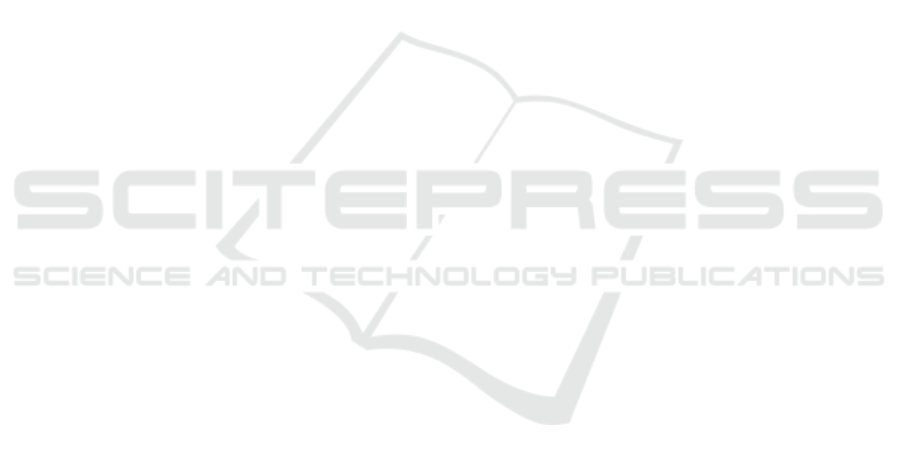
and closed-door decision-making. QoG Working Pa-
per Series, 8:1–32.
de Fine Licht, K. and de Fine Licht, J. (2020). Artificial in-
telligence, transparency, and public decision-making.
AI & SOCIETY, 35(4):917–926.
Diakopoulos, N. and Koliska, M. (2017). Algorithmic
Transparency in the News Media. Digital Journalism,
5(7):809–828.
Eslami, M., Vaccaro, K., Lee, M. K., Elazari Bar On, A.,
Gilbert, E., and Karahalios, K. (2019). User Atti-
tudes towards Algorithmic Opacity and Transparency
in Online Reviewing Platforms. In Proceedings of the
2019 CHI Conference on Human Factors in Comput-
ing Systems, pages 1–14. Association for Computing
Machinery, New York, NY, USA.
Garcia-Ceja, E., Osmani, V., and Mayora, O. (2016). Au-
tomatic Stress Detection in Working Environments
From Smartphones’ Accelerometer Data: A First
Step. IEEE Journal of Biomedical and Health Infor-
matics, 20(4):1053–1060.
Gebru, T., Morgenstern, J., Vecchione, B., Vaughan, J. W.,
Wallach, H., III, H. D., and Crawford, K. (2021).
Datasheets for datasets. Communications of the ACM,
64(12):86–92.
Griffin, R. J., Yang, Z., Ter Huurne, E., Boerner, F., Ortiz,
S., and Dunwoody, S. (2008). After the flood: Anger,
attribution, and the seeking of information. Science
Communication, 29(3):285–315.
Janic, M., Wijbenga, J. P., and Veugen, T. (2013). Trans-
parency Enhancing Tools (TETs): An Overview. In
2013 Third Workshop on Socio-Technical Aspects in
Security and Trust, pages 18–25.
Kang, R., Brown, S., Dabbish, L., and Kiesler, S. (2014).
Privacy Attitudes of Mechanical Turk Workers and the
U.S. Public. In 10th Symposium On Usable Privacy
and Security ({SOUPS} 2014), pages 37–49.
Kaushik, S., Yao, Y., Dewitte, P., and Wang, Y. (2021).
”How I Know For Sure”: People’s Perspectives on
Solely Automated Decision-Making ({SADM}). In
Seventeenth Symposium on Usable Privacy and Secu-
rity ({SOUPS} 2021), pages 159–180.
Kay, M., Elkin, L. A., Higgins, J. J., and Wobbrock, J. O.
(2021). ARTool: Aligned Rank Transform for Non-
parametric Factorial ANOVAs.
Kizilcec, R. F. (2016). How Much Information? effects of
Transparency on Trust in an Algorithmic Interface. In
Proceedings of the 2016 CHI Conference on Human
Factors in Computing Systems, CHI ’16, pages 2390–
2395, New York, NY, USA. Association for Comput-
ing Machinery.
