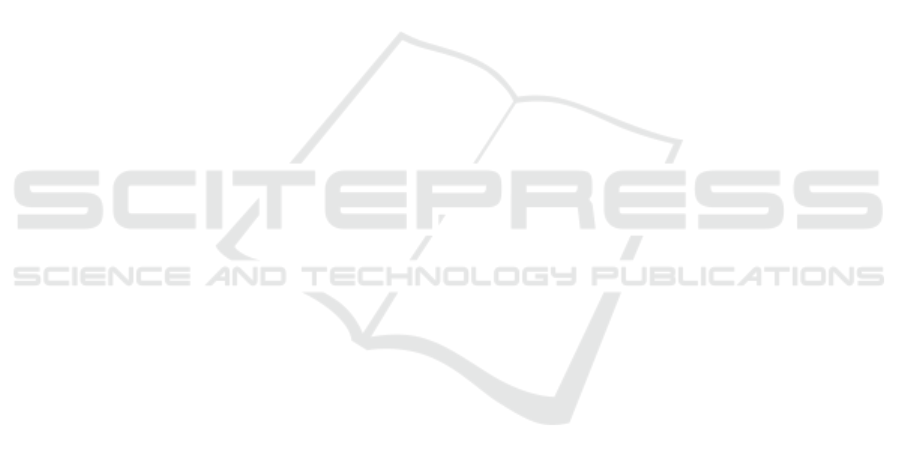
Fayyaz, R. A., Maqbool, M., and Hanif, M. (2020). Textile
design generation using gans. In 2020 IEEE Canadian
Conference on Electrical and Computer Engineering
(CCECE), pages 1–5.
Fuhr, J.-P. (2017). Schichtbasierte Modellierung von Ferti-
gungseffekten in der Struktursimulation von Faserver-
bundwerkstoffen. Verlag Dr. Hut.
Gatys, L., Ecker, A. S., and Bethge, M. (2015). Texture syn-
thesis using convolutional neural networks. Advances
in Neural Information Processing Systems, 28.
Goodfellow, I. J., Pouget-Abadie, J., Mirza, M., Xu, B.,
Warde-Farley, D., Ozair, S., Courville, A., and Ben-
gio, Y. (2014). Generative adversarial networks.
Heusel, M., Ramsauer, H., Unterthiner, T., Nessler, B., and
Hochreiter, S. (2017). GANs Trained by a Two Time-
Scale Update Rule Converge to a Local Nash Equi-
librium. In I. Guyon, U. V. Luxburg, S. Bengio, H.
Wallach, R. Fergus, S. Vishwanathan, and R. Garnett,
editors, Advances in Neural Information Processing
Systems, volume 30, pages 6626–6637. Curran Asso-
ciates, Inc.
Jetchev, N., Bergmann, U., and Vollgraf, R. (2017). Texture
synthesis with spatial generative adversarial networks.
Jing, J.-F., Ma, H., and Zhang, H.-H. (2019). Automatic
fabric defect detection using a deep convolutional neu-
ral network. Coloration Technology, 135(3):213–223.
K. He, X. Zhang, S. Ren, and J. Sun (2016). Deep Residual
Learning for Image Recognition. In 2016 IEEE Con-
ference on Computer Vision and Pattern Recognition
(CVPR), pages 770–778.
Liu, G., Gousseau, Y., and Xia, G.-S. (2016). Texture
synthesis through convolutional neural networks and
spectrum constraints. In 2016 23rd International Con-
ference on Pattern Recognition (ICPR), pages 3234–
3239.
McInnes, L., Healy, J., and Melville, J. (2020). Umap: Uni-
form manifold approximation and projection for di-
mension reduction.
Miao, M. and Xin, J. H. (2018). Engineering of high-
performance textiles. The Textile Institute Book Se-
ries. Woodhead Publishing.
