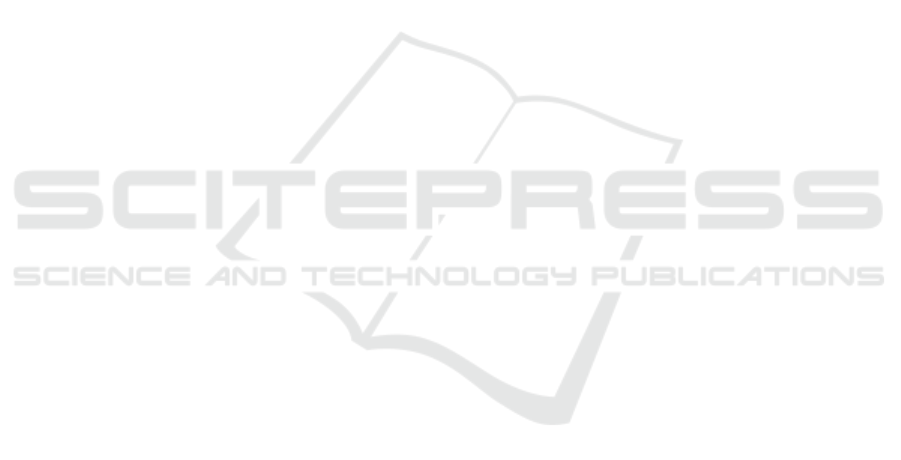
or trying to apprehend an issue in the physical sys-
tem. As a result, we propose to complete trendy data-
driven approaches for DT with a flexible and integra-
tive modelling approach.
Through this approach we defined MDDT proto-
types; they are composed by executable models (de-
fined in a static and dynamic ways) that are captured
from a variety of inputs (e.g., different domain exper-
tise, data consolidation, machine learning, etc). Those
executable models allow notably to provide an easy
to use simulation environment. In addition, MDDT
prototypes should be able to evolve, being reinforced,
some part being replace with data-driven ones (e.g.,
machine learning).
Accordingly, there are still a lot of challenges and
future works to be tackled and implemented to fully
support our vision. Let cite amongst other: ensur-
ing the continuity during MDDT evolution, integra-
tion MDDT with a more data-driven approach (e.g.,
FiWare), multi-language and view for stakeholders in-
volvement, and integration with other DT.
REFERENCES
Barricelli, B. R., Casiraghi, E., and Fogli, D. (2019). A
survey on digital twin: definitions, characteristics, ap-
plications, and design implications. IEEE access,
7:167653–167671.
B
´
ezivin, J. (2004). In search of a basic principle for model
driven engineering. Novatica Journal, Special Issue,
5(2):21–24.
Bjekovic, M., Proper, E., and Sottel, J.-S. (2012). Towards a
coherent enterprise modelling landscape. In 5th Conf.
on the Practice of Enterprise Modeling, Rostock, Ger-
many, 2012, pages 1–12. [Sl]: CEUR.
Bordeleau, F., Combemale, B., Eramo, R., van den Brand,
M., and Wimmer, M. (2020). Towards model-driven
digital twin engineering: Current opportunities and
future challenges. In Conf. on Systems Modelling and
Management, pages 43–54.
Bucchiarone, A., Cabot, J., Paige, R. F., and Pierantonio, A.
(2020). Grand challenges in model-driven engineer-
ing: an analysis of the state of the research. Software
and Systems Modeling, 19(1):5–13.
Chakraborty, S. and Adhikari, S. (2021). Machine learn-
ing based digital twin for dynamical systems with
multiple time-scales. Computers and Structures,
243:106410.
Conde, J., Munoz-Arcentales, A., Alonso, A., Lopez-
Pernas, S., and Salvachua, J. (2021). Modeling dig-
ital twin data and architecture: A building guide with
fiware as enabling technology. Internet Computing.
Famelis, M., Salay, R., and Chechik, M. (2012). Partial
models: Towards modeling and reasoning with uncer-
tainty. In 2012 34th International Conference on Soft-
ware Engineering (ICSE), pages 573–583. IEEE.
Grace, P., Pickering, B., and Surridge, M. (2016). Model-
driven interoperability: engineering heterogeneous iot
systems. Annals of telecommunications.
Guermazi, S., Tatibouet, J., Cuccuru, A., Dhouib, S.,
G
´
erard, S., and Seidewitz, E. (2015). Executable mod-
eling with fuml and alf in papyrus: Tooling and exper-
iments. strategies, 11:12.
Kaur, M. J., Mishra, V. P., and Maheshwari, P. (2020). The
convergence of digital twin, iot, and machine learn-
ing: transforming data into action. In Digital twin
technologies and smart cities, pages 3–17. Springer.
Ketzler, B., Naserentin, V., Latino, F., Zangelidis, C., Thu-
vander, L., and Logg, A. (2020). Digital twins for
cities: A state of the art review. Built Environment,
46(4):547–573.
Ladj, A., Wang, Z., Meski, O., Belkadi, F., Ritou, M.,
and Da Cunha, C. (2021). A knowledge-based dig-
ital shadow for machining industry in a digital twin
perspective. Manufacturing Systems, 58:168–179.
Lektauers, A., Pecerska, J., Bolsakovs, V., Romanovs, A.,
Grabis, J., and TEILANS, A. (2021). A multi-model
approach for simulation-based digital twin in resilient
services. WSEAS Trans. Syst. Control, 16:133–145.
Mayerhofer, T., Langer, P., Wimmer, M., and Kappel, G.
(2013). xmof: Executable dsmls based on fuml. In
International conference on software language engi-
neering, pages 56–75. Springer.
Moawad, A., Hartmann, T., Fouquet, F., Nain, G., Klein,
J., and Le Traon, Y. (2015). Beyond discrete mod-
eling: A continuous and efficient model for iot. In
Conf. Model Driven Engineering Languages and Sys-
tems (MODELS), pages 90–99.
Patni, H., Henson, C., and Sheth, A. (2010). Linked sensor
data. In 2010 International Symposium on Collabora-
tive Technologies and Systems, pages 362–370. IEEE.
Peltonen, J. and Selonen, P. (2001). Processing uml models
with visual scripts. In Proceedings IEEE Symposia on
Human-Centric Computing Languages and Environ-
ments (Cat. No. 01TH8587), pages 264–271. IEEE.
Raj, P. and Surianarayanan, C. (2020). Digital twin: the
industry use cases. In Advances in Computers, volume
117, pages 285–320. Elsevier.
Salay, R. and Chechik, M. (2013). Supporting agility in mde
through modeling language relaxation. In XM 2013–
Extreme Modeling Workshop, page 21. Citeseer.
Singh, S., Shehab, E., Higgins, N., Fowler, K., Reynolds,
D., Erkoyuncu, J. A., and Gadd, P. (2020). Data man-
agement for developing digital twin ontology model.
Proc. of the Institution of Mechanical Engineers.
Sottet, J.-S. and Biri, N. (2016). Jsmf: a javascript flex-
ible modelling framework. FlexMDE@ MoDELS,
1694:42–51.
Zarwin, Z., Bjekovic, M., Favre, M., Sottet, J.-S., and
Proper, H. (2014). Natural modelling. Journal of Ob-
ject Technology, 13:4–1.
Zolotas, A., Matragkas, N., Devlin, S., Kolovos, D. S.,
and Paige, R. F. (2019). Type inference in flexi-
ble model-driven engineering using classification al-
gorithms. Software & Systems Modeling, 18(1):345–
366.
Towards a Lightweight Model-driven Smart-city Digital Twin
327