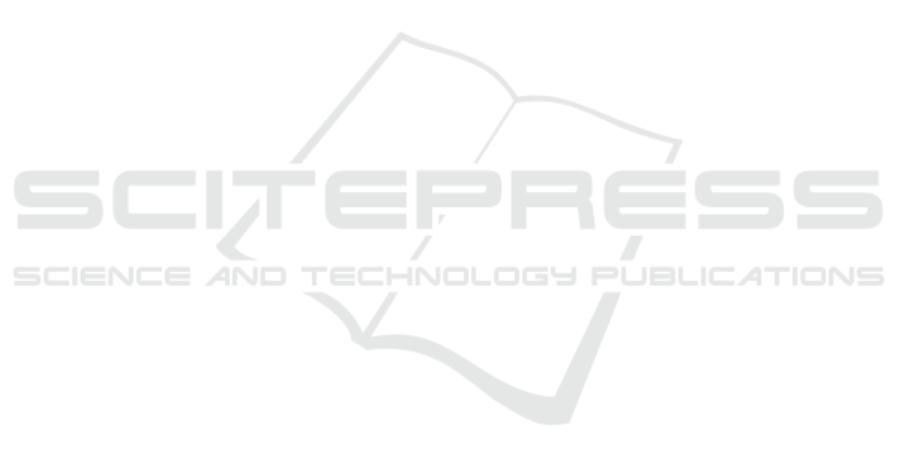
Lin, K., Zhao, R., Xu, Z., and Zhou, J. (2018). Efficient
large-scale fleet management via multi-agent deep re-
inforcement learning. In Proceedings of the 24th
ACM SIGKDD International Conference on Knowl-
edge Discovery & Data Mining, pages 1774–1783.
Liu, L., Qiu, Z., Li, G., Wang, Q., Ouyang, W., and Lin,
L. (2019). Contextualized spatial–temporal network
for taxi origin-destination demand prediction. IEEE
Transactions on Intelligent Transportation Systems,
20(10):3875–3887.
Lu, A., Frazier, P., and Kislev, O. (2018). Surge pric-
ing moves uber’s driver partners. Available at SSRN
3180246.
Ma, S., Zheng, Y., and Wolfson, O. (2014). Real-time city-
scale taxi ridesharing. IEEE Transactions on Knowl-
edge and Data Engineering, 27(7):1782–1795.
Puterman, M. L. (2014). Markov decision processes: dis-
crete stochastic dynamic programming. John Wiley &
Sons.
Qu, M., Zhu, H., Liu, J., Liu, G., and Xiong, H. (2014). A
cost-effective recommender system for taxi drivers. In
Proceedings of the 20th ACM SIGKDD international
conference on Knowledge discovery and data mining,
pages 45–54.
Stasha, S. (2021). Ride-Sharing Industry Statistics to get
you going in 2021. https://policyadvice.net/insurance/
insights/ride-sharing-industry-statistics/.
Wang, Z., Qin, Z., Tang, X., Ye, J., and Zhu, H. (2018).
Deep reinforcement learning with knowledge transfer
for online rides order dispatching. In 2018 IEEE Inter-
national Conference on Data Mining (ICDM), pages
617–626. IEEE.
Watkins, C. J. and Dayan, P. (1992). Q-learning. Machine
learning, 8(3-4):279–292.
Wen, J., Zhao, J., and Jaillet, P. (2017). Rebalancing shared
mobility-on-demand systems: A reinforcement learn-
ing approach. In 2017 IEEE 20th International Con-
ference on Intelligent Transportation Systems (ITSC),
pages 220–225. IEEE.
Wooldridge, M. (2009). An introduction to multiagent sys-
tems. John Wiley & Sons.
Xu, J., Rahmatizadeh, R., B
¨
ol
¨
oni, L., and Turgut, D. (2018).
Real-time prediction of taxi demand using recurrent
neural networks. IEEE Transactions on Intelligent
Transportation Systems, 19(8):2572–2581.
Xu, Z., Li, Z., Guan, Q., Zhang, D., Li, Q., Nan, J., Liu,
C., Bian, W., and Ye, J. (2018). Large-scale order dis-
patch in on-demand ride-hailing platforms: A learning
and planning approach. In Proceedings of the 24th
ACM SIGKDD International Conference on Knowl-
edge Discovery & Data Mining, pages 905–913.
Yao, H., Wu, F., Ke, J., Tang, X., Jia, Y., Lu, S., Gong, P.,
Ye, J., and Li, Z. (2018). Deep multi-view spatial-
temporal network for taxi demand prediction. In Pro-
ceedings of the AAAI Conference on Artificial Intelli-
gence, volume 32.
Zhang, J., Zheng, Y., and Qi, D. (2017a). Deep spatio-
temporal residual networks for citywide crowd flows
prediction. In Proceedings of the AAAI Conference on
Artificial Intelligence, volume 31.
Zhang, L., Hu, T., Min, Y., Wu, G., Zhang, J., Feng,
P., Gong, P., and Ye, J. (2017b). A taxi order dis-
patch model based on combinatorial optimization. In
Proceedings of the 23rd ACM SIGKDD international
conference on knowledge discovery and data mining,
pages 2151–2159.
Zheng, L., Cheng, P., and Chen, L. (2019). Auction-based
order dispatch and pricing in ridesharing. In 2019
IEEE 35th International Conference on Data Engi-
neering (ICDE), pages 1034–1045. IEEE.
T-Balance: A Unified Mechanism for Taxi Scheduling in a City-scale Ride-sharing Service
465