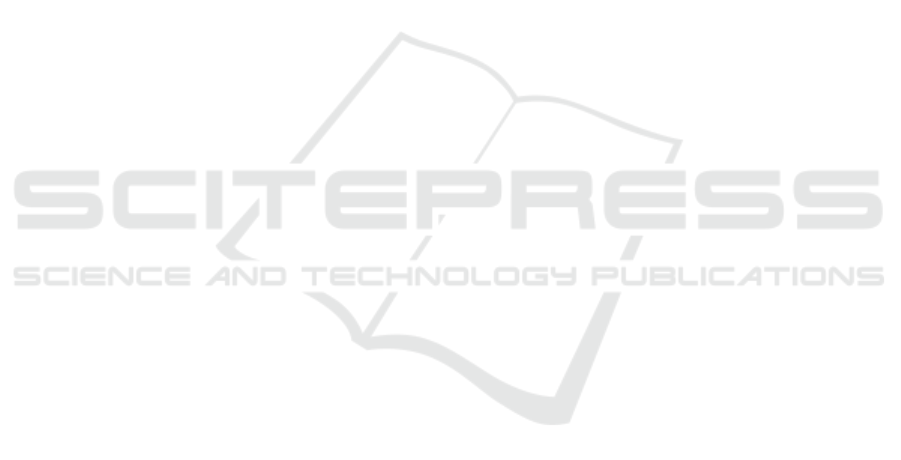
11 FUTURE WORK
After more than one year of intense work in order to
have the LSU-DS dataset, we can begin to use it in
different fields. The dataset will be useful for psy-
cholinguistic studies and also for the training and test-
ing of automatic segmentation, detection and recogni-
tion algorithms.
This first experience was carried in the controlled
conditions of the lab as other available datasets re-
ported in the literature. In the future we plan to en-
rich the dataset with acquisitions outside the lab with
varying scene conditions. This is a necessary trend
that only recently has begun (a notable example is the
AUTSL dataset (Sincan and Keles, 2020) in 2020).
The dataset will be enriched with new metadata
and temporal markers to identify different linguistic
units. In the future, these new data will include infor-
mation such as oral language translation in English
by sign and sentence, phonological description and
grammar type of each sign as well as the syntactic
function of the signs into sentences. A main challenge
is to include labels referring to non-manual sign pa-
rameters such as body tilt, head motion/position, and
facial expressions of the subjects done when signing.
ACKNOWLEDGEMENTS
The authors gratefully acknowledge the contribution
of Mar
´
ıa E. Rodino with the gloss, Federico Lecum-
berry and Gabriel G
´
omez for their help in the web site
installation and to the reviewers’ comments, which
improved the article.
REFERENCES
Cao, Z., Hidalgo Martinez, G., Simon, T., Wei, S., and
Sheikh, Y. A. (2019). Openpose: Realtime multi-
person 2d pose estimation using part affinity fields.
IEEE Transactions on Pattern Analysis and Machine
Intelligence.
Cheok, M. J., Omar, Z., and Jaward, M. H. (2019). A re-
view of hand gesture and sign language recognition
techniques. International Journal of Machine Learn-
ing and Cybernetics, 10(1):131–153.
Cooper, H., Holt, B., and Bowden, R. (2011). Sign language
recognition. In Visual analysis of humans, pages 539–
562. Springer.
De Coster, M., Van Herreweghe, M., and Dambre, J.
(2021). Isolated sign recognition from rgb video us-
ing pose flow and self-attention. In Proceedings of the
IEEE/CVF CVPR Workshops, pages 3441–3450.
Dreuw, P., Deselaers, T., Keysers, D., and Ney, H. (2006).
Modeling image variability in appearance-based ges-
ture recognition. In ECCV Workshop on Statistical
Methods in Multi-Image and Video Processing, pages
7–18.
Koller, O., Ney, H., and Bowden, R. (2016). Deep hand:
How to train a cnn on 1 million hand images when
your data is continuous and weakly labelled. In Pro-
ceedings of the IEEE conference on computer vision
and pattern recognition, pages 3793–3802.
Kozlov, A., Andronov, V., and Gritsenko, Y. (2019).
Lightweight network architecture for real-time action
recognition.
Kumar, P. P., Vadakkepat, P., and Loh, A. P. (2010). Hand
posture and face recognition using a fuzzy-rough ap-
proach. International Journal of Humanoid Robotics,
7(03):331–356.
Lugaresi, C., Tang, J., Nash, H., McClanahan, C., Uboweja,
E., Hays, M., Zhang, F., Chang, C., Yong, M. G., Lee,
J., Chang, W., Hua, W., Georg, M., and Grundmann,
M. (2019). Mediapipe: A framework for building per-
ception pipelines. CoRR, abs/1906.08172.
Peirce, J. W. (2007). Psychopy-psychophysics software in
python. Journal of neuroscience methods, 162(1-2):8–
13.
Pisharady, P. K., Vadakkepat, P., and Loh, A. P. (2013). At-
tention based detection and recognition of hand pos-
tures against complex backgrounds. International
Journal of Computer Vision, 101(3):403–419.
Pugeault, N. and Bowden, R. (2011). Spelling it out: Real-
time asl fingerspelling recognition. In Computer Vi-
sion (ICCV Workshops), IEEE International Confer-
ence on, pages 1114–1119. IEEE.
Ronchetti, F., Quiroga, F., Estrebou, C. A., and Lanzarini,
L. C. (2016a). Handshape recognition for argentinian
sign language using probsom. Journal of Computer
Science & Technology, 16.
Ronchetti, F., Quiroga, F., Estrebou, C. A., Lanzarini, L. C.,
and Rosete, A. (2016b). Lsa64: an argentinian sign
language dataset. In XXII Congreso Argentino de
Ciencias de la Computaci
´
on.
Sincan, O. M. and Keles, H. Y. (2020). AUTSL: A large
scale multi-modal turkish sign language dataset and
baseline methods. CoRR, abs/2008.00932.
Stassi, A. E., Delbracio, M., and Randall, G. (2020).
TReLSU-HS: a new handshape dataset for uruguayan
sign language recognition. In 1st International Virtual
Conference in Sign Language Processing.
Trettenbrein, P. C., Pendzich, N.-K., Cramer, J.-M., Stein-
bach, M., and Zaccarella, E. (2021). Psycholinguistic
norms for more than 300 lexical signs in german sign
language. Behavior Research Methods.
Von Agris, U., Knorr, M., and Kraiss, K.-F. (2008a). The
significance of facial features for automatic sign lan-
guage recognition. In 2008 8th IEEE International
Conference on Automatic Face & Gesture Recogni-
tion, pages 1–6. IEEE.
Von Agris, U. and Kraiss, K.-F. (2007). Towards a video
corpus for signer-independent continuous sign lan-
guage recognition. Gesture in Human-Computer In-
teraction and Simulation, Lisbon, Portugal, May.
ICPRAM 2022 - 11th International Conference on Pattern Recognition Applications and Methods
704