Ronneberger, O., Fischer, P., and Brox, T. (2015). U-Net:
Convolutional Networks for Biomedical Image Seg-
mentation. In Navab, N., Hornegger, J., Wells, W. M.,
and Frangi, A. F., editors, Medical image computing
and computer-assisted intervention - MICCAI 2015,
Lecture notes in computer science, pages 234–241,
Cham and Heidelberg and New York and Dordrecht
and London. Springer.
S. Schreiber, J. Geldenhuys, and H. de Villiers (2016).
Texture synthesis using convolutional neural networks
with long-range consistency and spectral constraints.
In 2016 Pattern Recognition Association of South
Africa and Robotics and Mechatronics International
Conference (PRASA-RobMech), pages 1–6.
Sacco, C., Radwan, A. B., Beatty, T., and Harik, R. (2019).
Machine learning based afp inspection: A tool for
characterization and integration. In SAMPE 2019
Conference & Exhibition. Charlotte, NC: SAMPE.
doi, volume 10, pages 19–1594.
Sacco, C., Radwan, A. B., Harik, R., and Van Tooren, M.
(2018). Automated fiber placement defects: Auto-
mated inspection and characterization. In Proceedings
of the SAMPE 2018 Conference and Exhibition, Long
Beach, CA, USA, pages 21–24.
Salimans, T., Goodfellow, I., Zaremba, W., Cheung, V.,
Radford, A., and Chen, X. (2016). Improved Tech-
niques for Training GANs. In D. Lee, M. Sugiyama,
U. Luxburg, I. Guyon, and R. Garnett, editors, Ad-
vances in Neural Information Processing Systems,
volume 29, pages 2234–2242. Curran Associates, Inc.
Shorten, C. and Khoshgoftaar, T. M. (2019). A survey on
image data augmentation for deep learning. Journal
of Big Data, 6(1):1–48.
Szegedy, C., Vanhoucke, V., Ioffe, S., Shlens, J., and Wojna,
Z. (2016). Rethinking the Inception Architecture for
Computer Vision. In 2016 IEEE Conference on Com-
puter Vision and Pattern Recognition (CVPR), pages
2818–2826.
T
¨
ure, Y. and T
¨
ure, C. (2020). An assessment of using
aluminum and magnesium on co2 emission in euro-
pean passenger cars. Journal of Cleaner Production,
247:119120.
Wei, B., Hao, K., Tang, X.-s., and Ding, Y. (2019). A
new method using the convolutional neural network
with compressive sensing for fabric defect classifica-
tion based on small sample sizes. Textile Research
Journal, 89(17):3539–3555.
Xian, W., Sangkloy, P., Agrawal, V., Raj, A., Lu, J., Fang,
C., Yu, F., and Hays, J. (2018). Texturegan: Control-
ling deep image synthesis with texture patches. pages
8456–8465.
Yi, X., Walia, E., and Babyn, P. (2019). Generative adver-
sarial network in medical imaging: A review. Medical
Image Analysis, 58:101552.
Zhu, J.-Y., Zhang, R., Pathak, D., Darrell, T., Efros, A. A.,
Wang, O., and Shechtman, E. (2017). Toward multi-
modal image-to-image translation. In I. Guyon, U. V.
Luxburg, S. Bengio, H. Wallach, R. Fergus, S. Vish-
wanathan, and R. Garnett, editors, Advances in Neural
Information Processing Systems 30, pages 465–476.
Curran Associates, Inc.
Zhu, X., Liu, Y., Li, J., Wan, T., and Qin, Z. (2018). Emo-
tion classification with data augmentation using gen-
erative adversarial networks. In Phung, D., Tseng,
V. S., Webb, G. I., Ho, B., Ganji, M., and Rashidi, L.,
editors, Advances in Knowledge Discovery and Data
Mining, Lecture notes in computer science, pages
349–360, Cham. Springer International Publishing.
Controlled Synthesis of Fibre-reinforced Plastics Images from Segmentation Maps using Generative Adversarial Neural Networks
809