Kunkel, J., Donkers, T., Michael, L., Barbu, C.-M., and
Ziegler, J. (2019). Let Me Explain: Impact of Per-
sonal and Impersonal Explanations on Trust in Rec-
ommender Systems. In Proceedings of the 2019 CHI
Conference on Human Factors in Computing Systems,
pages 1–12. Association for Computing Machinery,
New York, NY, USA.
Mittelstadt, B., Russell, C., and Wachter, S. (2019). Ex-
plaining Explanations in AI. In Proceedings of the
Conference on Fairness, Accountability, and Trans-
parency, FAT* ’19, pages 279–288, New York, NY,
USA. Association for Computing Machinery.
Murmann, P. and Fischer-H
¨
ubner, S. (2017). Tools for
Achieving Usable Ex Post Transparency: A Survey.
IEEE access : practical innovations, open solutions,
5:22965–22991.
Nourani, M., Kabir, S., Mohseni, S., and Ragan, E. D.
(2019). The Effects of Meaningful and Meaningless
Explanations on Trust and Perceived System Accu-
racy in Intelligent Systems. Proceedings of the AAAI
Conference on Human Computation and Crowdsourc-
ing, 7(1):97–105.
Reidenberg, J. R., Breaux, T., Cranor, L. F., French, B.,
Grannis, A., Graves, J. T., Liu, F., McDonald, A., Nor-
ton, T. B., and Ramanath, R. (2015). Disagreeable
privacy policies: Mismatches between meaning and
users’ understanding. Berkeley Tech. LJ, 30:39.
Schaub, F., Balebako, R., Durity, A. L., and Cranor, L. F.
(2015). A design space for effective privacy notices.
In Eleventh Symposium On Usable Privacy and Secu-
rity (SOUPS 2015), pages 1–17.
Tesfay, W. B., Hofmann, P., Nakamura, T., Kiyomoto, S.,
and Serna, J. (2018). PrivacyGuide: Towards an Im-
plementation of the EU GDPR on Internet Privacy
Policy Evaluation. In Proceedings of the Fourth ACM
International Workshop on Security and Privacy Ana-
lytics, IWSPA ’18, pages 15–21, New York, NY, USA.
ACM.
Tubaro, P., Casilli, A. A., and Coville, M. (2020). The
trainer, the verifier, the imitator: Three ways in which
human platform workers support artificial intelli-
gence. Big Data & Society, 7(1):2053951720919776.
Wang, N., Pynadath, D. V., and Hill, S. G. (2016). Trust
Calibration Within a Human-Robot Team: Compar-
ing Automatically Generated Explanations. In The
Eleventh ACM/IEEE International Conference on Hu-
man Robot Interaction, HRI ’16, pages 109–116, Pis-
cataway, NJ, USA. IEEE Press.
Wilson, S., Schaub, F., Dara, A. A., Liu, F., Cherivirala, S.,
Giovanni Leon, P., Schaarup Andersen, M., Zimmeck,
S., Sathyendra, K. M., Russell, N. C., B. Norton, T.,
Hovy, E., Reidenberg, J., and Sadeh, N. (2016). The
Creation and Analysis of a Website Privacy Policy
Corpus. Proceedings of the 54th Annual Meeting of
the Association for Computational Linguistics (Vol-
ume 1: Long Papers), pages 1330–1340. Association
for Computational Linguistics.
Wobbrock, J. O., Findlater, L., Gergle, D., and Higgins,
J. J. (2011). The aligned rank transform for nonpara-
metric factorial analyses using only anova procedures.
In Proceedings of the SIGCHI Conference on Human
Factors in Computing Systems, CHI ’11, pages 143–
146, New York, NY, USA. Association for Computing
Machinery.
Zaeem, R. N., German, R. L., and Barber, K. S. (2018).
PrivacyCheck: Automatic Summarization of Privacy
Policies Using Data Mining. ACM Trans. Internet
Technol., 18(4):53:1–53:18.
ICISSP 2022 - 8th International Conference on Information Systems Security and Privacy
550