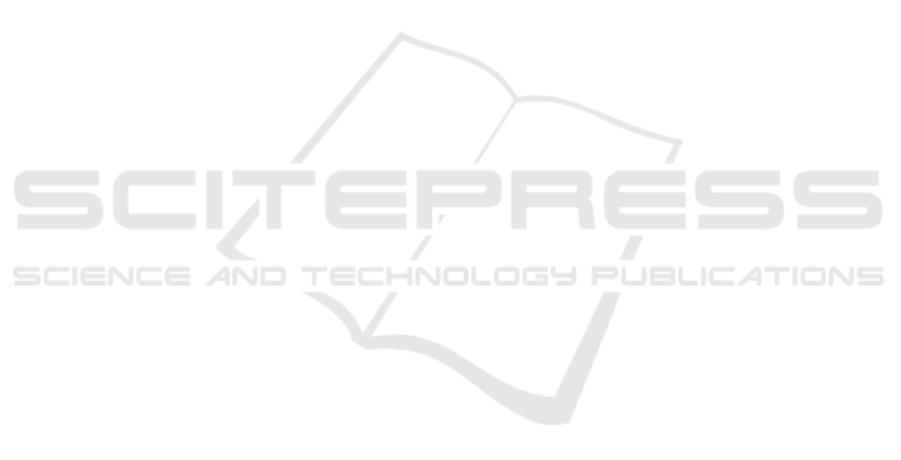
REFERENCES
Ahmad, S., Lavin, A., Purdy, S., and Agha, Z. (2017). Un-
supervised real-time anomaly detection for streaming
data. Neurocomputing, 262:134–147.
Bathelt, A., Ricker, N. L., and Jelali, M. (2015). Revi-
sion of the tennessee eastman process model. IFAC-
PapersOnLine, 48(8):309–314.
Bhatia, S., Jain, A., Li, P., Kumar, R., and Hooi, B. (2021).
Fast anomaly detection in multi-aspect streams. In
The Web Conference (WWW).
Breunig, M. M., Kriegel, H.-P., Ng, R. T., and Sander, J.
(2000). Lof: identifying density-based local outliers.
In Proceedings of the 2000 ACM SIGMOD interna-
tional conference on Management of data, pages 93–
104.
Cover, T. and Hart, P. (1967). Nearest neighbor pattern clas-
sification. IEEE transactions on information theory,
13(1):21–27.
Demetriou, P., Becker, I., and Hailes, S. (2022). High-
performance accessible anomaly detection for critical
systems. under review.
Detroja, K., Gudi, R., and Patwardhan, S. (2006). A
possibilistic clustering approach to novel fault de-
tection and isolation. Journal of Process Control,
16(10):1055–1073.
Downs, J. J. and Vogel, E. F. (1993). A plant-wide indus-
trial process control problem. Computers & chemical
engineering, 17(3):245–255.
Dua, D. and Graff, C. (2017). UCI machine learning repos-
itory.
Lee, J.-M., Qin, S. J., and Lee, I.-B. (2006). Fault detection
and diagnosis based on modified independent compo-
nent analysis. AIChE journal, 52(10):3501–3514.
Liu, F. T., Ting, K. M., and Zhou, Z.-H. (2012). Isolation-
based anomaly detection. ACM Transactions on
Knowledge Discovery from Data (TKDD), 6(1):1–39.
Mitchell, R. and Chen, I.-R. (2014). A survey of intrusion
detection techniques for cyber-physical systems. ACM
Computing Surveys (CSUR), 46(4):1–29.
Pedregosa, F., Varoquaux, G., Gramfort, A., Michel, V.,
Thirion, B., Grisel, O., Blondel, M., Prettenhofer,
P., Weiss, R., Dubourg, V., Vanderplas, J., Passos,
A., Cournapeau, D., Brucher, M., Perrot, M., and
Duchesnay, E. (2011). Scikit-learn: Machine learning
in Python. Journal of Machine Learning Research,
12:2825–2830.
Rieth, C., Amsel, B., Tran, R., and Cook, M. (2017). Addi-
tional tennessee eastman process simulation data for
anomaly detection evaluation. Harvard Dataverse, 1.
Rousseeuw, P. J. and Driessen, K. V. (1999). A fast algo-
rithm for the minimum covariance determinant esti-
mator. Technometrics, 41(3):212–223.
Sch
¨
olkopf, B., Platt, J. C., Shawe-Taylor, J., Smola, A. J.,
and Williamson, R. C. (2001). Estimating the support
of a high-dimensional distribution. Neural computa-
tion, 13(7):1443–1471.
Sharafaldin, I., Lashkari, A. H., and Ghorbani, A. A.
(2018). Toward generating a new intrusion detection
dataset and intrusion traffic characterization. ICISSp,
1:108–116.
Woods, K. S., Solka, J. L., Priebe, C. E., Kegelmeyer Jr,
W. P., Doss, C. C., and Bowyer, K. W. (1994). Com-
parative evaluation of pattern recognition techniques
for detection of microcalcifications in mammography.
In State of The Art in Digital Mammographic Image
Analysis, pages 213–231. World Scientific.
ICISSP 2022 - 8th International Conference on Information Systems Security and Privacy
586